We will reuse the Gaussian plotter as defined in
(The code is repeated here)
Data
The following code generates the above dataframe.
data = [
(24.122348, 1.827472, 98),
(24.828252, 4.333549, 186),
(26.810812, 1.728494, 276),
(25.997897, 1.882424, 373),
(24.503944, 2.222210, 471),
(27.488572, 1.750039, 604),
(31.556823, 3.844592, 683),
(27.920951, 0.891394, 792),
(27.009054, 1.917744, 897),
]
df = pd.DataFrame(data, columns=["height", "fwhm", "center"])
Gaussian
Taken from the reference post above.
import matplotlib.cm as mpl_cm
import matplotlib.colors as mpl_colors
import matplotlib.pyplot as plt
import numpy as np
from scipy.spatial.distance import cdist
class Gaussian:
def __init__(self, size):
self.size = size
self.center = np.array(self.size) / 2
self.axis = self._calculate_axis()
def _calculate_axis(self):
"""
Generate a list of rows, columns over multiple axis.
Example:
Input: size=(5, 3)
Output: [array([0, 1, 2, 3, 4]), array([[0], [1], [2]])]
"""
axis = [np.arange(size).reshape(-1, *np.ones(idx, dtype=np.uint8))
for idx, size in enumerate(self.size)]
return axis
def update_size(self, size):
""" Update the size and calculate new centers and axis. """
self.size = size
self.center = np.array(self.size) / 2
self.axis = self._calculate_axis()
def create(self, dim=1, fwhm=3, center=None):
""" Generate a gaussian distribution on the center of a certain width. """
center = center if center is not None else self.center[:dim]
distance = sum((ax - ax_center) ** 2 for ax_center, ax in zip(center, self.axis))
distribution = np.exp(-4 * np.log(2) * distance / fwhm ** 2)
return distribution
def creates(self, dim=2, fwhm=3, centers: np.ndarray = None):
""" Combines multiple gaussian distributions based on multiple centers. """
centers = np.array(centers or np.array([self.center]).T).T
indices = np.indices(self.size).reshape(dim, -1).T
distance = np.min(cdist(indices, centers, metric='euclidean'), axis=1)
distance = np.power(distance.reshape(self.size), 2)
distribution = np.exp(-4 * np.log(2) * distance / fwhm ** 2)
return distribution
@staticmethod
def plot(distribution, show=True):
""" Plotter, in case you do not know the dimensions of your distribution, or want the same interface. """
if len(distribution.shape) == 1:
return Gaussian.plot1d(distribution, show)
if len(distribution.shape) == 2:
return Gaussian.plot2d(distribution, show)
if len(distribution.shape) == 3:
return Gaussian.plot3d(distribution, show)
raise ValueError(f"Trying to plot {len(distribution.shape)}-dimensional data, "
f"Only 1D, 2D, and 3D distributions are valid.")
@staticmethod
def plot1d(distribution, show=True, vmin=None, vmax=None, cmap=None):
norm = mpl_colors.Normalize(
vmin=vmin if vmin is not None else distribution.min(),
vmax=vmax if vmin is not None else distribution.max()
)
cmap = mpl_cm.ScalarMappable(norm=norm, cmap=cmap or mpl_cm.get_cmap('jet'))
cmap.set_array(distribution)
c = [cmap.to_rgba(value) for value in distribution] # defines the color
fig, ax = plt.subplots()
ax.scatter(np.arange(len(distribution)), distribution, c=c)
ax.plot(distribution)
fig.colorbar(cmap)
if show: plt.show()
return fig
@staticmethod
def plot2d(distribution, show=True):
fig, ax = plt.subplots()
img = ax.imshow(distribution, cmap='jet')
fig.colorbar(img)
if show: plt.show()
return fig
@staticmethod
def plot3d(distribution, show=True):
m, n, c = distribution.shape
x, y, z = np.mgrid[:m, :n, :c]
out = np.column_stack((x.ravel(), y.ravel(), z.ravel(), distribution.ravel()))
x, y, z, values = np.array(list(zip(*out)))
fig = plt.figure()
ax = fig.add_subplot(111, projection='3d')
# Standalone colorbar, directly creating colorbar on fig results in strange artifacts.
img = ax.scatter([0, 0], [0, 0], [0, 0], c=[0, 1], cmap=mpl_cm.get_cmap('jet'))
img.set_visible = False
fig.colorbar(img)
ax.scatter(x, y, z, c=values, cmap=mpl_cm.get_cmap('jet'))
if show: plt.show()
return fig
Solution
Since it is unclear to me how you want to the Gaussian distributions to interact when they are in part of multiple widths, I will assume that you want the maximum value.
Then the main logic is that we can now generate a unique Gaussian distribution for every center with given full width half maximum (fwhm), and take the maximum of all the distrubutions.
distribution = np.zeros((1200,))
df = pd.DataFrame(data, columns=["height", "fwhm", "center"])
gaussian = Gaussian(size=distribution.shape)
for idx, row in df.iterrows():
distribution = np.maximum(distribution, gaussian.create(fwhm=row.fwhm, center=[row.center]))
gaussian.plot(distribution, show=True)
Result
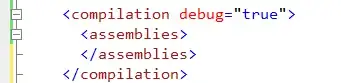
Edit
Since the question now asks for a different distribution, you can adjust the code in the create
(and creates
) method with the following to get different types of distributions:
def create(self, dim=1, fwhm=3, center=None):
""" Generate a gaussian distribution on the center of a certain width. """
center = center if center is not None else self.center[:dim]
distance = sum((ax - ax_center) for ax_center, ax in zip(center, self.axis))
distribution = sps.beta.pdf(distance / max(distance), a=3, b=100)
return distribution
Where sps.beta
comes from import scipy.stats as sps
, and can be changed with a gamma distribution as well. e.g. distribution = sps.gamma.pdf(distance, 10, 40)
.
Note that the distance is no longer squared, and that the argument fwhm
, could be replaced by the parameters required for the distribution.