Secondary axis
Update: It turns out this is much simpler with secondary_xaxis()
instead of twiny()
. You can use the functions
param to specify the transform and inverse functions between the bottom and top axes:
import matplotlib.pyplot as plt
import numpy as np
fig, ax1 = plt.subplots(1, figsize=(10,6))
ax1.set_ylabel('y axis')
ax1.set_xlabel('Linear axis')
ax1.set_ylim(0.1, 1.)
ax1.set_xlim(0.1e-9, 1.5e-9)
# secondary x-axis transformed with x*(a*b) and inverted with x/(a*b)
a, b = 4.*np.pi, np.float64((2.*3.086e22)**2.)
axup = ax1.secondary_xaxis('top', functions=(lambda x: x*(a*b), lambda x: x/(a*b)))
axup.set_xscale('log')
axup.set_xlabel('Log axis')
plt.show()
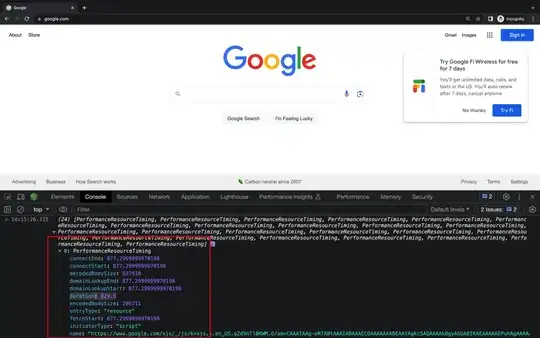
Original example:
# secondary x-axis transformed with x*a/b and inverted with x*b/a
ax1.set_xlim(0.1, 10.)
a, b = 1.e37, 2.*(3.809e8)
axup = ax1.secondary_xaxis('top', functions=(lambda x: x*a/b, lambda x: x*b/a))
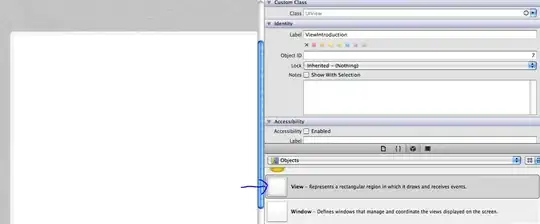
Callback
You can use Axes callbacks to connect ax1
with axup
:
[The Axes
callback] events you can connect to are xlim_changed
and ylim_changed
and the callback will be called with func(ax)
where ax
is the Axes
instance.
Here the ax1.xlim_changed
event triggers scale_axup()
to scale axup.xlim
as scale(ax1.xlim)
. Note that I increased the xlim
up to 10 to demonstrate more major ticks:
from matplotlib.ticker import LogFormatterMathtext
import matplotlib.pyplot as plt
import numpy as np
fig, ax1 = plt.subplots(1, figsize=(15,9))
# axup scaler
scale = lambda x: x*1.e37/(2.*(3.809e8))
# set axup.xlim to scale(ax1.xlim)
def scale_axup(ax1):
# mirror xlim on both axes
left, right = scale(np.array(ax1.get_xlim()))
axup.set_xlim(left, right)
# set xticks to 0.1e28 intervals
xticks = np.arange(float(f'{left:.1e}'), float(f'{right:.1e}'), 0.1e28)
axup.set_xticks([float(f'{tick:.0e}') for tick in xticks])
axup.xaxis.set_major_formatter(LogFormatterMathtext())
# redraw to update xticks
axup.figure.canvas.draw()
# connect ax1 with axup (before ax1.set_xlim())
axup = ax1.twiny()
axup.set_xscale('log')
axup.set_xlabel(r'Log axis')
ax1.callbacks.connect(r'xlim_changed', scale_axup)
ax1.set_ylabel(r'y axis')
ax1.set_xlabel(r'Linear axis')
ax1.set_ylim(0.1, 1.)
ax1.set_xlim(0.1, 10.)
plt.show()