For non-ascending x
splines can be easily computed if you make both x
and y
functions of another parameter t
: x(t)
, y(t)
.
In your case you have 5 points so t
should be just enumeration of these points, i.e. t = 0, 1, 2, 3, 4
for 5 points.
So if x = [5, 2, 7, 3, 6]
then x(t) = x(0) = 5
, x(1) = 2
, x(2) = 7
, x(3) = 3
, x(4) = 6
. Same for y
.
Then compute spline function for both x(t)
and y(t)
. Afterwards compute values of splines in all many intermediate t
points. Lastly just use all calculated values x(t)
and y(t)
as a function y(x)
.
Once before I implemented cubic spline computation from scratch using Numpy, so I use this code in my example below if you don't mind (it could be useful for you to learn about spline math), replace with your library functions. Also in my code you can see numba
lines commented out, if you want you can use these Numba annotations to speed up computation.
You have to look at main()
function at the bottom of code, it shows how to compute and use x(t)
and y(t)
.
Try it online!
import numpy as np, matplotlib.pyplot as plt
# Solves linear system given by Tridiagonal Matrix
# Helper for calculating cubic splines
#@numba.njit(cache = True, fastmath = True, inline = 'always')
def tri_diag_solve(A, B, C, F):
n = B.size
assert A.ndim == B.ndim == C.ndim == F.ndim == 1 and (
A.size == B.size == C.size == F.size == n
) #, (A.shape, B.shape, C.shape, F.shape)
Bs, Fs = np.zeros_like(B), np.zeros_like(F)
Bs[0], Fs[0] = B[0], F[0]
for i in range(1, n):
Bs[i] = B[i] - A[i] / Bs[i - 1] * C[i - 1]
Fs[i] = F[i] - A[i] / Bs[i - 1] * Fs[i - 1]
x = np.zeros_like(B)
x[-1] = Fs[-1] / Bs[-1]
for i in range(n - 2, -1, -1):
x[i] = (Fs[i] - C[i] * x[i + 1]) / Bs[i]
return x
# Calculate cubic spline params
#@numba.njit(cache = True, fastmath = True, inline = 'always')
def calc_spline_params(x, y):
a = y
h = np.diff(x)
c = np.concatenate((np.zeros((1,), dtype = y.dtype),
np.append(tri_diag_solve(h[:-1], (h[:-1] + h[1:]) * 2, h[1:],
((a[2:] - a[1:-1]) / h[1:] - (a[1:-1] - a[:-2]) / h[:-1]) * 3), 0)))
d = np.diff(c) / (3 * h)
b = (a[1:] - a[:-1]) / h + (2 * c[1:] + c[:-1]) / 3 * h
return a[1:], b, c[1:], d
# Spline value calculating function, given params and "x"
#@numba.njit(cache = True, fastmath = True, inline = 'always')
def func_spline(x, ix, x0, a, b, c, d):
dx = x - x0[1:][ix]
return a[ix] + (b[ix] + (c[ix] + d[ix] * dx) * dx) * dx
# Compute piece-wise spline function for "x" out of sorted "x0" points
#@numba.njit([f'f{ii}[:](f{ii}[:], f{ii}[:], f{ii}[:], f{ii}[:], f{ii}[:], f{ii}[:])' for ii in (4, 8)],
# cache = True, fastmath = True, inline = 'always')
def piece_wise_spline(x, x0, a, b, c, d):
xsh = x.shape
x = x.ravel()
ix = np.searchsorted(x0[1 : -1], x)
y = func_spline(x, ix, x0, a, b, c, d)
y = y.reshape(xsh)
return y
def main():
x0 = np.array([4.0, 5.638304088577984, 6.785456961280076, 5.638304088577984, 4.0])
y0 = np.array([0.0, 1.147152872702092, 2.7854569612800755, 4.423761049858059, 3.2766081771559668])
t0 = np.arange(len(x0)).astype(np.float64)
plt.plot(x0, y0)
vs = []
for e in (x0, y0):
a, b, c, d = calc_spline_params(t0, e)
x = np.linspace(0, t0[-1], 100)
vs.append(piece_wise_spline(x, t0, a, b, c, d))
plt.plot(vs[0], vs[1])
plt.show()
if __name__ == '__main__':
main()
Output:
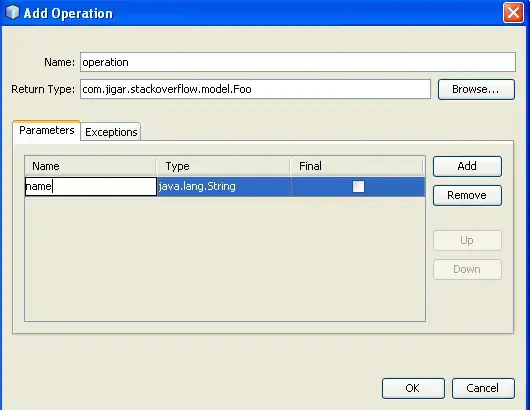