As fmw42 suggested, you need to restrict the problem more. There are way too many variables to build a "works under all circumstances" solution. A possible, very basic, solution would be to try and get the convex hull
of the page.
Another, more robust approach, would be to search for the four vertices of the corners and extrapolate lines to approximate the paper edges. That way you don't need perfect, clean edges, because you would reconstruct them using the four (maybe even three) corners.
To find the vertices you can run Hough Line detector or a Corner Detector on the edges and get at least four discernible clusters of end/starting points. From that you can average the four clusters to get a pair of (x, y)
points per corner and extrapolate lines using those points.
That solution would be hypothetical and pretty laborious for a Stack Overflow question, so let me try the first proposal - detection via convex hull. Here are the steps:
- Threshold the input image
- Get edges from the input
- Get the external contours of the edges using a minimum area filter
- Get the convex hull of the filtered image
- Get the corners of the convex hull
Let's see the code:
# imports:
import cv2
import numpy as np
# image path
path = "D://opencvImages//"
fileName = "img2.jpg"
# Reading an image in default mode:
inputImage = cv2.imread(path + fileName)
# Deep copy for results:
inputImageCopy = inputImage.copy()
# Convert BGR to grayscale:
grayInput = cv2.cvtColor(inputImageCopy, cv2.COLOR_BGR2GRAY)
# Threshold via Otsu:
_, binaryImage = cv2.threshold(grayInput, 0, 255, cv2.THRESH_BINARY + cv2.THRESH_OTSU)
The first step is to get a binary image, very straightforward. This is the result if you threshold via Otsu:
It is never a good idea to try and segment an object from a textured (or high frequency) background, however, in this case the paper it is discernible in the image histogram and the binary image is reasonably good. Let's try and detect edges on this image, I'm applying Canny
with the same parameters as your code:
# Get edges:
cannyImage = cv2.Canny(binaryImage, threshold1=120, threshold2=255, edges=1)
Which produces this:
Seems good enough, the target edges are mostly present. Let's detect contours. The idea is to set an area filter, because the target contour is the biggest amongst the rest. I (heuristically) set a minimum area of 100000
pixels. Once the target contour is found I get its convex hull, like this:
# Find the EXTERNAL contours on the binary image:
contours, hierarchy = cv2.findContours(cannyImage, cv2.RETR_EXTERNAL, cv2.CHAIN_APPROX_SIMPLE)
# Store the corners:
cornerList = []
# Look for the outer bounding boxes (no children):
for i, c in enumerate(contours):
# Approximate the contour to a polygon:
contoursPoly = cv2.approxPolyDP(c, 3, True)
# Convert the polygon to a bounding rectangle:
boundRect = cv2.boundingRect(contoursPoly)
# Get the bounding rect's data:
rectX = boundRect[0]
rectY = boundRect[1]
rectWidth = boundRect[2]
rectHeight = boundRect[3]
# Estimate the bounding rect area:
rectArea = rectWidth * rectHeight
# Set a min area threshold
minArea = 100000
# Filter blobs by area:
if rectArea > minArea:
# Get the convex hull for the target contour:
hull = cv2.convexHull(c)
# (Optional) Draw the hull:
color = (0, 0, 255)
cv2.polylines(inputImageCopy, [hull], True, color, 2)
You'll notice I've prepared beforehand a list (cornerList
) in which I'll store (hopefully) all the corners. The last two lines of the previous snippet are optional, they draw the convex hull via cv2.polylines, this would be the resulting image:
Still inside the loop, after we compute the convex hull, we will get the corners via cv2.goodFeaturesToTrack
, which implements a Corner Detector. The function receives a binary image, so we need to prepare a black image with the convex hull points drawn in white:
# Create image for good features to track:
(height, width) = cannyImage.shape[:2]
# Black image same size as original input:
hullImg = np.zeros((height, width), dtype =np.uint8)
# Draw the points:
cv2.drawContours(hullImg, [hull], 0, 255, 2)
cv2.imshow("hullImg", hullImg)
cv2.waitKey(0)
This is the image:
Now, we must set the corner detector. It needs the number of corners you are looking for, a minimum "quality" parameter that discards poor points detected as "corners" and a minimum distance between the corners. Check out the documentation for more parameters. Let's set the detector, it will return an array of points where it detected a corner. After we get this array, we will store each point in our cornerList
, like this:
# Set the corner detection:
maxCorners = 4
qualityLevel = 0.01
minDistance = int(max(height, width) / maxCorners)
# Get the corners:
corners = cv2.goodFeaturesToTrack(hullImg, maxCorners, qualityLevel, minDistance)
corners = np.int0(corners)
# Loop through the corner array and store/draw the corners:
for c in corners:
# Flat the array of corner points:
(x, y) = c.ravel()
# Store the corner point in the list:
cornerList.append((x,y))
# (Optional) Draw the corner points:
cv2.circle(inputImageCopy, (x, y), 5, 255, 5)
cv2.imshow("Corners", inputImageCopy)
cv2.waitKey(0)
Additionally you can draw the corners as circles, it will yield this image:
This is the same algorithm tested on your third image:
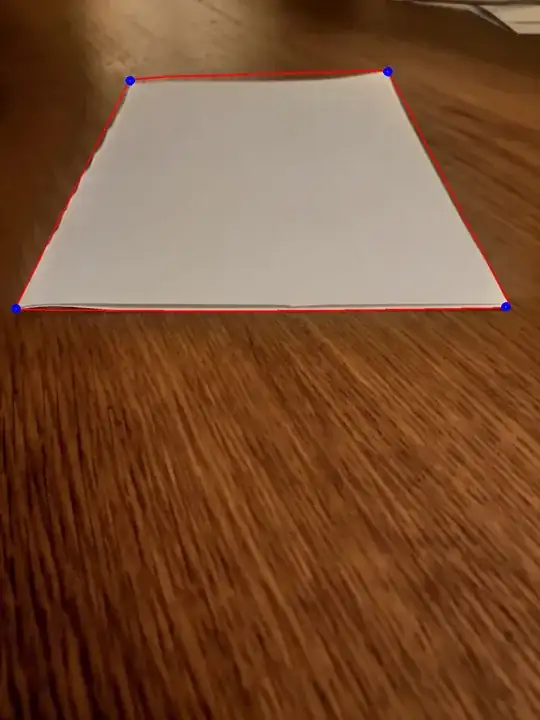