As pointed out by @desertnaut the MLP initialization seems indeed to be the issue as the difference between the MLP and LR coefficients appear to decrease as the sample size increases.
from sklearn.neural_network import MLPClassifier
from sklearn.linear_model import LogisticRegression
from sklearn.preprocessing import StandardScaler
from sklearn.datasets import make_classification
random_state = 100
n_samples = 1000
X, y = make_classification(n_samples=n_samples, n_features=2, n_redundant=0, n_informative=2, n_clusters_per_class=1, random_state=random_state)
X = StandardScaler().fit_transform(X)
nn = MLPClassifier(hidden_layer_sizes=(), solver='lbfgs', activation='logistic', alpha=0, max_iter=1000, tol=0, random_state=random_state).fit(X,y)
lr = LogisticRegression(penalty='none', solver='lbfgs', fit_intercept=True, max_iter=1000, tol=0, random_state=random_state).fit(X,y)
print(nn.intercepts_[0])
print(lr.intercept_)
# [-1.08397244]
# [-1.08397505]
print(nn.coefs_[0].T)
print(lr.coef_)
# [[ 2.90716947 -3.08525711]]
# [[ 2.90718263 -3.08525826]]
The code below shows that as the sample size increases the variance of the MLP coefficients decreases and both the MLP coefficients and the LR coefficients converge to the true coefficients, even though the exact cut-off point depends on the dataset.
import numpy as np
import pandas as pd
from sklearn.neural_network import MLPClassifier
from sklearn.linear_model import LogisticRegression
import plotly.graph_objects as go
from plotly.subplots import make_subplots
# sample sizes
n_samples = [25, 50, 75, 100, 250, 500, 750, 1000, 5000, 10000]
# number of refits of the MLP and LR
# models for each sample size
n_repetitions = 100
# synthetic data
true_intercept = 10
true_weights = [20, 30]
X = np.random.multivariate_normal(np.zeros(2), np.eye(2), np.max(n_samples))
Z = true_intercept + np.dot(X, true_weights) + np.random.normal(0, 1, np.max(n_samples))
p = 1 / (1 + np.exp(- Z))
y = np.random.binomial(1, p, np.max(n_samples))
# data frame for storing the results for each sample size
output = pd.DataFrame(columns=['sample size', 'label avg.', 'LR intercept avg.', 'LR intercept std.', 'NN intercept avg.',
'NN intercept std.', 'LR first weight avg.', 'LR first weight std.', 'NN first weight avg.', 'NN first weight std.',
'LR second weight avg.', 'LR second weight std.', 'NN second weight avg.', 'NN second weight std.'])
# loop across the different
# sample sizes "n"
for n in n_samples:
lr_intercept, lr_coef = [], []
nn_intercept, nn_coef = [], []
# refit the MLP and LR models multiple times
# using the first "n" samples
for k in range(n_repetitions):
nn = MLPClassifier(hidden_layer_sizes=(), solver='lbfgs', activation='logistic', alpha=0, max_iter=1000, tol=0)
lr = LogisticRegression(penalty='none', solver='lbfgs', fit_intercept=True, max_iter=1000, tol=0)
nn.fit(X[:n, :], y[:n])
lr.fit(X[:n, :], y[:n])
lr_intercept.append(lr.intercept_)
nn_intercept.append(nn.intercepts_[0])
lr_coef.append(lr.coef_)
nn_coef.append(nn.coefs_[0].T)
# save the sample mean and sample standard deviations
# of the MLP and LR estimated coefficients for the
# considered sample size "n"
output = output.append(pd.DataFrame({
'sample size': [n],
'label avg.': [np.mean(y[:n])],
'LR intercept avg.': [np.mean(lr_intercept)],
'LR intercept std.': [np.std(lr_intercept, ddof=1)],
'NN intercept avg.': [np.mean(nn_intercept)],
'NN intercept std.': [np.std(nn_intercept, ddof=1)],
'LR first weight avg.': [np.mean(lr_coef, axis=0)[0][0]],
'LR first weight std.': [np.std(lr_coef, ddof=1, axis=0)[0][0]],
'NN first weight avg.': [np.mean(nn_coef, axis=0)[0][0]],
'NN first weight std.': [np.std(nn_coef, ddof=1, axis=0)[0][0]],
'LR second weight avg.': [np.mean(lr_coef, axis=0)[0][1]],
'LR second weight std.': [np.std(lr_coef, ddof=1, axis=0)[0][1]],
'NN second weight avg.': [np.mean(nn_coef, axis=0)[0][1]],
'NN second weight std.': [np.std(nn_coef, ddof=1, axis=0)[0][1]],
}), ignore_index=True)
# plot the results
fig = make_subplots(rows=3, cols=1, subplot_titles=['Intercept', 'First Weight', 'Second Weight'])
fig.add_trace(go.Scatter(
x=output['sample size'],
y=[true_intercept] * output.shape[0],
mode='lines',
line=dict(color='rgb(82, 188, 163)', dash='dot', width=1),
legendgroup='True Value',
name='True Value',
showlegend=True,
), row=1, col=1)
fig.add_trace(go.Scatter(
x=output['sample size'],
y=output['LR intercept avg.'] + output['LR intercept std.'],
mode='lines',
line=dict(color='rgba(229, 134, 6, 0.2)'),
legendgroup='Logistic Regression',
showlegend=False,
), row=1, col=1)
fig.add_trace(go.Scatter(
x=output['sample size'],
y=output['LR intercept avg.'] - output['LR intercept std.'],
mode='lines',
fill='tonexty',
fillcolor='rgba(229, 134, 6, 0.2)',
line=dict(color='rgba(229, 134, 6, 0.2)'),
legendgroup='Logistic Regression',
showlegend=False,
), row=1, col=1)
fig.add_trace(go.Scatter(
x=output['sample size'],
y=output['LR intercept avg.'],
mode='lines',
line=dict(color='rgb(229, 134, 6)', dash='dot', width=1),
legendgroup='Logistic Regression',
name='Logistic Regression',
showlegend=True,
), row=1, col=1)
fig.add_trace(go.Scatter(
x=output['sample size'],
y=output['NN intercept avg.'] + output['NN intercept std.'],
mode='lines',
line=dict(color='rgba(93, 105, 177, 0.2)'),
legendgroup='Logistic Regression',
showlegend=False,
), row=1, col=1)
fig.add_trace(go.Scatter(
x=output['sample size'],
y=output['NN intercept avg.'] - output['NN intercept std.'],
mode='lines',
fill='tonexty',
fillcolor='rgba(93, 105, 177, 0.2)',
line=dict(color='rgba(93, 105, 177, 0.2)'),
legendgroup='Logistic Regression',
showlegend=False,
), row=1, col=1)
fig.add_trace(go.Scatter(
x=output['sample size'],
y=output['NN intercept avg.'],
mode='lines',
line=dict(color='rgb(93, 105, 177)', dash='dot', width=1),
legendgroup='MLP Regression',
name='MLP Regression',
showlegend=True,
), row=1, col=1)
fig.update_xaxes(
title='Sample Size',
type='category',
mirror=True,
linecolor='#d9d9d9',
showgrid=False,
zeroline=False,
row=1, col=1
)
fig.update_yaxes(
title='Estimate',
mirror=True,
linecolor='#d9d9d9',
showgrid=False,
zeroline=False,
row=1, col=1
)
fig.add_trace(go.Scatter(
x=output['sample size'],
y=[true_weights[0]] * output.shape[0],
mode='lines',
line=dict(color='rgb(82, 188, 163)', dash='dot', width=1),
legendgroup='True Value',
showlegend=False,
), row=2, col=1)
fig.add_trace(go.Scatter(
x=output['sample size'],
y=output['LR first weight avg.'] + output['LR first weight std.'],
mode='lines',
line=dict(color='rgba(229, 134, 6, 0.2)'),
legendgroup='Logistic Regression',
showlegend=False,
), row=2, col=1)
fig.add_trace(go.Scatter(
x=output['sample size'],
y=output['LR first weight avg.'] - output['LR first weight std.'],
mode='lines',
fill='tonexty',
fillcolor='rgba(229, 134, 6, 0.2)',
line=dict(color='rgba(229, 134, 6, 0.2)'),
legendgroup='Logistic Regression',
showlegend=False,
), row=2, col=1)
fig.add_trace(go.Scatter(
x=output['sample size'],
y=output['LR first weight avg.'],
mode='lines',
line=dict(color='rgb(229, 134, 6)', dash='dot', width=1),
legendgroup='Logistic Regression',
showlegend=False,
), row=2, col=1)
fig.add_trace(go.Scatter(
x=output['sample size'],
y=output['NN first weight avg.'] + output['NN first weight std.'],
mode='lines',
line=dict(color='rgba(93, 105, 177, 0.2)'),
legendgroup='MLP Regression',
showlegend=False,
), row=2, col=1)
fig.add_trace(go.Scatter(
x=output['sample size'],
y=output['NN first weight avg.'] - output['NN first weight std.'],
mode='lines',
fill='tonexty',
fillcolor='rgba(93, 105, 177, 0.2)',
line=dict(color='rgba(93, 105, 177, 0.2)'),
legendgroup='MLP Regression',
showlegend=False,
), row=2, col=1)
fig.add_trace(go.Scatter(
x=output['sample size'],
y=output['NN first weight avg.'],
mode='lines',
line=dict(color='rgb(93, 105, 177)', dash='dot', width=1),
legendgroup='MLP Regression',
showlegend=False,
), row=2, col=1)
fig.update_xaxes(
title='Sample Size',
type='category',
mirror=True,
linecolor='#d9d9d9',
showgrid=False,
zeroline=False,
row=2, col=1
)
fig.update_yaxes(
title='Estimate',
mirror=True,
linecolor='#d9d9d9',
showgrid=False,
zeroline=False,
row=2, col=1
)
fig.add_trace(go.Scatter(
x=output['sample size'],
y=[true_weights[1]] * output.shape[0],
mode='lines',
line=dict(color='rgb(82, 188, 163)', dash='dot', width=1),
legendgroup='True Value',
showlegend=False,
), row=3, col=1)
fig.add_trace(go.Scatter(
x=output['sample size'],
y=output['LR second weight avg.'] + output['LR second weight std.'],
mode='lines',
line=dict(color='rgba(229, 134, 6, 0.2)'),
legendgroup='Logistic Regression',
showlegend=False,
), row=3, col=1)
fig.add_trace(go.Scatter(
x=output['sample size'],
y=output['LR second weight avg.'] - output['LR second weight std.'],
mode='lines',
fill='tonexty',
fillcolor='rgba(229, 134, 6, 0.2)',
line=dict(color='rgba(229, 134, 6, 0.2)'),
legendgroup='Logistic Regression',
showlegend=False,
), row=3, col=1)
fig.add_trace(go.Scatter(
x=output['sample size'],
y=output['LR second weight avg.'],
mode='lines',
line=dict(color='rgb(229, 134, 6)', dash='dot', width=1),
legendgroup='Logistic Regression',
showlegend=False,
), row=3, col=1)
fig.add_trace(go.Scatter(
x=output['sample size'],
y=output['NN second weight avg.'] + output['NN second weight std.'],
mode='lines',
line=dict(color='rgba(93, 105, 177, 0.2)'),
legendgroup='MLP Regression',
showlegend=False,
), row=3, col=1)
fig.add_trace(go.Scatter(
x=output['sample size'],
y=output['NN second weight avg.'] - output['NN second weight std.'],
mode='lines',
fill='tonexty',
fillcolor='rgba(93, 105, 177, 0.2)',
line=dict(color='rgba(93, 105, 177, 0.2)'),
legendgroup='MLP Regression',
showlegend=False,
), row=3, col=1)
fig.add_trace(go.Scatter(
x=output['sample size'],
y=output['NN second weight avg.'],
mode='lines',
line=dict(color='rgb(93, 105, 177)', dash='dot', width=1),
legendgroup='MLP Regression',
showlegend=False,
), row=3, col=1)
fig.update_xaxes(
title='Sample Size',
type='category',
mirror=True,
linecolor='#d9d9d9',
showgrid=False,
zeroline=False,
row=3, col=1
)
fig.update_yaxes(
title='Estimate',
mirror=True,
linecolor='#d9d9d9',
showgrid=False,
zeroline=False,
row=3, col=1
)
fig.update_layout(
plot_bgcolor='white',
paper_bgcolor='white',
legend=dict(x=0, y=1.125, orientation='h'),
font=dict(family='Arial', size=6),
margin=dict(t=40, l=20, r=20, b=20)
)
fig.update_annotations(
font=dict(family='Arial', size=8)
)
# fig.write_image('LR_MLP_comparison.png', engine='orca', scale=4, height=500, width=400)
fig.write_image('LR_MLP_comparison.png', engine='kaleido', scale=4, height=500, width=400)
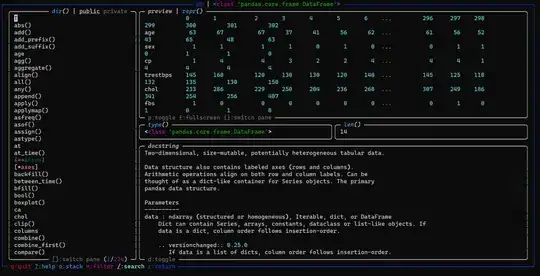