"Moments" can be strange things. They're building blocks and show up most often in statistics.
It helps to have a little background in statistics, and see the application of those calculations to image data, which can be considered a set of points. If you've ever calculated the weighted average or "centroid" of something, you'll recognize some of the sums that show up in "moments".
Higher order moments can be building blocks to higher statistical measures such as covariance and skewness.
Using covariance, you can calculate the major axis of your set of points, or your arrow in this case.
Using skewness, you can figure out which side of a distribution is heavier than the other... i.e. which side is the arrow's tip and which is its tail.
This should give you a very precise angle. The scale/radius however is best estimated using other ways. You'll notice that the radius estimated from the area of the arrow fluctuates a little. You could find the points belonging to the arrow that are furthest away from the center, and take that as a somewhat stable length.
Here's a longish program that implements the two ideas above and shows the direction of an arrow:
#!/usr/bin/env python3
import os
import sys
import numpy as np
import cv2 as cv
# utilities to convert between 2D vectors and complex numbers
# complex numbers are handy for rotating stuff
def to_complex(vec):
assert vec.shape[-1] == 2
if vec.dtype == np.float32:
return vec.view(np.complex64)
elif vec.dtype == np.float64:
return vec.view(np.complex128)
else:
assert False, vec.dtype
def from_complex(cplx):
if cplx.dtype == np.complex64:
return cplx.view(np.float32)
elif cplx.dtype == np.complex128:
return cplx.view(np.float64)
else:
assert False, cplx.dtype
# utilities for drawing with fractional bits of position
# just to make a pretty picture
def iround(val):
return int(round(val))
def ipt(vec, shift=0):
if isinstance(vec, (int, float)):
return iround(vec * 2**shift)
elif isinstance(vec, (tuple, list, np.ndarray)):
return tuple(iround(el * 2**shift) for el in vec)
else:
assert False, type(vec)
# utilities for affine transformation
# just to make a pretty picture
def rotate(degrees=0):
# we want positive rotation
# meaning move +x towards +y
# getRotationMatrix2D does it differently
result = np.eye(3).astype(np.float32)
result[0:2, 0:3] = cv.getRotationMatrix2D(center=(0,0), angle=-degrees, scale=1.0)
return result
def translate(dx=0, dy=0):
result = np.eye(3).astype(np.float32)
result[0:2,2] = [dx, dy]
return result
# main logic
def calculate_direction(im):
# using "nonzero" (default behavior) is a little noisy
mask = (im >= 128)
m = cv.moments(mask.astype(np.uint8), binaryImage=True)
# easier access... see below for details
m00 = m['m00']
m10 = m['m10']
m01 = m['m01']
mu00 = m00
mu20 = m['mu20']
mu11 = m['mu11']
mu02 = m['mu02']
nu30 = m['nu30']
nu03 = m['nu03']
# that's just the centroid
cx = m10 / m00
cy = m01 / m00
centroid = np.array([cx, cy]) # as a vector
# and that's the size in pixels:
size = m00
# and that's an approximate "radius", if it were a circle which it isn't
radius = (size / np.pi) ** 0.5
# (since the "size" in pixels can fluctuate due to resampling, so will the "radius")
# wikipedia helpfully mentions "image orientation" as an example:
# https://en.wikipedia.org/wiki/Image_moment#Examples_2
# we'll use that for the major axis
mup20 = mu20 / mu00
mup02 = mu02 / mu00
mup11 = mu11 / mu00
theta = 0.5 * np.arctan2(2 * mup11, mup20 - mup02)
#print(f"angle: {theta / np.pi * 180:+6.1f} degrees")
# we only have the axis, not yet the direction
# we will assess "skewness" now
# https://en.wikipedia.org/wiki/Skewness#Definition
# note how "positive" skewness appears in a distribution:
# it points away from the heavy side, towards the light side
# fortunately, cv.moments() also calculates those "standardized moments"
# https://en.wikipedia.org/wiki/Standardized_moment#Standard_normalization
skew = np.array([nu30, nu03])
#print("skew:", skew)
# we'll have to *rotate* that so it *roughly* lies along the x axis
# then assess which end is the heavy/light end
# then use that information to maybe flip the axis,
# so it points in the direction of the arrow
skew_complex = to_complex(skew) # reinterpret two reals as one complex number
rotated_skew_complex = skew_complex * np.exp(1j * -theta) # rotation
rotated_skew = from_complex(rotated_skew_complex)
#print("rotated skew:", rotated_skew)
if rotated_skew[0] > 0: # pointing towards tail
theta = (theta + np.pi) % (2*np.pi) # flip direction 180 degrees
else: # pointing towards head
pass
print(f"angle: {theta / np.pi * 180:+6.1f} degrees")
# construct a vector that points like the arrow in the picture
direction = np.exp([1j * theta])
direction = from_complex(direction)
return (radius, centroid, direction)
def draw_a_picture(im, radius, centroid, direction):
height, width = im.shape[:2]
# take the source at half brightness
canvas = cv.cvtColor(im // 2, cv.COLOR_GRAY2BGR)
shift = 4 # prettier drawing
cv.circle(canvas,
center=ipt(centroid, shift),
radius=ipt(radius, shift),
thickness=iround(radius * 0.1),
color=(0,0,255),
lineType=cv.LINE_AA,
shift=shift)
# (-direction) meaning point the *opposite* of the arrow's direction, i.e. towards tail
cv.line(canvas,
pt1=ipt(centroid + direction * radius * -3.0, shift),
pt2=ipt(centroid + direction * radius * +3.0, shift),
thickness=iround(radius * 0.05),
color=(0,255,255),
lineType=cv.LINE_AA,
shift=shift)
cv.line(canvas,
pt1=ipt(centroid + (-direction) * radius * 3.5, shift),
pt2=ipt(centroid + (-direction) * radius * 4.5, shift),
thickness=iround(radius * 0.15),
color=(0,255,255),
lineType=cv.LINE_AA,
shift=shift)
return canvas
if __name__ == '__main__':
imfile = sys.argv[1] if len(sys.argv) >= 2 else "p7cmR.png"
src = cv.imread(imfile, cv.IMREAD_GRAYSCALE)
src = 255 - src # invert (white arrow on black background)
height, width = src.shape[:2]
diagonal = np.hypot(height, width)
outsize = int(np.ceil(diagonal * 1.3)) # fudge factor
cv.namedWindow("arrow", cv.WINDOW_NORMAL)
cv.resizeWindow("arrow", 5*outsize, 5*outsize)
angle = 0 # degrees
increment = +1
do_spin = True
while True:
print(f"{angle:+.0f} degrees")
M = translate(dx=+outsize/2, dy=+outsize/2) @ rotate(degrees=angle) @ translate(dx=-width/2, dy=-height/2)
im = cv.warpAffine(src, M=M[:2], dsize=(outsize, outsize), flags=cv.INTER_CUBIC, borderMode=cv.BORDER_REPLICATE)
# resampling introduces blur... except when it's an even number like 0 degrees, 90 degrees, ...
# so at even rotations, things will jump a little.
# this rotation is only for demo purposes
(radius, centroid, direction) = calculate_direction(im)
canvas = draw_a_picture(im, radius, centroid, direction)
cv.imshow("arrow", canvas)
if do_spin:
angle = (angle + increment) % 360
print()
key = cv.waitKeyEx(30 if do_spin else -1)
if key == -1:
continue
elif key in (0x0D, 0x20): # ENTER (CR), SPACE
do_spin = not do_spin # toggle spinning
elif key == 27: # ESC
break # end program
elif key == 0x250000: # VK_LEFT
increment = -abs(increment)
angle += increment
elif key == 0x270000: # VK_RIGHT
increment = +abs(increment)
angle += increment
else:
print(f"key 0x{key:02x}")
cv.destroyAllWindows()
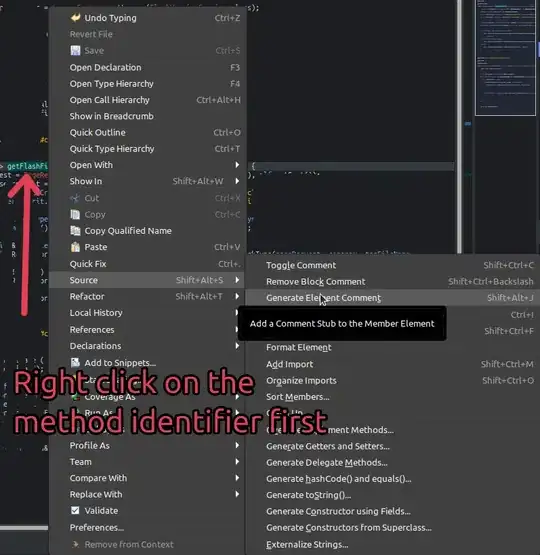