The issue is that BeautifulSoup won't see any results besides those for the first page. BeautifulSoup is just an XML/HTML parser, it's not a headless browser or JavaScript-capable runtime environment that can run JavaScript asynchronously. When you make a simple HTTP GET request to your page, the response is an HTML document, in which the first page's results are directly baked into the HTML. These contents are baked into the document at the time the server served the document to you, so BeautifulSoup can see these elements no problem. All the other pages of results, however, are more tricky.
View the page in a browser. While logging your network traffic, click on the "next" button to view the next page's results. If you're filtering your traffic by XHR/Fetch
requests only, you'll notice an HTTP POST request being made to an ASP.NET server, the response of which is HTML containing JavaScript containing JSON containing HTML. It's this nested HTML structure that represents the new content with which to update the table. Clicking this button doesn't actually take you to a different URL - the contents of the table simply change. The DOM is being updated/populated asynchronously using JavaScript, which is not uncommon.
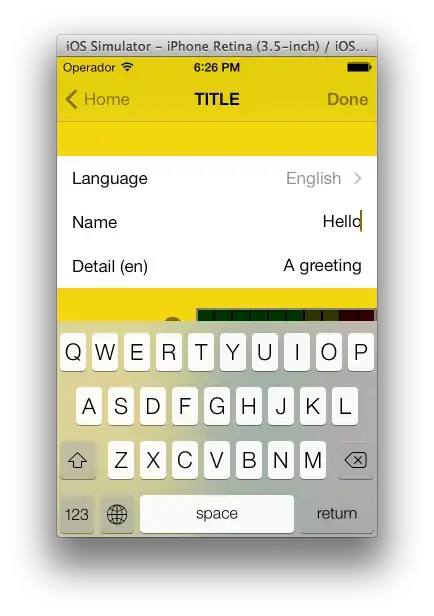
The challenge, then, is to mimic these requests and parse the response to extract the HREFs of only those links in which you're interested. I would split this up into three distinct scripts:
- One script to generate a
.txt
file of all sub-page URLs (these
would be the URLs you navigate to when clicking links like "Agenda
and Minutes",
example)
- One script to read from that
.txt
file, make requests to each URL,
and extract the HREF to the PDF on that page (if one is available).
These direct URLs to PDFs will be saved in another .txt
file.
- A script to read from the PDF-URL
.txt
file, and perform PDF
analysis.
You could combine scripts one and two if you really want to. I felt like splitting it up.
The first script makes an initial request to the main page to get some necessary cookies, and to extract a hidden input __OSVSTATE
that's baked into the HTML which the ASP.NET server cares about in our future requests. It then simulates "clicks" on the "next" button by sending HTTP POST requests to a specific ASP.NET server endpoint. We keep going until we can't find a "next" button on the page anymore. It turns out there are around ~260 pages of results in total. For each of these 260 responses, we parse the response, pull the HTML out of it, and extract the HREFs. We only keep those tags whose HREF ends with the substring ".htm", and whose text contains the substring "minute" (case-insensitive). We then write all HREFs to a text file page_urls.txt
. Some of these will be duplicated for some reason, and other's end up being invalid links, but we'll worry about that later. Here's the entire generated text file.
def get_urls():
import requests
from bs4 import BeautifulSoup as Soup
import datetime
import re
import json
# Start by making the initial request to store the necessary cookies in a session
# Also, retrieve the __OSVSTATE
url = "https://covapp.vancouver.ca/councilMeetingPublic/CouncilMeetings.aspx?SearchType=3"
headers = {
"user-agent": "Mozilla/5.0"
}
session = requests.Session()
response = session.get(url, headers=headers)
response.raise_for_status()
soup = Soup(response.content, "html.parser")
osv_state = soup.select_one("input[id=\"__OSVSTATE\"]")["value"]
# Get all results from all pages
url = "https://covapp.vancouver.ca/councilMeetingPublic/CouncilMeetings.aspx"
headers = {
"user-agent": "Mozilla/5.0",
"x-requested-with": "XMLHttpRequest"
}
payload = {
"__EVENTTARGET": "LiverpoolTheme_wt93$block$wtMainContent$RichWidgets_wt132$block$wt28",
"__AJAX": "980,867,LiverpoolTheme_wt93_block_wtMainContent_RichWidgets_wt132_block_wt28,745,882,0,277,914,760,"
}
while True:
params = {
"_ts": round(datetime.datetime.now().timestamp())
}
payload["__OSVSTATE"] = osv_state
response = session.post(url, params=params, headers=headers, data=payload)
response.raise_for_status()
pattern = "OsJSONUpdate\\(({\"outers\":{[^\\n]+})\\)//\\]\\]"
jsn = re.search(pattern, response.text).group(1)
data = json.loads(jsn)
osv_state = data["hidden"]["__OSVSTATE"]
html = data["outers"]["LiverpoolTheme_wt93_block_wtMainContent_wtTblCommEventTable_Wrapper"]["inner"]
soup = Soup(html, "html.parser")
# Select only those a-tags whose href attribute ends with ".htm" and whose text contains the substring "minute"
tags = soup.select("a[href$=\".htm\"]")
hrefs = [tag["href"] for tag in tags if "minute" in tag.get_text().casefold()]
yield from hrefs
page_num = soup.select_one("a.ListNavigation_PageNumber").get_text()
records_message = soup.select_one("div.Counter_Message").get_text()
print("Page #{}:\n\tProcessed {}, collected {} URL(s)\n".format(page_num, records_message, len(hrefs)))
if soup.select_one("a.ListNavigation_Next") is None:
break
def main():
with open("page_urls.txt", "w") as file:
for url in get_urls():
file.write(url + "\n")
return 0
if __name__ == "__main__":
import sys
sys.exit(main())
The second script reads the output file of the previous one, and makes a request to each URL in the file. Some of these will be invalid, some need to be cleaned up in order to be used, many will be duplicates, some will be valid but won't contain a link to a PDF, etc. We visit each page and extract the PDF URL, and save each in a file. In the end I've managed to collect 287 usable PDF URLs. Here is the generated text file.
def get_pdf_url(url):
import requests
from bs4 import BeautifulSoup as Soup
url = url.replace("/ctyclerk", "")
base_url = url[:url.rfind("/")+1]
headers = {
"user-agent": "Mozilla/5.0"
}
try:
response = requests.get(url, headers=headers)
response.raise_for_status()
except requests.exceptions.HTTPError:
return ""
soup = Soup(response.content, "html.parser")
pdf_tags = soup.select("a[href$=\".pdf\"]")
tag = next((tag for tag in pdf_tags if "minute" in tag.get_text()), None)
if tag is None:
return ""
return tag["href"] if tag["href"].startswith("http") else base_url + tag["href"]
def main():
with open("page_urls.txt", "r") as file:
page_urls = set(file.read().splitlines())
with open("pdf_urls.txt", "w") as file:
for count, pdf_url in enumerate(map(get_pdf_url, page_urls), start=1):
if pdf_url:
status = "Success"
file.write(pdf_url + "\n")
file.flush()
else:
status = "Skipped"
print("{}/{} - {}".format(count, len(page_urls), status))
return 0
if __name__ == "__main__":
import sys
sys.exit(main())
The third script would read from the pdf_urls.txt
file, make a request to each URL, and then interpret the response bytes as a PDF:
def main():
import requests
from io import BytesIO
from PyPDF2 import PdfFileReader
with open("pdf_urls.txt", "r") as file:
pdf_urls = file.read().splitlines()
for pdf_url in pdf_urls:
response = requests.get(pdf_url)
response.raise_for_status()
content = BytesIO(response.content)
reader = PdfFileReader(content)
# do stuff with reader
return 0
if __name__ == "__main__":
import sys
sys.exit(main())