Let's say I have a table that looks like this:
table = pd.DataFrame(
columns=["Name", "Size", "Color"],
data=[['A', 1, 'Red'], ['B', 2, 'Green'], ['C', 3, 'Blue']]
)
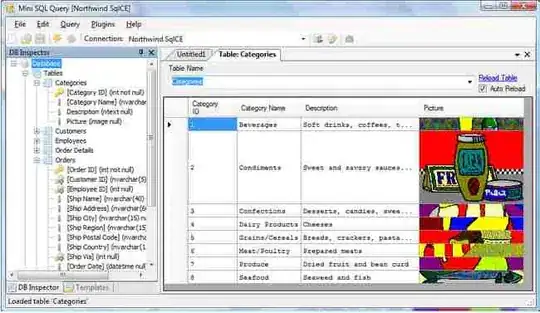
And a lookup table that looks like this:
lookup = pd.DataFrame(
columns=["Color", "Source", "Lead Days"],
data=[["Red", "Europe", 2],
["Green", "Europe", 3],
["Blue", "US", 1],
["Yellow", "Europe", 2]]
)
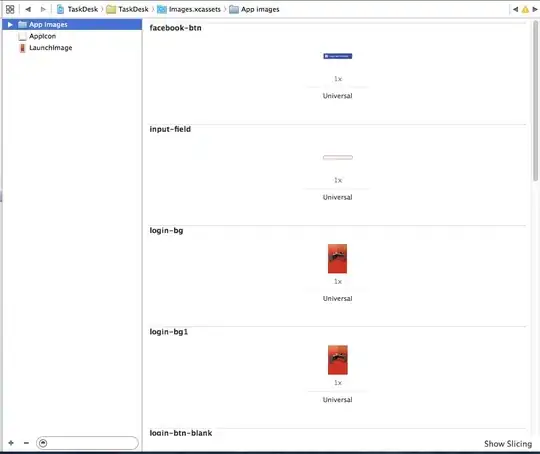
How might I add columns "Source" and "Lead Days" to table
by looking up "Color" from lookup
?
A story sometimes helps.
table
has all the items I need to order.
lookup
has where I order them from and how long it takes.
I want to transform table
so that it can show the "Source" and "Lead Days" for each item I need to order.
Here's what the final table should look like:

Note: while I'm sure there's a way to do this with merge, or top level table operations. In the spirit of Minimally Sufficient Pandas, and to avoid the huge kluge that is pandas' over-provisioning of operations, I'd prefer to do it with apply
. Apply is nice because it's easy to consistently reach for apply
in all situations.
Here's my current approach, but it results in the error ValueError: Columns must be same length as key
To me, this makes little sense, since I'm returning a list of length 2 and putting it into two columns. But I'm sure pandas has its reasons for being anti-intuitive here.
lookup_columns = ["Source", "Lead Days"]
table[lookup_columns] = table.apply(
lambda row:
lookup.query('`Color` == "{color}"'.format(color=row["Color"])).loc[:, lookup_columns].values[0]
, axis = 1)