- this is an enhancement to Plotly.py Sankey Diagrams - Controlling Node Destination
- your stated requirement is to create columns by date, use date part of concatenated sankey node for column
- clearly formatting can be further beautified. This shows how columns can be defined and annotated
sample data
from_date |
to_date |
from_type |
to_type |
value |
source |
target |
2022-01-01 00:00:00 |
2022-02-01 00:00:00 |
Consumer |
Home Office |
3 |
Consumer_20220101 |
Home Office_20220201 |
2022-01-01 00:00:00 |
2022-03-01 00:00:00 |
Consumer |
Corporate |
6 |
Consumer_20220101 |
Corporate_20220301 |
2022-01-01 00:00:00 |
2022-03-01 00:00:00 |
Small Business |
Corporate |
21 |
Small Business_20220101 |
Corporate_20220301 |
2022-01-01 00:00:00 |
2022-04-01 00:00:00 |
Consumer |
Home Office |
14 |
Consumer_20220101 |
Home Office_20220401 |
2022-02-01 00:00:00 |
2022-03-01 00:00:00 |
Corporate |
Consumer |
20 |
Corporate_20220201 |
Consumer_20220301 |
solution
import pandas as pd
import numpy as np
import plotly.graph_objects as go
ms = pd.date_range("1-jan-2022", freq="MS", periods=4)
types = ["Consumer", "Home Office", "Corporate", "Small Business"]
# simulate some data, date and type to date and type
s = 50
df = pd.DataFrame(
{
"from_date": np.random.choice(ms, s),
"to_date": np.random.choice(ms, s),
"from_type": np.random.choice(types, s),
"to_type": np.random.choice(types, s),
"value": np.random.randint(1, 20, s),
}
).loc[
# remove invalid combis from random generation
lambda d: (d["to_date"] > d["from_date"]) & (d["from_type"] != d["to_type"])
].groupby(
["from_date", "to_date", "from_type", "to_type"], as_index=False
).sum()
# start of solution, define source and target of sankey from column concat
df = df.assign(source=lambda d: d["from_type"] + "_" + d["from_date"].dt.strftime("%Y%m%d"),
target=lambda d: d["to_type"] + "_" + d["to_date"].dt.strftime("%Y%m%d"),
)
def factorize(s):
a = pd.factorize(s, sort=True)[0]
return (a + 0.01) / (max(a) + 0.1)
# unique nodes
nodes = np.unique(df[["source", "target"]], axis=None)
nodes = pd.Series(index=nodes, data=range(len(nodes)))
# work out positioning of nodes
nodes = (
nodes.to_frame("id")
.assign(
y=lambda d: factorize(d.index.to_series().apply(lambda s: s.split("_")[0])),
x=lambda d: factorize(d.index.to_series().apply(lambda s: s.split("_")[1])),
)
)
# now simple job of building sankey
fig = go.Figure(
go.Sankey(
arrangement="snap",
node={"label": nodes.index.to_series().apply(lambda s: s.split("_")[0]), "x": nodes["x"], "y": nodes["y"]},
link={
"source": nodes.loc[df["source"], "id"],
"target": nodes.loc[df["target"], "id"],
"value": df["value"],
},
)
)
for i, x in nodes["x"].drop_duplicates().iteritems():
fig.add_annotation(x=x, y=1.4, text=i.split("_")[1], showarrow=False)
fig
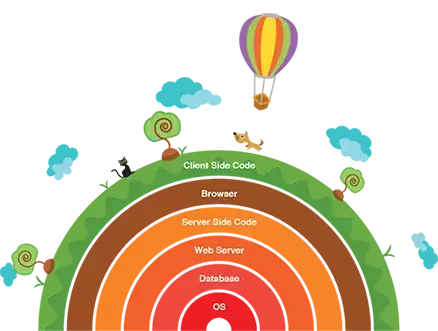