Expanding helpful comments from @r2evans and @Dave2e into an answer.
1) .Random.seed[1]
From ?.Random.seed
, it says:
".Random.seed
is an integer vector whose first element codes the
kind of RNG and normal generator. The lowest two decimal digits are in
0:(k-1)
where k
is the number of available RNGs. The hundreds
represent the type of normal generator (starting at 0), and the ten
thousands represent the type of discrete uniform sampler."
Therefore the first value doesn't change unless one changes the generator method (RNGkind
).
Here is a small demonstration of this for each of the available RNGkind
s:
library(tidyverse)
# available RNGkind options
kinds <- c(
"Wichmann-Hill",
"Marsaglia-Multicarry",
"Super-Duper",
"Mersenne-Twister",
"Knuth-TAOCP-2002",
"Knuth-TAOCP",
"L'Ecuyer-CMRG"
)
# test over multiple seeds
seeds <- c(1:3)
f <- function(kind, seed) {
# set seed with simulation parameters
set.seed(seed = seed, kind = kind)
# check value of first element in .Random.seed
return(.Random.seed[1])
}
# run on simulated conditions and compare value over different seeds
expand_grid(kind = kinds, seed = seeds) %>%
pmap(f) %>%
unlist() %>%
matrix(
ncol = length(seeds),
byrow = T,
dimnames = list(kinds, paste0("seed_", seeds))
)
#> seed_1 seed_2 seed_3
#> Wichmann-Hill 10400 10400 10400
#> Marsaglia-Multicarry 10401 10401 10401
#> Super-Duper 10402 10402 10402
#> Mersenne-Twister 10403 10403 10403
#> Knuth-TAOCP-2002 10406 10406 10406
#> Knuth-TAOCP 10404 10404 10404
#> L'Ecuyer-CMRG 10407 10407 10407
Created on 2022-01-06 by the reprex package (v2.0.1)
2) .Random.seed[2]
At least for the default "Mersenne-Twister"
method, .Random.seed[2]
is an index that indicates the current position in the random set. From the docs:
The ‘seed’ is a 624-dimensional set of 32-bit integers plus a current
position in that set.
This is updated when random processes using the seed are executed. However for other methods it the documentation doesn't mention something like this and there doesn't appear to be a clear trend in the same way.
See below for an example of changes in .Random.seed[2]
over iterative random process after set.seed()
.
library(tidyverse)
# available RNGkind options
kinds <- c(
"Wichmann-Hill",
"Marsaglia-Multicarry",
"Super-Duper",
"Mersenne-Twister",
"Knuth-TAOCP-2002",
"Knuth-TAOCP",
"L'Ecuyer-CMRG"
)
# create function to run random process and report .Random.seed[2]
t <- function(n = 1) {
p <- .Random.seed[2]
runif(n)
p
}
# create function to set seed and iterate a random process
f2 <- function(kind, seed = 1, n = 5) {
set.seed(seed = seed,
kind = kind)
replicate(n, t())
}
# set simulation parameters
trials <- 5
seeds <- 1:2
x <- expand_grid(kind = kinds, seed = seeds, n = trials)
# evaluate and report
x %>%
pmap_dfc(f2) %>%
mutate(n = paste0("trial_", 1:trials)) %>%
pivot_longer(-n, names_to = "row") %>%
pivot_wider(names_from = "n") %>%
select(-row) %>%
bind_cols(x[,1:2], .)
#> # A tibble: 14 x 7
#> kind seed trial_1 trial_2 trial_3 trial_4 trial_5
#> <chr> <int> <int> <int> <int> <int> <int>
#> 1 Wichmann-Hill 1 23415 8457 23504 2.37e4 2.28e4
#> 2 Wichmann-Hill 2 21758 27800 1567 2.58e4 2.37e4
#> 3 Marsaglia-Multicarry 1 1280795612 945095059 14912928 1.34e9 2.23e8
#> 4 Marsaglia-Multicarry 2 -897583247 -1953114152 2042794797 1.39e9 3.71e8
#> 5 Super-Duper 1 1280795612 -1162609806 -1499951595 5.51e8 6.35e8
#> 6 Super-Duper 2 -897583247 224551822 -624310 -2.23e8 8.91e8
#> 7 Mersenne-Twister 1 624 1 2 3 4
#> 8 Mersenne-Twister 2 624 1 2 3 4
#> 9 Knuth-TAOCP-2002 1 166645457 504833754 504833754 5.05e8 5.05e8
#> 10 Knuth-TAOCP-2002 2 967462395 252695483 252695483 2.53e8 2.53e8
#> 11 Knuth-TAOCP 1 1050415712 999978161 999978161 1.00e9 1.00e9
#> 12 Knuth-TAOCP 2 204052929 776729829 776729829 7.77e8 7.77e8
#> 13 L'Ecuyer-CMRG 1 1280795612 -169270483 -442010614 4.71e8 1.80e9
#> 14 L'Ecuyer-CMRG 2 -897583247 -1619336578 -714750745 2.10e9 -9.89e8
Created on 2022-01-06 by the reprex package (v2.0.1)
Here you can see that from the Mersenne-Twister
method, .Random.seed[2]
increments from it's maximum of 624
back to 1
and increased by the size of the random draw and that this is the same for set.seed(1)
and set.seed(2)
. However the same trend is not seen in the other methods. To illustrate the last point, see that runif(1)
increments .Random.seed[2]
by 1
while runif(2)
increments it by 2
:
# create function to run random process and report .Random.seed[2]
t <- function(n = 1) {
p <- .Random.seed[2]
runif(n)
p
}
set.seed(1, kind = "Mersenne-Twister")
replicate(9, t(1))
#> [1] 624 1 2 3 4 5 6 7 8
set.seed(1, kind = "Mersenne-Twister")
replicate(5, t(2))
#> [1] 624 2 4 6 8
Created on 2022-01-06 by the reprex package (v2.0.1)
3) Sequential Randoms
Because the index or state of .Random.seed
(apparently for all the RNG methods) advances according to the size of the 'random draw' (number of random values genearted from the .Random.seed
), it is possible to generate the same series of random numbers from the same seed in different sized increments. Furthermore, as long as you run the same random process at the same point in the sequence after setting the same seed, it seems that you will get the same result. Observe the following example:
# draw 3 at once
set.seed(1, kind = "Mersenne-Twister")
sample(100, 3, T)
#> [1] 68 39 1
# repeat single draw 3 times
set.seed(1, kind = "Mersenne-Twister")
sample(100, 1)
#> [1] 68
sample(100, 1)
#> [1] 39
sample(100, 1)
#> [1] 1
# draw 1, do something else, draw 1 again
set.seed(1, kind = "Mersenne-Twister")
sample(100, 1)
#> [1] 68
runif(1)
#> [1] 0.5728534
sample(100, 1)
#> [1] 1
Created on 2022-01-06 by the reprex package (v2.0.1)
4) Correlated Randoms
As we saw above, two random processes run at the same point after setting the same seed are expected to give the same result. However, even when you provide constraints on how similar the result can be (e.g. by changing the mean
of rnorm()
or even by providing different functions) it seems that the results are still perfectly correlated within their respective ranges.
# same function with different constraints
set.seed(1, kind = "Mersenne-Twister")
a <- runif(50, 0, 1)
set.seed(1, kind = "Mersenne-Twister")
b <- runif(50, 10, 100)
plot(a, b)
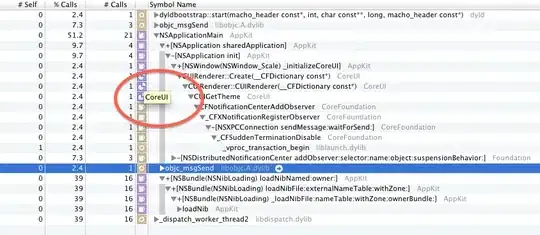
# different functions
set.seed(1, kind = "Mersenne-Twister")
d <- rnorm(50)
set.seed(1, kind = "Mersenne-Twister")
e <- rlnorm(50)
plot(d, e)
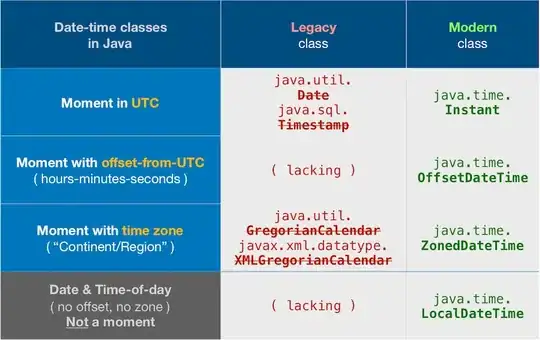
Created on 2022-01-06 by the reprex package (v2.0.1)