
The DFT (FFT being its algorithmic computation) is a dot product between a finite discrete number of samples N of an analogue signal s(t) (a function of time or space) and a set of basis vectors of complex exponentials (sin and cos functions). Although the sample is naturally finite and may show no periodicity, it is implicitly thought of as a periodically repeating discrete function. Even when dealing with real-valued signals (the usual situation) it is convenient to work with complex numbers (Euler's equation). It may be intimidating to implement the function on a signal with np.fft.fft(s)
only to get the output coefficients in complex numbers and get stuck in their interpretation. Some steps are essential:
What are the frequencies in the complex exponentials?
- The DFT does not necessarily preserve the sampling frequency in Hertz. The frequencies are indices (k).
- The indices k range from
0 to N - 1
and can be thought of as having units of cycles / set (the set being the N
samples of the signal s
). I will omit discussing the Nyquist limit, but for real signals the frequencies form a mirror image after N / 2, and given as negative decreasing values after that point (not a problem within the framework of implicit periodicity). The frequencies used in the FFT are not simply k, but k / N, thought of as having units of cycles / sample. See this reference. Example (reference): If a signal is sampled N = 5
times the frequencies are: np.fft.fftfreq(5)
, yielding [ 0 , 0.2, 0.4, -0.4, -0.2]
, i.e. [0/5, 1/5, 2/5, -2/5, -1/5]
.
- To convert these frequencies to meaningful units (e.g. Hetz or mm) the values in cycles/sample above will need to be divided by sampling interval T (e.g. distance in seconds between samples). Continuing with the example above, there is a built-in call:
np.fft.fftfreq(5, d=T)
: If the analogue signal s
is sampled 5
times at equidistant intervals T = 1/2
sec for a total sample of NT = 5 x 1/2 sec
, the normalized frequencies will be np.fft.fftfreq(5, d = 1/2)
, yielding [0 0.4 0.8 -0.8 -0.4]
or [0/NT, 1/NT, 2/NT, -2/NT, -1/NT]
.
- Either normalized or un-normalized frequencies are used to control angular frequencies (ω_m), expressed as ω_m = 2π k/NT. Note that
NT
is the total duration for
which the signal was sampled. The index k
does result in multiples of a fundamental frequency (ω-naught) corresponding to k = 1
- the frequency of (co-)sine wave that completes
exactly one oscillation over NT
(here).
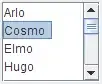
Magnitude, frequency and phase of the coefficients in the FFT
- Given the output of the FFT
S = fft.fft(s)
, the magnitude of the output coefficients (here) is just the Euclidean norm of the complex numbers in the output coefficients adjusted for the symmetry in real signals (x 2
) and for the number of samples 1/N
: magnitudes = 1/N * np.abs(S)
- The frequencies are matched to the call explained above
np.fft.fftfreq(N)
, or more expediently to incorporate the actual analogue frequency units, frequencies = np.fft.fftfreq(N, d=T)
.
- The phase of each coefficients is the angle of the complex number in polar form
phase = np.arctan(np.imag(S)/np.real(S))
How to find the dominant frequencies in the signal s in the FFT and their coefficients?
- Plotting aside, finding the index
k
corresponding the frequency with the highest magnitude can be accomplished as index = np.argmax(np.abs(S))
. To find the 4
indices with the highest magnitude, for example, the call is indices = np.argpartition(S,-4)[-4:]
.
- And finding the actual corresponding coefficient:
S[index]
with frequency freq_max = np.fft.fftfreq(N, d=T)[index]
.
Reproducing the original signal after obtaining the coefficients:
Reproducing s
through sines and cosines (p.150 in here):
Re = np.real(S[index])
Im = np.imag(S[index])
s_recon = Re * 2/N * np.cos(-2 * np.pi * freq_max * t) + abs(Im) * 2/N * np.sin(-2 * np.pi * freq_max * t)
Here is a complete example:
import numpy as np
import matplotlib.pyplot as plt
N = 10000 # Sample points
T = 1/5000 # Spacing
# Total duration N * T= 2
t = np.linspace(0.0, N*T, N, endpoint=False) # Time: Vector of 10,000 elements from 0 to N*T=2.
frequency = np.fft.fftfreq(t.size, d=T) # Normalized Fourier frequencies in spectrum.
f0 = 25 # Frequency of the sampled wave
phi = np.pi/8 # Phase
A = 50 # Amplitude
s = A * np.cos(2 * np.pi * f0 * t + phi) # Signal
S = np.fft.fft(s) # Unnormalized FFT
index = np.argmax(np.abs(S))
print(S[index])
magnitude = np.abs(S[index]) * 2/N
freq_max = frequency[index]
phase = np.arctan(np.imag(S[index])/np.real(S[index]))
print(f"magnitude: {magnitude}, freq_max: {freq_max}, phase: {phase}")
print(phi)
fig, [ax1,ax2] = plt.subplots(nrows=2, ncols=1, figsize=(10, 5))
ax1.plot(t,s, linewidth=0.5, linestyle='-', color='r', marker='o', markersize=1,markerfacecolor=(1, 0, 0, 0.1))
ax1.set_xlim([0, .31])
ax1.set_ylim([-51,51])
ax2.plot(frequency[0:N//2], 2/N * np.abs(S[0:N//2]), '.', color='xkcd:lightish blue', label='amplitude spectrum')
plt.xlim([0, 100])
plt.show()
Re = np.real(S[index])
Im = np.imag(S[index])
s_recon = Re*2/N * np.cos(-2 * np.pi * freq_max * t) + abs(Im)*2/N * np.sin(-2 * np.pi * freq_max * t)
fig = plt.figure(figsize=(10, 2.5))
plt.xlim(0,0.3)
plt.ylim(-51,51)
plt.plot(t,s_recon, linewidth=0.5, linestyle='-', color='r', marker='o', markersize=1,markerfacecolor=(1, 0, 0, 0.1))
plt.show()
s.all() == s_recon.all()