You may apply "contrast stretching".
The dynamic range of image_data
is about [0, 4095] - the minimum value is about 0, and the maximum value is about 4095 (2^12-1).
You are saving the image as 16 bits PNG.
When you display the PNG file, the viewer, assumes the maximum value is 2^16-1 (the dynamic range of 16 bits is [0, 65535]).
The viewer assumes 0 is black, 2^16-1 is white, and values in between scales linearly.
In your case the white pixels value is about 4095, so it translated to be a very dark gray in the [0, 65535] range.
The simplest solution is to multiply image_data
by 16
:
from medpy.io import load, save
image_data, image_header = load('image_path/c0001.mha')
save(image_data*16, 'image_save_path/new_image.png', image_header)
A more complicated solution is applying linear "contrast stretching".
We may transform the lower 1% of all pixel to 0, the upper 1% of the pixels to 2^16-1, and scale the pixels in between linearly.
import numpy as np
from medpy.io import load, save
image_data, image_header = load('image_path/c0001.mha')
tmp = image_data.copy()
tmp[tmp == 0] = np.median(tmp) # Ignore zero values by replacing them with median value (there are a lot of zeros in the margins).
tmp = tmp.astype(np.float32) # Convert to float32
# Get the value of lower and upper 1% of all pixels
lo_val, up_val = np.percentile(tmp, (1, 99)) # (for current sample: lo_val = 796, up_val = 3607)
# Linear stretching: Lower 1% goes to 0, upper 1% goes to 2^16-1, other values are scaled linearly
# Clipt to range [0, 2^16-1], round and convert to uint16
# https://stackoverflow.com/questions/49656244/fast-imadjust-in-opencv-and-python
img = np.round(((tmp - lo_val)*(65535/(up_val - lo_val))).clip(0, 65535)).astype(np.uint16) # (for current sample: subtract 796 and scale by 23.31)
img[image_data == 0] = 0 # Restore the original zeros.
save(img, 'image_save_path/new_image.png', image_header)
The above method enhance the contrast, but looses some of the original information.
In case you want higher contrast, you may use non-linear methods, improving the visibility, but loosing some "integrity".
Here is the "linear stretching" result (downscaled):
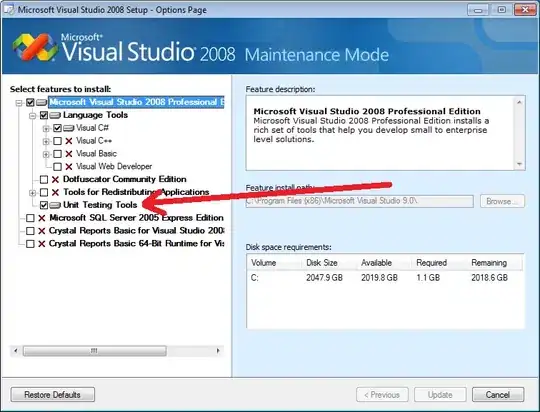