First of all : Use the solution from the other question you link to if you want to be safe. As R is call-by-value, forget about an "in-place" method that doesn't copy your dataframes in the memory.
One not advisable method of saving quite a bit of memory, is to pretend your dataframes are lists, coercing a list using a for-loop (apply will eat memory like hell) and make R believe it actually is a dataframe.
I'll warn you again : using this on more complex dataframes is asking for trouble and hard-to-find bugs. So be sure you test well enough, and if possible, avoid this as much as possible.
You could try following approach :
n1 <- 1000000
n2 <- 1000000
ncols <- 20
dtf1 <- as.data.frame(matrix(sample(n1*ncols), n1, ncols))
dtf2 <- as.data.frame(matrix(sample(n2*ncols), n1, ncols))
dtf <- list()
for(i in names(dtf1)){
dtf[[i]] <- c(dtf1[[i]],dtf2[[i]])
}
attr(dtf,"row.names") <- 1:(n1+n2)
attr(dtf,"class") <- "data.frame"
It erases rownames you actually had (you can reconstruct them, but check for duplicate rownames!). It also doesn't carry out all the other tests included in rbind.
Saves you about half of the memory in my tests, and in my test both the dtfcomb and the dtf are equal. The red box is rbind, the yellow one is my list-based approach.
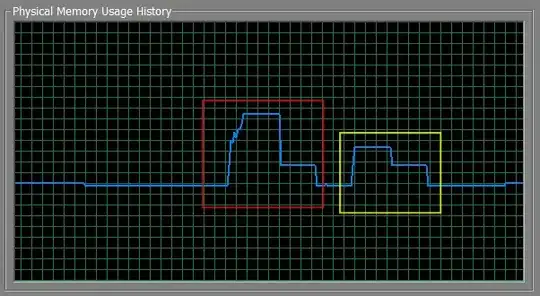
Test script :
n1 <- 3000000
n2 <- 3000000
ncols <- 20
dtf1 <- as.data.frame(matrix(sample(n1*ncols), n1, ncols))
dtf2 <- as.data.frame(matrix(sample(n2*ncols), n1, ncols))
gc()
Sys.sleep(10)
dtfcomb <- rbind(dtf1,dtf2)
Sys.sleep(10)
gc()
Sys.sleep(10)
rm(dtfcomb)
gc()
Sys.sleep(10)
dtf <- list()
for(i in names(dtf1)){
dtf[[i]] <- c(dtf1[[i]],dtf2[[i]])
}
attr(dtf,"row.names") <- 1:(n1+n2)
attr(dtf,"class") <- "data.frame"
Sys.sleep(10)
gc()
Sys.sleep(10)
rm(dtf)
gc()