Your function is wrong. If you want the troughs to be at odd multiples of 0.5
and the peaks to be at even multiples of 0.5
, and you want the value to be 1
and -1
, your function needs to be -4 * t + 1
between 0
and 0.5
, and 4 * t - 3
between 0.5
and 1
.
Also, your function returns 0
for t < 0
and for t > 1
, so your output doesn't match the expected output outside those bounds.
Since your if
conditions describe the correct behavior when you have only the part of the input after the decimal point, you can extract that part and use it instead of t
:
def F(t):
decimal_part = t % 1
if 0 <= decimal_part <= 0.5:
return -4*decimal_part + 1
else:
return 4*decimal_part - 3
Which gives this:
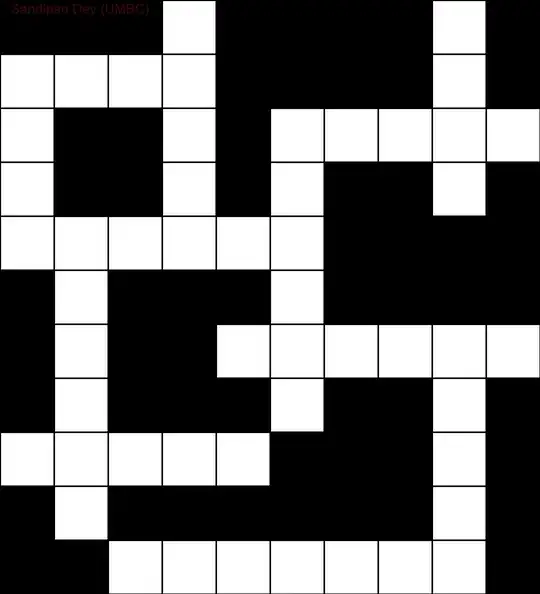
Remember to discretize the x-axis so it has enough points. I plotted the blue line with t = np.linspace(0, 4, 1000)
, and the red line with t = np.linspace(0, 4, 10)
and everything else remained the same.
The np.vectorize()
function doesn't really vectorize a function though. If you want to actually see speed improvements, you're going to have to vectorize the function yourself by expressing its logic as a vector operation.
def g(t):
dec = (t % 1) - 0.5
return (np.abs(dec) - 0.25) * 4
Both give the same plots, but timing the execution shows the difference:
import timeit
t1 = timeit.timeit('f(t)', setup='from __main__ import np, f, t', number=10000)
t2 = timeit.timeit('g(t)', setup='from __main__ import np, g, t', number=10000)
# t1 = 3.322270042001037
# t2 = 0.17450489799375646
print(t1 / t2)
# 19.038262422410074
The actually vectorized function is ~19x faster on my computer (Intel Python 3.7 with numpy+MKL on Macbook Air)