I'm trying to detect and draw a rectangular contour on every painting on for example this image:
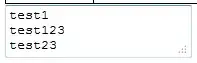
I followed some guides and did the following:
- Grayscale conversion
- Applied median blur
- Sharpen image
- Applied adaptive Threshold
- Applied Morphological Gradient
- Find contours
- Draw contours
And got the following result:
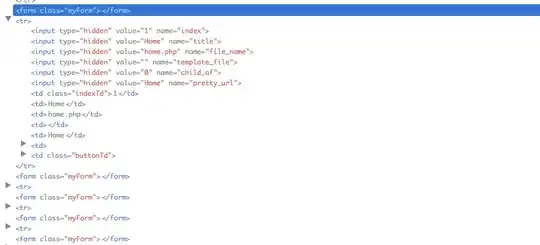
I know it's messy but is there a way to somehow detect and draw a contour around the paintings better?
Here is the code I used:
path = '<PATH TO THE PICTURE>'
#reading in and showing original image
image = cv2.imread(path)
image = cv2.resize(image,(880,600)) # resize was nessecary because of the large images
cv2.imshow("original", image)
cv2.waitKey(0)
cv2.destroyAllWindows()
# grayscale conversion
gray = cv2.cvtColor(image, cv2.COLOR_BGR2GRAY)
cv2.imshow("painting_gray", gray)
cv2.waitKey(0)
cv2.destroyAllWindows()
# we need to find a way to detect the edges better so we implement a couple of things
# A little help was found on stackoverflow: https://stackoverflow.com/questions/55169645/square-detection-in-image
median = cv2.medianBlur(gray,5)
cv2.imshow("painting_median_blur", median) #we use median blur to smooth the image
cv2.waitKey(0)
cv2.destroyAllWindows()
# now we sharpen the image with help of following URL: https://www.analyticsvidhya.com/blog/2021/08/sharpening-an-image-using-opencv-library-in-python/
kernel = np.array([[0, -1, 0],
[-1, 5,-1],
[0, -1, 0]])
image_sharp = cv2.filter2D(src=median, ddepth=-1, kernel=kernel)
cv2.imshow('painting_sharpend', image_sharp)
cv2.waitKey(0)
cv2.destroyAllWindows()
# now we apply adapptive thresholding
# thresholding: https://opencv24-python-tutorials.readthedocs.io/en/latest/py_tutorials/py_imgproc/py_thresholding/py_thresholding.html#adaptive-thresholding
thresh = cv2.adaptiveThreshold(src=image_sharp,maxValue=255,adaptiveMethod=cv2.ADAPTIVE_THRESH_GAUSSIAN_C,
thresholdType=cv2.THRESH_BINARY,blockSize=61,C=20)
cv2.imshow('thresholded image', thresh)
cv2.waitKey(0)
cv2.destroyAllWindows()
# lets apply a morphological transformation
kernel = np.ones((7,7),np.uint8)
gradient = cv2.morphologyEx(thresh, cv2.MORPH_GRADIENT, kernel)
cv2.imshow('dilated image', gradient)
cv2.waitKey(0)
cv2.destroyAllWindows()
# # lets now find the contours of the image
# # find contours: https://docs.opencv.org/4.x/dd/d49/tutorial_py_contour_features.html
contours, hierarchy = cv2.findContours(gradient, cv2.RETR_TREE, cv2.CHAIN_APPROX_SIMPLE)
print("contours: ", len(contours))
print("hierachy: ", len(hierarchy))
print(hierarchy)
cv2.drawContours(image, contours, -1, (0,255,0), 3)
cv2.imshow("contour image", image)
cv2.waitKey(0)
cv2.destroyAllWindows()
Tips, help or code is appreciated!