Another solution:
arr = np.array([0, 1, 0, 0, 0, 2, 2])
a = arr.argsort()
v, cnt = np.unique(arr, return_counts=True)
x = dict(zip(v, np.split(a, cnt.cumsum()[:-1])))
print(x)
Prints:
{0: array([0, 2, 3, 4]), 1: array([1]), 2: array([5, 6])}
But the speed-up depends on your data (how big is the array, how many unique elements are in the array...)
Some benchmark (Ubuntu 20.04 on AMD 3700x, Python 3.9.7, numpy==1.21.5):
import perfplot
NUM_UNIQUE_VALUES = 10
def make_data(n):
return np.random.randint(0, NUM_UNIQUE_VALUES, n)
def k1(arr):
vals = np.unique(arr)
return {val: np.where(arr == val)[0] for val in vals}
def k2(arr):
a = arr.argsort()
v, cnt = np.unique(arr, return_counts=True)
return dict(zip(v, np.split(a, cnt.cumsum()[:-1])))
perfplot.show(
setup=make_data,
kernels=[k1, k2],
labels=["Nico", "Andrej"],
equality_check=None,
n_range=[2 ** k for k in range(1, 25)],
xlabel="2**N",
logx=True,
logy=True,
)
With NUM_UNIQUE_VALUES = 10
:
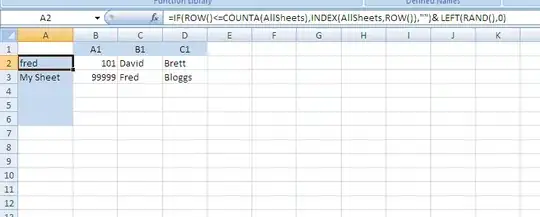
With NUM_UNIQUE_VALUES = 1024
:
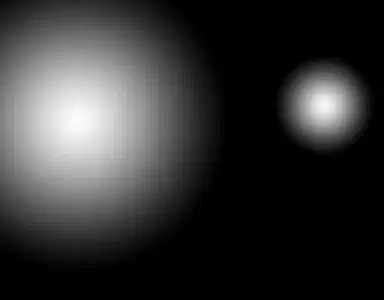
Getting bins from array of 1 million elements (changing only number of unique values):
def make_data(n):
return np.random.randint(0, n, 1_000_000)
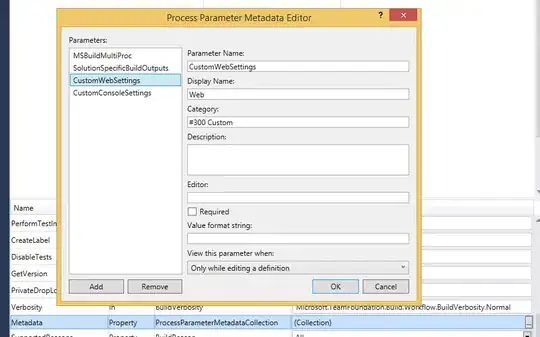