The problem here is that the fit algorithm starts with "wrong" approximations for parameters a, k, and b, so during the minimalization it finds a local minimum, not the global one. You can improve the result if you provide the algorithm with starting values, which are close to the optimal ones. For example, let's start with the following parameters:
gnuplot> a=47.5087
gnuplot> k=0.226
gnuplot> b=1.0016
gnuplot> f(x)=a*log(k*x+b)
gnuplot> fit f(x) 'R_B.txt' via a,k,b
....
....
....
After 40 iterations the fit converged.
final sum of squares of residuals : 16.2185
rel. change during last iteration : -7.6943e-06
degrees of freedom (FIT_NDF) : 18
rms of residuals (FIT_STDFIT) = sqrt(WSSR/ndf) : 0.949225
variance of residuals (reduced chisquare) = WSSR/ndf : 0.901027
Final set of parameters Asymptotic Standard Error
======================= ==========================
a = 35.0415 +/- 2.302 (6.57%)
k = 0.372381 +/- 0.0461 (12.38%)
b = 1.07012 +/- 0.02016 (1.884%)
correlation matrix of the fit parameters:
a k b
a 1.000
k -0.994 1.000
b 0.467 -0.531 1.000
The resulting plot is
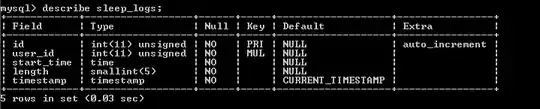
Now the question is how you can find "good" initial approximations for your parameters? Well, you start with
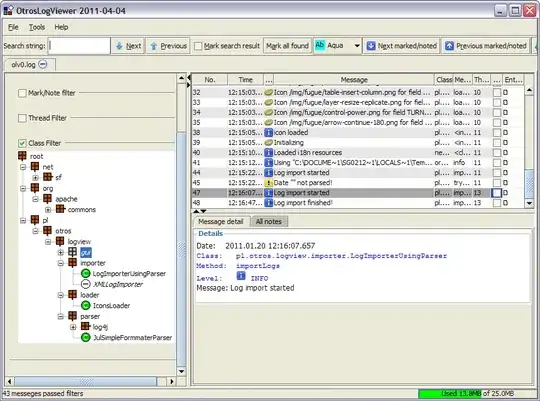
If you differentiate this equation you get
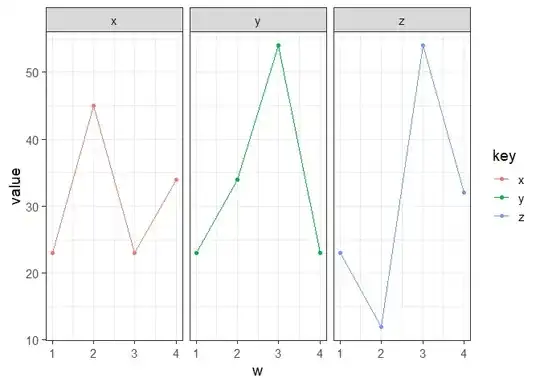
or
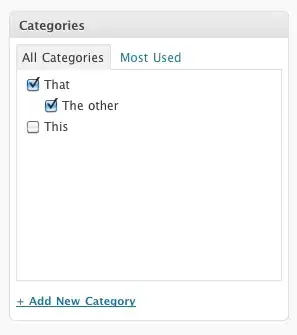
The left-hand side of this equation is some constant 'C', so the expression in the right-hand side should be equal to this constant as well:
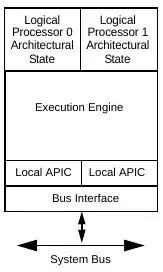
In other words, the reciprocal of the derivative of your data should be approximated by a linear function. So, from your data x[i]
, y[i]
you can construct the reciprocal derivatives x[i]
, (x[i+1]-x[i])/(y[i+1]-y[i])
and the linear fit of these data:
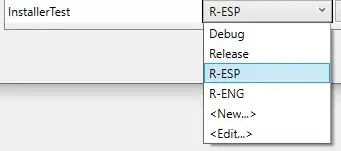
The fit gives the following values:
C*k = 0.0236179
C*b = 0.106268
Now, we need to find the values for a
, and C
. Let's say, that we want the resulting graph to pass close to the starting and the ending point of our dataset. That means, that we want
a*log(k*x1 + b) = y1
a*log(k*xn + b) = yn
Thus,
a*log((C*k*x1 + C*b)/C) = a*log(C*k*x1 + C*b) - a*log(C) = y1
a*log((C*k*xn + C*b)/C) = a*log(C*k*xn + C*b) - a*log(C) = yn
By subtracting the equations we get the value for a:
a = (yn-y1)/log((C*k*xn + C*b)/(C*k*x1 + C*b)) = 47.51
Then,
log(k*x1+b) = y1/a
k*x1+b = exp(y1/a)
C*k*x1+C*b = C*exp(y1/a)
From this we can calculate C:
C = (C*k*x1+C*b)/exp(y1/a)
and finally find the k
and b
:
k=0.226
b=1.0016
These are the values used above for finding the better fit.
UPDATE
You can automate the process described above with the following script:
# Name of the file with the data
data='R_B.txt'
# The coordinates of the last data point
xn=NaN
yn=NaN
# The temporary coordinates of a data point used to calculate a derivative
x0=NaN
y0=NaN
linearFit(x)=Ck*x+Cb
fit linearFit(x) data using (xn=$1,dx=$1-x0,x0=$1,$1):(yn=$2,dy=$2-y0,y0=$2,dx/dy) via Ck, Cb
# The coordinates of the first data point
x1=NaN
y1=NaN
plot data using (x1=$1):(y1=$2) every ::0::0
a=(yn-y1)/log((Ck*xn+Cb)/(Ck*x1+Cb))
C=(Ck*x1+Cb)/exp(y1/a)
k=Ck/C
b=Cb/C
f(x)=a*log(k*x+b)
fit f(x) data via a,k,b
plot data, f(x)
pause -1