The Fibonacci sphere approximation is quite easy to generalize efficiently to a subset of points computation, as the analytic formulas are very straight-forward.
The below code computes the subset of points shown below for a trillion points in a few seconds of runtime on my weak laptop and a relatively under optimised python implementation.
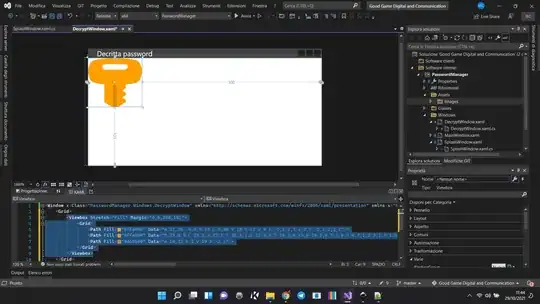
Code to compute the above is below, and includes a means to verify the subset computation is exactly the same as a brute-force computation (however don't try it for trillion points, it will never finish unless you have a super-computer!)
Please note, the use of 128-bit doubles is an absolute requirement when you do the computation over more than about a billion points as there are major quantisation artefacts otherwise!
Runtime scales with r' * N
where r'
is the ratio of the subset to that of the full sphere. Thus, a very small r'
can be computed very efficiently.
#!/usr/bin/env python3
import argparse
import mpl_toolkits.mplot3d.axes3d as ax3d
import matplotlib.pyplot as plt
import numpy as np
def fibonacci_sphere_pts(num_pts):
ga = (3 - np.sqrt(5)) * np.pi # golden angle
# Create a list of golden angle increments along tha range of number of points
theta = ga * np.arange(num_pts)
# Z is a split into a range of -1 to 1 in order to create a unit circle
z = np.linspace(1 / num_pts - 1, 1 - 1 / num_pts, num_pts)
# a list of the radii at each height step of the unit circle
radius = np.sqrt(1 - z * z)
# Determine where xy fall on the sphere, given the azimuthal and polar angles
y = radius * np.sin(theta)
x = radius * np.cos(theta)
return np.asarray(list(zip(x,y,z)))
def fibonacci_sphere(num_pts):
x,y,z = zip(*fibonacci_sphere_subset(num_pts))
# Display points in a scatter plot
fig = plt.figure()
ax = fig.add_subplot(111, projection="3d")
ax.scatter(x, y, z)
plt.show()
def fibonacci_sphere_subset_pts(num_pts, p0, r0 ):
"""
Get a subset of a full fibonacci_sphere
"""
ga = (3 - np.sqrt(5)) * np.pi # golden angle
x0, y0, z0 = p0
z_s = 1 / num_pts - 1
z_e = 1 - 1 / num_pts
# linspace formula for range [z_s,z_e] for N points is
# z_k = z_s + (z_e - z_s) / (N-1) * k , for k [0,N)
# therefore k = (z_k - z_s)*(N-1) / (z_e - z_s)
# would be the closest value of k
k = int(np.round((z0 - z_s) * (num_pts - 1) / (z_e - z_s)))
# here a sufficient number of "layers" of the fibonacci sphere must be
# selected to obtain enough points to be a superset of the subset given the
# radius, we use a heuristic to determine the number but it can be obtained
# exactly by the correct formula instead (by choosing an upperbound)
dz = (z_e - z_s) / (num_pts-1)
n_dk = int(np.ceil( r0 / dz ))
dk = np.arange(k - n_dk, k + n_dk+1)
dk = dk[np.where((dk>=0)&(dk<num_pts))[0]]
# NOTE: *must* use long double over regular doubles below, otherwise there
# are major quantization errors in the output for large number of points
theta = ga * dk.astype(np.longdouble)
z = z_s + (z_e - z_s ) / (num_pts-1) *dk
radius = np.sqrt(1 - z * z)
y = radius * np.sin(theta)
x = radius * np.cos(theta)
idx = np.where(np.square(x - x0) + np.square(y-y0) + np.square(z-z0) <= r0*r0)[0]
return x[idx],y[idx],z[idx]
def fibonacci_sphere_subset(num_pts, p0, r0, do_compare=False ):
"""
Display fib sphere subset points and optionally compare against bruteforce computation
"""
x,y,z = fibonacci_sphere_subset_pts(num_pts,p0,r0)
if do_compare:
subset = zip(x,y,z)
subset_bf = fibonacci_sphere_pts(num_pts)
x0,y0,z0 = p0
subset_bf = [ (x,y,z) for (x,y,z) in subset_bf if np.square(x - x0) + np.square(y-y0) + np.square(z-z0) <= r0*r0 ]
subset_bf = np.asarray(subset_bf)
if np.allclose(subset,subset_bf):
print('PASS: subset and bruteforce computation agree completely')
else:
print('FAIL: subset and bruteforce computation DO NOT agree completely')
# Display points in a scatter plot
fig = plt.figure()
ax = fig.add_subplot(111, projection="3d")
ax.scatter(x, y, z)
plt.show()
if __name__ == "__main__":
parser = argparse.ArgumentParser(description="fibonacci sphere")
parser.add_argument(
"numpts", type=int, help="number of points to distribute along sphere"
)
args = parser.parse_args()
# hard-coded point to query with a tiny fixed radius
p0 = (.5,.5,np.sqrt(1. - .5*.5 - .5*.5)) # coordinate of query point representing center of subset, note all coordinates fall between -1 and 1
r0 = .00001 # the radius of the subset, a very small number is chosen as radius of full sphere is 1.0
fibonacci_sphere_subset(int(args.numpts),p0,r0,do_compare=False)