This is possible. Here is a simple approach:
Flow:
You can perform histogram equalization for a given region with the help of the mask.
- Using the mask, store coordinates where pixels are in white.
- Store pixel intensities from these coordinates present in the grayscale image
- Perform histogram equalization on these stored pixels. You will now get a new set of values.
- Replace the old pixel values with the new ones based on the coordinate positions.
Code:
The following is an illustration using a grayscale image.
img = cv2.imread('flower.jpg')
gray = cv2.cvtColor(img, cv2.COLOR_BGR2GRAY)
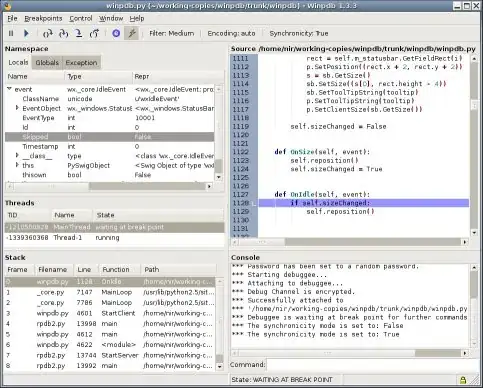
The following is the mask:
mask = cv2.imread('mask.jpg', 0)

I want to perform histogram equalization only on the flower.
Store the coordinates where pixels are white (255) in mask
:
coord = np.where(mask == 255)
Store all pixel intensities on these coordinates present in gray
:
pixels = gray[coord]
Perform histogram equalization on these pixel intensities:
equalized_pixels = cv2.equalizeHist(pixels)
Create a copy of gray
named gray2
. Place the equalized intensities in the same coordinates:
gray2 = gray.copy()
for i, C in enumerate(zip(coord[0], coord[1])):
gray2[C[0], C[1]] = equalized_pixels[i][0]
cv2.imshow('Selective equalization', gray2)

Comparison:
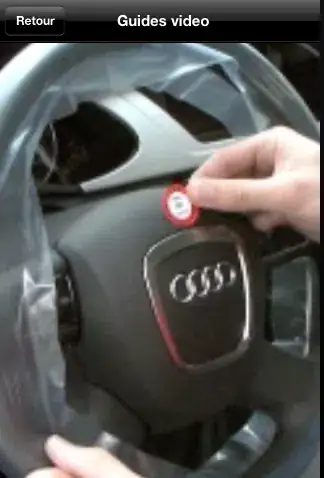
Note: This process can be extended for Histogram Equalization or CLAHE, ON RGB or grayscale images.