It's not really clear what you're going for, so I'll provide a couple of demonstrations. Up front, I'm assuming that you have two distinct datasets here, first in columns 1-2, second in columns 3-4. This can be done literally in ggplot2
with:
library(ggplot2)
ggplot(dat) +
geom_line(aes(distance, grey1), color="red") +
geom_line(aes(distance1, grey2), color="blue")
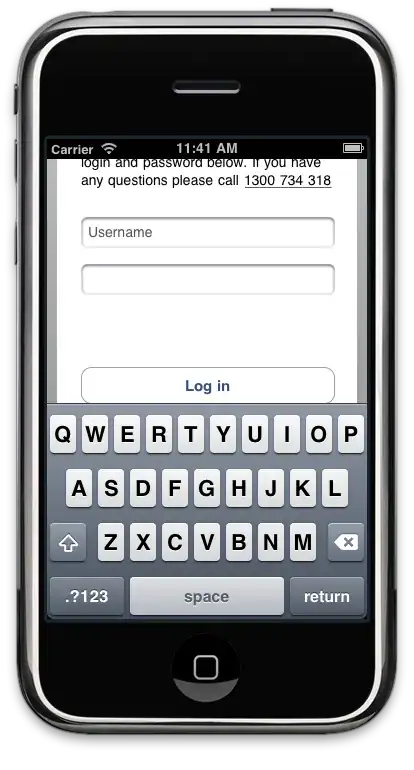
But this approach is brute-forcing it a bit, and will make things like legends, color-control, etc, rather painful. I suggest this process would benefit from reshaping the data into a long-format, with just the x and y variables plus one to indicate the group from which that row came. For example,
library(data.table)
newdat <- data.table::melt(as.data.table(dat),
measure = patterns("^distance","^grey"),
value.name = c("distance", "grey"))
newdat
# variable distance grey
# <fctr> <num> <num>
# 1: 1 0.0000000 -300.364
# 2: 1 0.2174741 -296.963
# 3: 1 0.4349482 -292.887
# 4: 1 0.6520882 -290.310
# 5: 1 0.8695623 -285.777
# 6: 1 1.0870364 -279.921
# 7: 1 1.3045105 -274.418
# 8: 1 1.5216505 -272.005
# 9: 1 1.7391246 -273.666
# 10: 1 1.9565987 -270.381
# ---
# 15: 2 0.4229937 -131.959
# 16: 2 0.6341657 -133.514
# 17: 2 0.8456625 -127.111
# 18: 2 1.0571594 -116.404
# 19: 2 1.2686562 -116.850
# 20: 2 1.4798282 -115.464
# 21: 2 1.6913251 -102.823
# 22: 2 1.9028219 -101.497
# 23: 2 2.1143188 -98.245
# 24: 2 2.3254907 -98.474
Where the new variable
column indicates from which column-group the data came from.
Here, the plotting in ggplot becomes a bit simpler:
ggplot(newdat, aes(distance, grey)) +
geom_line(aes(color = variable, group = variable))
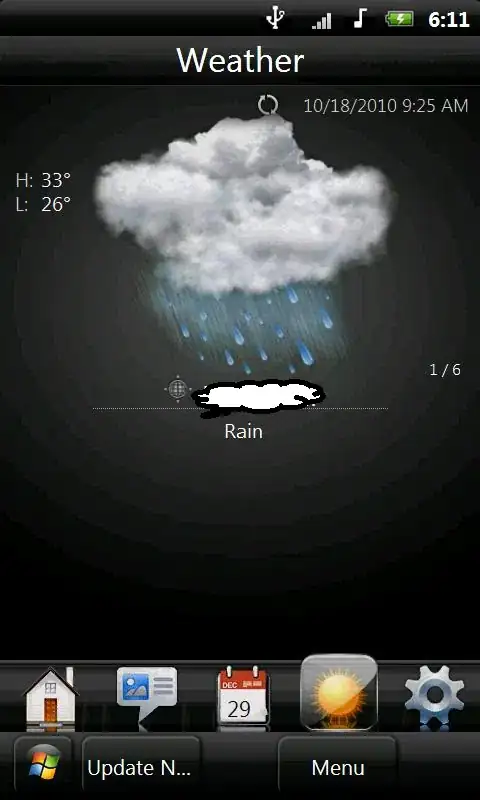
Notice that we now have a legend, and it is handling colors itself. These can be overridden, but that's a different topic (and addressed in numerous questions here on SO).
As for "average plot of the 2 samples", this will take a bit more context into the data, and is currently not well-enough informed. My biggest concern is that neither distance
nor grey
for each group of data is perfectly aligned. That is, if distance
had a value of exactly 1.000
in both, then I think we can safely average the grey
values of those two observations. However, this is not the case in general (nor anywhere in this sample dataset).
If you really want to find a form of average, I suggest you interpolate both lines onto a known domain of distance
and show the average. I'll demo what I mean.
First, I'll add points so that we can see the x-wise misalignment:
ggplot(newdat, aes(distance, grey, color = variable)) +
geom_line() +
geom_point()
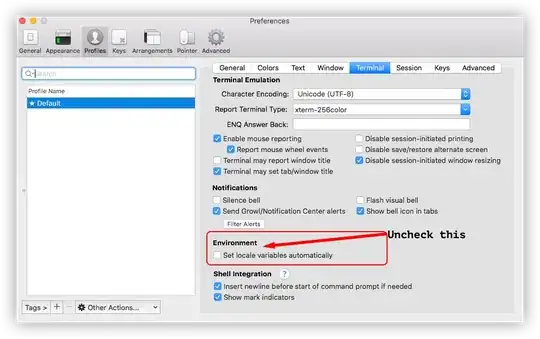
Now, let's aggregate the "average" (from interpolated distance
and add them to the original long-form data.
newdist <- seq(0, min(max(dat$distance), max(dat$distance1)), by = 0.1)
newdat2 <- newdat[, setNames(approx(distance, grey, xout = newdist), c("distance", "grey")), by = variable
][, .(variable = "Avg", grey = mean(grey)), by = distance]
newdat2 <- rbindlist(list(newdat, newdat2), use.names = TRUE)
Now, we can use the same plot command and get the third line:
ggplot(newdat2, aes(distance, grey, color = variable)) +
geom_line() +
geom_point()
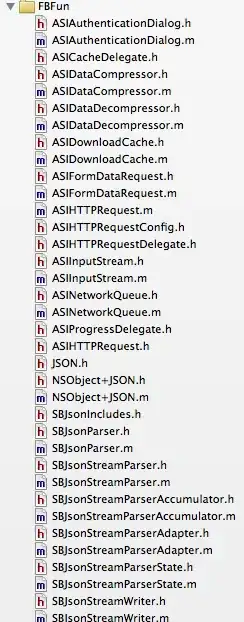
This method is making some inferences on the data, something we don't have much of here in the question. I think this is a safe step, but make sure it makes sense statistically before blindly using this technique on your data.
Data (I started writing this before the columns were renamed, so follow-on code may need to be adjusted).
dat <- structure(list(distance = c(0, 0.2174741, 0.4349482, 0.6520882, 0.8695623, 1.0870364, 1.3045105, 1.5216505, 1.7391246, 1.9565987, 2.1740728, 2.3912128), grey1 = c(-300.364, -296.963, -292.887, -290.31, -285.777, -279.921, -274.418, -272.005, -273.666, -270.381, -270.273, -270.705), distance1 = c(0, 0.2114969, 0.4229937, 0.6341657, 0.8456625, 1.0571594, 1.2686562, 1.4798282, 1.6913251, 1.9028219, 2.1143188, 2.3254907), grey2 = c(-135.219, -132.601, -131.959, -133.514, -127.111, -116.404, -116.85, -115.464, -102.823, -101.497, -98.245, -98.474)), class = "data.frame", row.names = c("1", "2", "3", "4", "5", "6", "7", "8", "9", "10", "11", "12"))