It's possible to perform loess smoothing within ggplot.
library(data.table)
library(ggplot2)
df <- economics
##
#
gg.melt <- setDT(df) |> melt(id='date', variable.name = 'KPI')
ggplot(gg.melt, aes(x=date, y=value))+
geom_line()+
stat_smooth(method=loess, color='red', size=0.5, se=FALSE, method.args = list(span=0.1))+
facet_wrap(~KPI, scales = 'free_y')

Regarding combining everything on one plot I'm not seeing how you would do that as the y-scales are so different. If the point is to see how the peaks line up, etc. you could do this:
ggplot(gg.melt, aes(x=date, y=value))+
geom_line()+
stat_smooth(method=loess, color='red', size=0.5, se=FALSE, method.args = list(span=0.1))+
facet_grid(KPI~., scales = 'free_y')
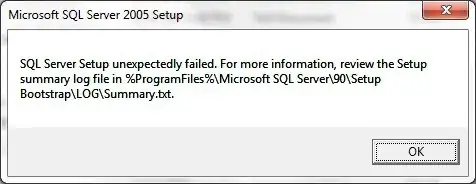
There is also the dygraphs
package which allows creation of dynamic graphics that can be saved to html:
gg.melt[, scaled:=scale(value, center = FALSE, scale=diff(range(value))), by=.(KPI)]
gg.melt[, pred:=predict(loess(scaled~as.integer(date), .SD, span=0.1)), by=.(KPI)]
gg.dt <- dcast(gg.melt, date~KPI, value.var = list('scaled', 'pred'))
library(dygraphs)
dygraph(gg.dt) |>
dyCrosshair(direction = 'vertical') |>
dyRangeSelector()

It's possible to create a dygraph(...)
version of the second plot, where the different KPI are in different facets, but you have to use RMarkdown for that.