Using GNU Parallel
parallel --jobs 4 python execute_function.py ::: files*
By default it will run one job per cpu-core. This can be adjusted with --jobs
.
GNU Parallel is a general parallelizer and makes is easy to run jobs in parallel on the same machine or on multiple machines you have ssh access to.
If you have 32 different jobs you want to run on 4 CPUs, a straight forward way to parallelize is to run 8 jobs on each CPU:
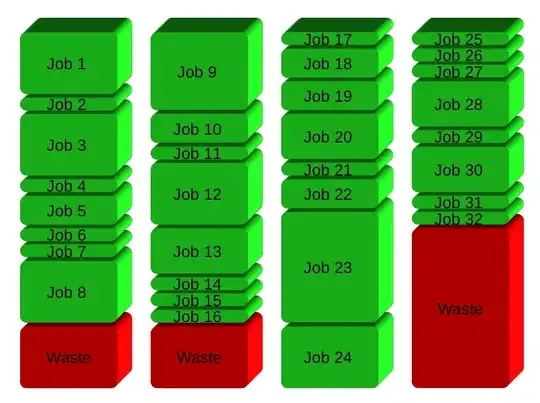
GNU Parallel instead spawns a new process when one finishes - keeping the CPUs active and thus saving time:

Installation
For security reasons you should install GNU Parallel with your package manager, but if GNU Parallel is not packaged for your distribution, you can do a personal installation, which does not require root access. It can be done in 10 seconds by doing this:
$ (wget -O - pi.dk/3 || lynx -source pi.dk/3 || curl pi.dk/3/ || \
fetch -o - http://pi.dk/3 ) > install.sh
$ sha1sum install.sh | grep 883c667e01eed62f975ad28b6d50e22a
12345678 883c667e 01eed62f 975ad28b 6d50e22a
$ md5sum install.sh | grep cc21b4c943fd03e93ae1ae49e28573c0
cc21b4c9 43fd03e9 3ae1ae49 e28573c0
$ sha512sum install.sh | grep da012ec113b49a54e705f86d51e784ebced224fdf
79945d9d 250b42a4 2067bb00 99da012e c113b49a 54e705f8 6d51e784 ebced224
fdff3f52 ca588d64 e75f6033 61bd543f d631f592 2f87ceb2 ab034149 6df84a35
$ bash install.sh
For other installation options see http://git.savannah.gnu.org/cgit/parallel.git/tree/README