Both your first and second loops are O(n)
, so together they are O(n^2)
.
Inside your loop, neither the max or multiplication depend on the number of array elements. For languages like C, C++, C#, Java, etc., getting a value from an array does not add time complexity as n increases, so we say that is O(1)
.
While the multiplication and max will take time, they are also O(1)
, because they will always run at a constant time, regardless of n. (I will note that the values here will get extremely large for arrays containing values >1 because of the max, so I'm guessing some languages might start to slow down past a certain value, but that's just speculation.)
All in all, you have
O(n):
O(n):
O(1)
So the total is O(n^2)
Verification:
As a reminder, while time complexity analysis is a vital tool, you can always measure it. I did this in C# and measured both the time and number of inner loop executions.
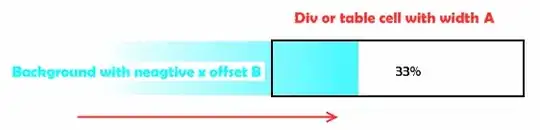
That trendline is just barely under executions = 0.5n^2
. Which makes sense if you think about low numbers of n. You can step through your loops on a piece of paper and immediately see the pattern.
n=5
: 5 outer loop iterations, 10 total inner loop iterations
n=10
10 outer loop iterations, 45 total inner loop iterations
n=20
20 outer loop iterations, 190 total inner loop iterations
For timing, we see an almost identical trend with just a different constant. This indicates that our time, T(n), is directly proportional to the number of inner loop iterations.
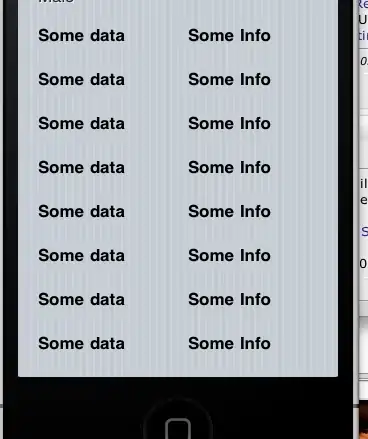
Takeaway:
The analysis which gave O(n^2) worked perfectly. The statements within the loop were O(1) as expected. The check didn't end up being necessary, but it's useful to see just how closely the analysis and verification match.