Given a 2D array of points the area covered by the points can be calculated with help of the return value of the same hist2d()
function provided in the matplotlib module (as matplotlib.pyplot.hist2d()
) which is used to show the scatterplot.
The 'trick' is to set the cmin
parameter value of the function to 1 ( cmin=1
) and then calculate the number of numpy.nan
values in the by the function returned array setting them in relation to entire amount of array values.
In other words all what is necessary to calculate the area when creating the scatterplot is already there for easy use in a simple area calculation formulas if you know that the histogram creating function provide as return value all what is therefore necessary.
Below code of a ready to use function for the area calculation along with demonstration of function usage:
def area_of_points(points, grid_size = [1000, 1000]):
"""
Returns the area covered by N 2D-points provided in a 'points' array
points = [ (x1,y1), (x2,y2), ... , (xN, yN) ]
'grid_size' gives the number of grid cells in x and y direction the
'points' bounding box is divided into for calculation of the area.
Larger 'grid_size' values mean smaller grid cells, higher precision
of the area calculation and longer runtime.
area_of_points() requires installed matplotlib module. """
import matplotlib.pyplot as plt
import numpy as np
pts_x = [x for x,y in points]
pts_y = [y for x,y in points]
pts_bb_area = (max(pts_x)-min(pts_x))*(max(pts_y)-min(pts_y))
h2D,_,_,_ = plt.hist2d( pts_x, pts_y, bins = grid_size, cmin=1)
numberOfWhiteBins = np.count_nonzero(np.isnan(h2D))
numberOfAll2Dbins = h2D.shape[0]*h2D.shape[1]
areaFactor = 1.0 - numberOfWhiteBins/numberOfAll2Dbins
pts_pts_area = areaFactor * pts_bb_area
print(f'Areas: b-box = {pts_bb_area:8.4f}, points = {pts_pts_area:8.4f}')
plt.show()
return pts_pts_area
#:def area_of_points(points, grid_size = [1000, 1000])
import numpy as np
np.random.seed(12345)
x = np.random.normal(size=100000)
y = x + np.random.normal(size=100000)
pts = [[xi,yi] for xi,yi in zip(x,y)]
print(area_of_points(pts))
# ^-- prints: Areas: b-box = 114.5797, points = 7.8001
# ^-- prints: 7.800126875291629
The above code creates following scatterplot:

Notice that the printed output Areas: b-box = 114.5797, points = 7.8001
and the by the function returned area value 7.800126875291629
give the area in units in which the x,y coordinates in the array of points are specified.
Instead of usage of a function when utilizing the know how you can play around with the parameter of the scatterplot calculating the area of what can be seen in the scatterplot.
Below code which changes the displayed scatterplot using the same underlying point data:
import numpy as np
np.random.seed(12345)
x = np.random.normal(size=100000)
y = x + np.random.normal(size=100000)
pts = [[xi,yi] for xi,yi in zip(x,y)]
pts_values_example = \
[[0.53005, 2.79209],
[0.73751, 0.18978],
... ,
[-0.6633, -2.0404],
[1.51470, 0.86644]]
# ---
pts_x = [x for x,y in pts]
pts_y = [y for x,y in pts]
pts_bb_area = (max(pts_x)-min(pts_x))*(max(pts_y)-min(pts_y))
# ---
import matplotlib.pyplot as plt
bins = [320, 300] # resolution of the grid (for the scatter plot)
# ^-- resolution of precision for the calculation of area
pltRetVal = plt.hist2d( pts_x, pts_y, bins = bins, cmin=1, cmax=15 )
plt.colorbar() # display the colorbar (for a 2d density histogram)
plt.show()
# ---
h2D, xedges1D, yedges1D, h2DhistogramObject = pltRetVal
numberOfWhiteBins = np.count_nonzero(np.isnan(h2D))
numberOfAll2Dbins = (len(xedges1D)-1)*(len(yedges1D)-1)
areaFactor = 1.0 - numberOfWhiteBins/numberOfAll2Dbins
area = areaFactor * pts_bb_area
print(f'Areas: b-box = {pts_bb_area:8.4f}, points = {area:8.4f}')
# prints "Areas: b-box = 114.5797, points = 20.7174"
creating following scatterplot:
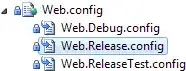
Notice that the calculated area is now larger due to smaller values used for grid resolution resulting in more of the area colored.