tl;dr
My answer is
view = np.lib.stride_tricks.sliding_window_view(tmp_, (win_,))
xxx=np.vstack([np.arange(win_), np.ones(win_)]).T
roll_mat=(np.linalg.inv(xxx.T @ xxx) @ (xxx.T) @ view.T)[0]
And it takes 1.2 ms to compute, compared to 2 seconds for your pandas and numpy version, and 3.5 seconds for your stat version.
Long version
One method could be to use sliding_window_view
to transform your tmp_
array, into an array of window (a fake one: it is just a view, not really a 10000x30 array of data. It is just tmp_
but viewed differenty. Hence the _view
in the function name).
No direct advantage. But then, from there, you can try to take advantage of vectorization.
I do that two different way: an easy one, and one that takes a minute of thinking. Since I put the best answer first, the rest of this message can appear inconsistent chronologically (I say things like "in my previous answer" when the previous answer come later), but I tried to redact both answer consistently.
New answer : matrix operations
One method to do that (since lstsq
is of the rare numpy method that wouldn't just do it naturally) is to go back to what lstsq(X,Y)
does in reality: it computes (XᵀX)⁻¹Xᵀ Y
So let's just do that. In python, with xxx
being the X array (of arange and 1 in your example) and view
the array of windows to your data (that is view[i]
is tmp_[i:i+win_]
), that would be np.linalg.inv(xxx.T@xxx)@xxx.T@view[i]
for i being each row. We could vectorize that operation with np.vectorize
to avoid iterating i
, as I did for my first solution (see below). But the thing is, we don't need to. That is just a matrix times a vector. And the operation computing a matrix times a vector for each vector in an array of vectors, is just matrix multiplication!
Hence my 2nd (and probably final) answer
view = np.lib.stride_tricks.sliding_window_view(tmp_, (win_,))
xxx=np.vstack([np.arange(win_), np.ones(win_)]).T
roll_mat=(np.linalg.inv(xxx.T @ xxx) @ (xxx.T) @ view.T)[0]
roll_mat
is still identical (with one extra row because your roll_np stopped one row short of the last possible one) to roll_np
(see below for graphical proof with my first answer. I could provide a new image for this one, but it is indistinguishable from the one I already used). So same result (unsurprisingly I should say... but sometimes it is still a surprise when things work exactly like theory says they do)
But timing, is something else. As promised, my previous factor 4 was nothing compared to what real vectorization can do. See updated timing table:
Method |
Time |
pandas |
2.10 s |
numpy roll |
2.03 s |
stat |
3.58 s |
numpy view/vectorize (see below) |
0.46 s |
numpy view/matmult |
1.2 ms |
The important part is 'ms', compared to other 's'.
So, this time factor is 1700 !
Old-answer : vectorize
A lame method, once we have this view
could be to use np.vectorize
from there. I call it lame
because vectorize
is not supposed to be efficient. It is just a for loop called by another name. Official documentation clearly says "not to be used for performance". And yet, it would be an improvement from your code
view = np.lib.stride_tricks.sliding_window_view(tmp_, (win_,))
xxx=np.vstack([np.arange(win_), np.ones(win_)]).T
f = np.vectorize(lambda y: np.linalg.lstsq(xxx,y,rcond=None)[0][0], signature='(n)->()')
roll_vectorize=f(view)
Firt let's verify the result
plt.scatter(f(view)[:-1], roll_np))
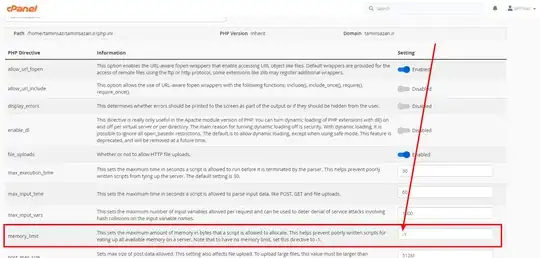
So, obviously, same results as roll_np
(which, I've checked the same way, are the same results as the two others. With also the same variation on indexing since all 3 methods have not the same strategy for border)
And the interesting part, timings:
Method |
Time |
pandas |
2.10 s |
numpy roll |
2.03 s |
stat |
3.58 s |
numpy view/vectorize |
0.46 s |
So, you see, it is not supposed to be for performance, and yet, I gain more that x4 times with it.
I am pretty sure that a more vectorized method (alas, lstsq doesn't allow directly it, unlike most numpy functions) would be even faster.