This answer completes the good answer of @user2357112 by providing additional details. Both functions are optimized. That being said the pair-wise summation is generally a bit slower while providing generally a more accurate result. It is also sub-optimal yet though relatively good. OpenBLAS which is used by default on Windows does not perform a pair-wise summation.
Here is the assembly code for the Numpy code:
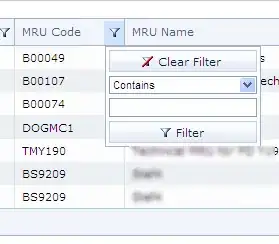
Here is the assembly code for the OpenBLAS code:
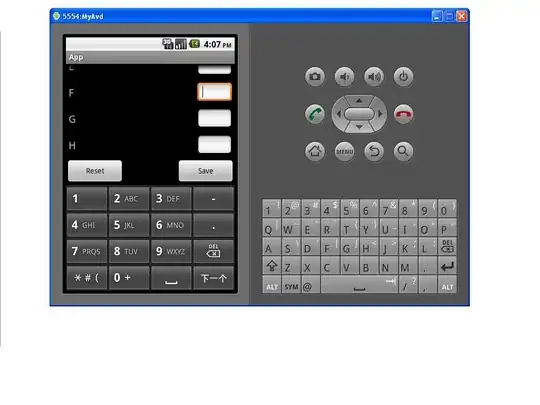
The main issue with the Numpy code is that it does not use AVX (256-bit SIMD instruction set) but SSE (128-bit SIMD instruction set) as opposed to OpenBLAS, at least at the version 1.22.4 (the one I use) and before. Even worst: the instructions are scalar one in the Numpy code! We recently worked on this and the last version of Numpy should now use AVX. That being said, it may still be not as fast as OpenBLAS because of the pair-wise summation (especially for big arrays).
Note that both functions spent a non-negligible time in overheads because of the arrays being too small. Such overheads can be removed using a hand-written implementation in Numba.
The order of the timings reverses if the array is much longer.
This is expected. Indeed, functions are rather compute-bound when they operate in the cache, but they become memory-bound when the array is big and fit in the L3 cache or even the RAM. As a result, np.dot
stats to be slower for bigger array since it needs to read twice bigger data from memory. More specifically, it needs to read 8*1000000*2/1024**2 ~= 15.3 MiB
from memory so you likely need to read data from your RAM which have a pretty limited throughout. In fact, a good bi-channel 3200 MHz DDR4 RAM like mine can reach a practical throughput close to 40 GiB and 15.3/(40*1024) ~= 374 µs
. That being said, sequential codes can hardly completely saturate this throughput so reaching a 30 GiB/s in sequential is already great not to mention many mainstream PC RAM operate at a lower frequency. A 30 GHz/s throughput result in ~500 µs which is close to your timings. Meanwhile, np.sum
and np.add.reduce
are more compute-bound because of their inefficient implementation, but the amount of data to be read is twice smaller and may actually fit better in the L3 cache having a significantly bigger throughput.
To prove this effect, you can simply try to run:
# L3 cache of 9 MiB
# 2 x 22.9 = 45.8 MiB
a = np.ones(3_000_000)
b = np.ones(3_000_000)
%timeit -n 100 np.dot(a, a) # 494 µs => read from RAM
%timeit -n 100 np.dot(a, b) # 1007 µs => read from RAM
# 2 x 7.6 = 15.2 MiB
a = np.ones(1_000_000)
b = np.ones(1_000_000)
%timeit -n 100 np.dot(a, a) # 90 µs => read from the L3 cache
%timeit -n 100 np.dot(a, b) # 283 µs => read from RAM
# 2 x 1.9 = 3.8 MiB
a = np.ones(250_000)
b = np.ones(250_000)
%timeit -n 100 np.dot(a, a) # 40 µs => read from the L3 cache (quite compute-bound)
%timeit -n 100 np.dot(a, b) # 46 µs => read from the L3 cache too (quite memory-bound)
On my machine, the L3 has only a size of 9 MiB so the second call needs not only to read twice more data but also read it more from the slower RAM than from the L3 cache.
For small array, the L1 cache is so fast that reading data should not be a bottleneck. On my i5-9600KF machine, the throughput of the L1 cache is huge : ~268 GiB/s. This means the optimal time to read two arrays of size 1000 is 8*1000*2/(268*1024**3) ~= 0.056 µs
. In practice, the overhead of calling a Numpy function is much bigger than that.
Fast implementation
Here is a fast Numba implementation:
import numba as nb
# Function eagerly compiled only for 64-bit contiguous arrays
@nb.njit('float64(float64[::1],)', fastmath=True)
def fast_sum(arr):
s = 0.0
for i in range(arr.size):
s += arr[i]
return s
Here are performance results:
array items | time | speedup (dot/numba_seq)
--------------------------|------------------------
3_000_000 | 870 µs | x0.57
1_000_000 | 183 µs | x0.49
250_000 | 29 µs | x1.38
If you use the flag parallel=True
and nb.prange
instead of range
, Numba will use multiple threads. This is good for large arrays, but it may not be for small ones on some machine (due to the overhead to create threads and share the work):
array items | time | speedup (dot/numba_par)
--------------------------|--------------------------
3_000_000 | 465 µs | x1.06
1_000_000 | 66 µs | x1.36
250_000 | 10 µs | x4.00
As expected, Numba can be faster for small array (because the Numpy call overhead is mostly removed) and be competitive with OpenBLAS for large array. The code generated by Numba is pretty efficient:
.LBB0_7:
vaddpd (%r9,%rdx,8), %ymm0, %ymm0
vaddpd 32(%r9,%rdx,8), %ymm1, %ymm1
vaddpd 64(%r9,%rdx,8), %ymm2, %ymm2
vaddpd 96(%r9,%rdx,8), %ymm3, %ymm3
vaddpd 128(%r9,%rdx,8), %ymm0, %ymm0
vaddpd 160(%r9,%rdx,8), %ymm1, %ymm1
vaddpd 192(%r9,%rdx,8), %ymm2, %ymm2
vaddpd 224(%r9,%rdx,8), %ymm3, %ymm3
vaddpd 256(%r9,%rdx,8), %ymm0, %ymm0
vaddpd 288(%r9,%rdx,8), %ymm1, %ymm1
vaddpd 320(%r9,%rdx,8), %ymm2, %ymm2
vaddpd 352(%r9,%rdx,8), %ymm3, %ymm3
vaddpd 384(%r9,%rdx,8), %ymm0, %ymm0
vaddpd 416(%r9,%rdx,8), %ymm1, %ymm1
vaddpd 448(%r9,%rdx,8), %ymm2, %ymm2
vaddpd 480(%r9,%rdx,8), %ymm3, %ymm3
addq $64, %rdx
addq $-4, %r11
jne .LBB0_7
That being said, it is not optimal : the LLVM-Lite JIT compiler uses a 4x unrolling while a 8x unrolling should be optimal on my Intel CoffeeLake processor. Indeed, the latency of the vaddpd
instruction is 4 cycle while 2 instruction can be executed per cycle so 8 registers are needed to avoid a stall and the resulting code being latency bound. Besides, this assembly code is optimal on Intel Alderlake and Sapphire Rapids processors since they have a twice lower vaddpd
latency. Saturating FMA SIMD processing units is far from being easy. I think the only way to write a faster function is to write a (C/C++) native code using SIMD intrinsics though it is less portable.
Note the Numba code does not support special numbers like NaN or Inf value because of fastmath
(AFAIK OpenBLAS does). In practice, it should still work on x86-64 machines but this is not guaranteed. Besides, the Numba code is not numerically stable for very large arrays. The Numpy code should be the most numerically stable of the three variants (then the OpenBLAS code). You can compute the sum by chunk to improve the numerical stability though it makes the code more complex. There is not free lunch.