What you are trying to achieve reminded me of a textbook example of a noise-free Gaussian Process (GP) Regression. GP is an advance machine learning topic that could be very useful for your use case. Here is a terrific interactive tool for understanding GPs.
As explained there
They allow us to make predictions about our data by incorporating prior knowledge. Their most obvious area of application is fitting a function to the data. This is called regression and is used, for example, in robotics or time series forecasting. But Gaussian processes are not limited to regression — they can also be extended to classification and clustering tasks. For a given set of training points, there are potentially infinitely many functions that fit the data. Gaussian processes offer an elegant solution to this problem by assigning a probability to each of these functions. The mean of this probability distribution then represents the most probable characterization of the data. Furthermore, using a probabilistic approach allows us to incorporate the confidence of the prediction into the regression result.
Let's see how you can use GP regression to accomplish what you want using the scikit-learn
library.
The code below is made of three functions:
train_gaussian_process
: your observations x
and y
are used to train the GP.
generate_samples_from_gp
: from the GP we've just trained, we sample 5 functions (in your case it will be 5000). The function values are stored in a 2D numpy array, where the i-th column represents the i-th function.
draw_samples
: draw the observations and the sampled functions.
A critical component of a GP is the covariance function. You asked for smooth functions and you want to avoid oscillatory behavior, so the RBF kernel of the Matern one could be the right choice. The code below uses the Matern kernel. Play around with their parameters to get what you want.
import numpy as np
from matplotlib import pyplot as plt
from sklearn.gaussian_process import GaussianProcessRegressor
from sklearn.gaussian_process.kernels import Matern
rng = np.random.RandomState(42)
def main() -> None:
x = [7.81, 21.65, 186.29, 246.62, 402.74, 446.24, 572.99, 585.11, 613.57, 762.97]
y = [26.27, 26.41, 26.46, 27.36, 34.32, 34.50, 37.94, 39.05, 39.23, 39.88]
x = np.array(x).reshape((-1, 1))
y = np.array(y).reshape(-1, 1)
z = data_generating_process(x, y)
sample_size = 5 # replace with 5_000
gp_regressor = train_gaussian_process(x, y)
gp_regressor_3d = train_gaussian_process(np.column_stack((x, y)), z)
offset = 5.0
additional_x = np.linspace(min(x) - offset, max(x) + offset, 1000).reshape((-1, 1))
x_ = np.linspace(min(x) - offset, max(x) + offset, 50)
y_ = np.linspace(min(y) - offset, max(y) + offset, 50)
additional_xy = np.array([[_x, _y] for _x in x_ for _y in y_]).squeeze(-1)
samples = generate_samples_from_gp(gp_regressor, additional_x, sample_size)
samples_3d = generate_samples_from_gp(gp_regressor_3d, additional_xy, sample_size)
draw_samples(x, y, samples, additional_x)
draw_samples_3d(x, y, z, samples_3d, additional_xy)
def data_generating_process(x: np.ndarray, y: np.ndarray) -> np.ndarray:
"""
Generate points from a test function.
Parameters
----------
x : np.ndarray
y : np.ndarray
Returns
-------
np.ndarray
"""
return y + np.log(x)
def train_gaussian_process(x: np.ndarray, y: np.ndarray) -> GaussianProcessRegressor:
"""
Train Gaussian Process.
Parameters
----------
x : np.ndarray
y : np.ndarray
Returns
-------
GaussianProcessRegressor
"""
kernel = Matern(length_scale=4.0, nu=2.5)
gaussian_process = GaussianProcessRegressor(kernel=kernel, n_restarts_optimizer=9)
gaussian_process.fit(x, y)
return gaussian_process
def generate_samples_from_gp(
gaussian_process: GaussianProcessRegressor, points: np.ndarray, sample_size: int
) -> np.ndarray:
"""
Sample functions from Gaussian Process.
Parameters
----------
gaussian_process : np.ndarray
points : np.ndarray
sample_size : int
Returns
-------
np.ndarray
"""
return gaussian_process.sample_y(points, n_samples=sample_size)
def draw_samples(
x: np.ndarray, y: np.ndarray, samples: np.ndarray, points: np.ndarray
) -> None:
"""
Draw samples.
Parameters
----------
x : np.ndarray
y : np.ndarray
samples : np.ndarray
points : np.ndarray
"""
plt.figure(figsize=(14, 3))
plt.scatter(x, y, label="Observations")
for i in range(samples.shape[1]):
plt.plot(points, samples[:, i], linewidth=1.2)
plt.legend()
plt.show()
def draw_samples_3d(
x: np.ndarray, y: np.ndarray, z: np.ndarray, samples: np.ndarray, points: np.ndarray
) -> None:
"""
Draw samples.
Parameters
----------
x : np.ndarray
y : np.ndarray
z : np.ndarray
samples : np.ndarray
points : np.ndarray
"""
fig = plt.figure(figsize=(17, 4))
n = int(np.sqrt(points.shape[0]))
x_grid = points[:, 0].reshape((n, n))
y_grid = points[:, 1].reshape((n, n))
for i in range(samples.shape[1]):
axis = fig.add_subplot(1, 5, i + 1, projection="3d")
axis.scatter(x, y, z, label="Observations", s=100, alpha=1)
z_grid = samples[:, i].reshape((n, n))
axis.plot_surface(x_grid, y_grid, z_grid, cmap=plt.cm.plasma)
axis.set_xlabel("x-axis")
axis.set_ylabel("y-axis")
axis.set_zlabel("z-axis")
axis.set_title(f"Sample {i + 1}")
plt.legend()
plt.tight_layout()
plt.show()
if __name__ == "__main__":
main()
As you can see from the image below, all the functions will pass through your observations.
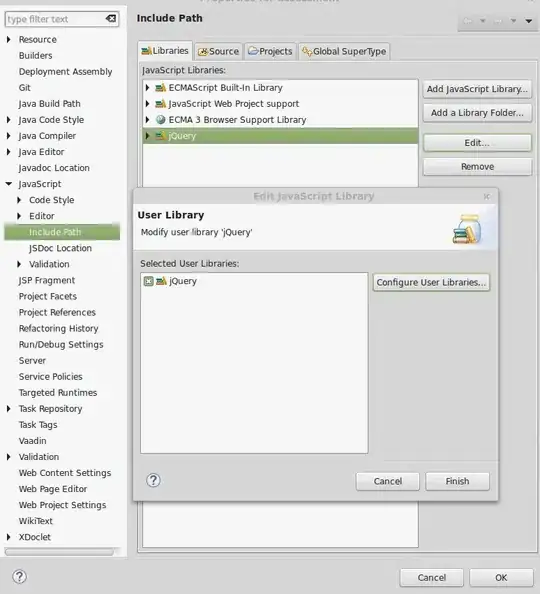
You can also get the mean function by typing:
mean_prediction = gp_regressor.predict(additional_x)
Here is the resulting plot with 5000 functions!
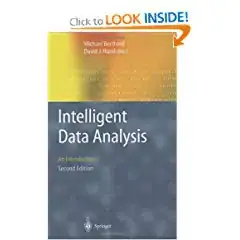
The code above is general so it's ready to be used for a n-dimensional use case. For instance, below 5 sample for a 3D case (I have suppose that the data generating process is x + ln(y)
, but you can choose whatever you want, I needed this equation to get the z
values for the training step).
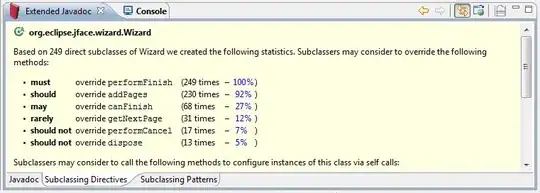