Case of data sorted by month
If I understand you correctly, you're trying to do something like this:
import pandas as pd
data = {
"id": [123456]*16,
"month": range(1,17),
"transactions": [0, 0, 2, 0, 3, 1, 4, 0, 6, 5, 7, 5, 0, 2, 3, 7],
"label": [1, 1, 0, 1, 1, 0, 0, 0, 1, 0, 0, 1, 0, 0, 0, 0]
}
df = pd.DataFrame(data=data)
def collect_transactions(df):
groups = df.groupby(df['label'].cumsum().shift(fill_value=0))
res = groups.agg({
'month': ['first', 'last']
, 'transactions': 'sum'
})
res[('month','first')] -= 1
res['status'] = 1
res.loc[len(res)-1, 'status'] = df['label'].iloc[-1]
return res
# collect data for all users
all_transactions = df.groupby('id').apply(collect_transactions)
# see how it goes for each user separately
for user_id, user_group in df.groupby('id'):
display(collect_transactions(user_group).style.set_caption(f'ID: {user_id}'))
Let's see on the data and code in details. I assume that:
- data are sorted by
month
df['label']
marks the right edges of the month intervals
- the left edges of the intervals are open
df['transactions']
are summarized over the intervals
So we have to split data in groups by these intervals. After that, we take the sum of transactions as well as the first and last items to mark interval edges on each group.
# when marking interval with cumulative sum we have to shift result
# in order to include records with label == 1
# in a previous marked group as its right edge
intervals = df['label'].cumsum().shift(fill_value=0)
groups = df.groupby(intervals)
# when aggregating over a groups we can pass as an argument
# a dictionary with columns of the original data as keys,
# where values are a function or a list of functions
# to run on a corresponding column
res = groups.agg({
'month': ['first', 'last']
, 'transactions': 'sum'
})
# correct the first item in a group
# to make it an open left edge of an interval
res[('month','first')] -= 1
# mark if the last interval has any labels equal 1
# which happens only if in the last record we have label == 1
# so we just copy the last label value at the end of status
res['status'] = 1
res.loc[len(res)-1, 'status'] = df['label'].iloc[-1]
What if data are not sorted by month?
If data are not sorted by month, then we can either sort them before these operations, or use pd.cut(df.month, ...)
to create bins to group by. Let's see if the latter option can be useful.
# make sure we can use labels in `.loc[labels, ...]` operations
# (skip this if labels are boolean)
labels = df.label.astype(bool)
# need inf to cover the last moth;
# later we can use it instead status to see
# if the labels ended by 1 (see the example below)
inf = float('inf')
# create a sequence of the month right edges to cut data in bins;
# include 0 and inf to cover first and last months
month_bins = sorted([0, inf, *df.loc[labels, 'month']])
grouper = pd.cut(df['month'], month_bins)
# when we group by intervals, they automatically are used
# as index of groups, and the output of aggregation is sorted by index,
# so there's no need in the start and end points
res = (
df['transactions']
.groupby(grouper)
.sum(min_count=1) # preserve nan in the presence of (max(df.month), inf]
.dropna() # remove (max(df.month), inf] if exists
.rename('transactions')
)
Let's say we have the data:
data = {
"id": [123456]*16,
"month": range(16,0,-1),
'transactions': reversed([0, 0, 2, 0, 3, 1, 4, 0, 6, 5, 7, 5, 0, 2, 3, 7]),
"label": reversed([1, 1, 0, 1, 1, 0, 0, 0, 1, 0, 0, 1, 0, 0, 0, 0])
}
df = pd.DataFrame(data=data)
On them we gonna have the output:
month
(0.0, 1.0] 0
(1.0, 2.0] 0
(2.0, 4.0] 2
(4.0, 5.0] 3
(5.0, 9.0] 11
(9.0, 12.0] 17
(12.0, inf] 12
Name: transactions, dtype: int64
Here (..., inf]
is the same as status == 0
and (..., max(df.month)]
as status == 1
.
General case
The assumption here is that months by user are not duplicated.
def collect_transactions(df):
month_bins = sorted([0, float('inf'), *df.loc[df.label, 'month']])
grouper = pd.cut(df['month'], month_bins)
res = (
df['transactions']
.groupby(grouper)
.sum(min_count=1)
.dropna()
.rename('transactions')
)
return res.to_frame()
df['label'] = df['label'].astype(bool)
user_groups = df.groupby('id')
# collect the data for all user id
# returned data have multilevel index
# with level_0 - user id, level_1 - month interval
all_transactions = user_groups.apply(collect_transactions)
# for demonstration purposes only
# let's see each group separately
for user_id, user_group in user_groups:
display(
collect_transactions(user_group)
.style
.set_caption(f'ID: {user_id}')
.format(precision=0)
)
On the following test case:
data = {
"id": [123]*16 + [456]*17
, "month": [*range(16,0,-1), *range(1,18)]
, "transactions": [*reversed([0, 0, 2, 0, 3, 1, 4, 0, 6, 5, 7, 5, 0, 2, 3, 7])] +\
[0, 0, 2, 0, 3, 1, 4, 0, 6, 5, 7, 5, 0, 2, 3, 7, 0]
, "label": [*reversed([1, 1, 0, 1, 1, 0, 0, 0, 1, 0, 0, 1, 0, 0, 0, 0])] +\
[1, 1, 0, 1, 1, 0, 0, 0, 1, 0, 0, 1, 0, 0, 0, 0, 1]
}
df = pd.DataFrame(data)
df['label'] = df['label'].astype(bool)
we have the output:
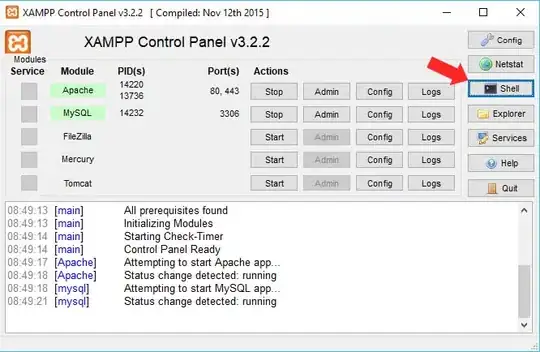