I came here today to check the state of the art.
This is a subject come up from time to time, modern CPUs have a the population count algorithm. This is useful to calculate Bit Error Rate in certain communications application. This is the hamming weight, related to Hamming distance, scipy has an implementation but it works with arrays, not with the binary representation of numbers. For fixed size computation, e.g numpy arrays, the fastest approach I know is the method this answer, however the mentioned answer, I am calling this the divide and conquer method. In the general case the divide and conquer has complexity O(log(n))
instead of O(n)
. The accepted answer is really good for most cases, and even better in languages such as C where you could simply take ((*char)bits)[i]
, instead of shifting the number.
Here I give a general implementation for the divide and conquer approach where the masks are computed dynamically depending on the size of the input number.
def dq_number_of_ones(n):
nb = 1
while n >> nb:
nb *= 2
t = (1 << nb) - 1
masks = []
while nb > 1:
nb //= 2
t ^= t >> nb
masks.append(t)
t = n
s = 1
for tm in masks[::-1]:
tm = masks.pop()
t = ((tm & t) >> s) + ((tm >> s) & t)
s *= 2
return t
And for completenes here the OP's method and the accepted lookup table method
def number_of_ones(n):
# do something
# I want to MAKE this FASTER (computationally less complex).
c = 0
while n:
c += n%2
n //= 2
return c
lookup_table = [number_of_ones(i) for i in range(256)]
def lt_number_of_ones(n):
sum = 0
while n != 0:
sum += lookup_table[n & 0xff]
n >>= 8
return sum
A practical comparison of the two
import time
import matplotlib.pyplot as plt
x = []
t1 = []
t2 = []
t3 = []
for i in range(3,8000,20):
y = i**i
if i < 1000:
time.sleep(0.0001)
t0 = time.time()
w1 = number_of_ones(y)
t1.append(time.time() - t0)
else:
t1.append(np.nan)
time.sleep(0.0001)
t0 = time.time()
w2 = dq_number_of_ones(y)
t2.append(time.time() - t0)
time.sleep(0.0001)
t0 = time.time()
_ = lt_number_of_ones(y)
t3.append(time.time() - t0)
time.sleep(0.0001)
x.append(i)
plt.figure(figsize=(12, 4))
plt.subplot(121)
plt.plot(x, t1)
plt.plot(x, t2)
plt.plot(x, t3)
plt.xlim([10,None])
plt.title('Linear scale')
plt.ylabel('time to count bits in $x^x$')
plt.subplot(122)
plt.loglog(x, t1)
plt.loglog(x, t2)
plt.loglog(x, t3)
plt.xlim([10,None])
plt.title('Log scale')
plt.legend(['direct counting', 'lookup table', 'divide and conquer'])
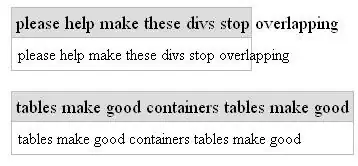