Here is one possibility, using @Vincent's code. It works with the latest release of ggplot2 (v. 0.9) and the R-forge version of directlabels (v. 2.5). I also have tested the code with ggplot2 0.8.9
and directlabels 2.4
. (The version of directlabels
released on CRAN won't work with ggplot2 0.9
, though.)
The idea is basically to replace your labels A
, B
, C
, G
with the regression equations. Of course, you could store the latter in a different manner but I think this would sensibly complicate the plotting expression, so let's keep that as simple as possible. Assuming we already have @Vincent's melted variable d
,
> head(d)
Xax variable value
1 0.22 A 0.451
2 0.34 A 0.491
3 0.54 A 0.389
4 0.34 A 0.425
5 0.53 A 0.457
6 0.12 A 0.436
let's replace variable
labels with the equations you computed:
library(plyr)
lm.stats <- ddply(d, "variable",
function(u) {
r <- lm(value ~ Xax, data=u)
c(coef(r), r.squared=summary(r)$r.squared)
})
my.formatter <- function(x, digits=2) {
x <- round(x, digits=digits)
out <- paste(x[1], ifelse(x[2]>0, "+", ""), x[2], "x", sep="")
out <- paste(out, " (R2=", x[3], ")", sep="")
return(out)
}
d$variablef <- d$variable
levels(d$variablef) <- apply(lm.stats[2:4], 1, my.formatter)
The little helper function, my.formatter
, is in charge of assembling the different statistics you computed with ddply
. Note that I made a copy of variable
in case we need this latter. And here is the plotting stuff:
p <- ggplot(d, aes(Xax,value, col=variablef)) +
geom_point() +
stat_smooth(method=lm)
library(directlabels)
direct.label(p)
I should note that you can also have annotated curves with the labcurve()
function from the Hmisc package. I can also imagine simpler solutions using ggplot or lattice, namely just write the regression equations along the regression lines, with proper orientation and a slight shift on the x-axis to avoid overlapping, but that might not necessarily be very portable if your dataset happens to change.
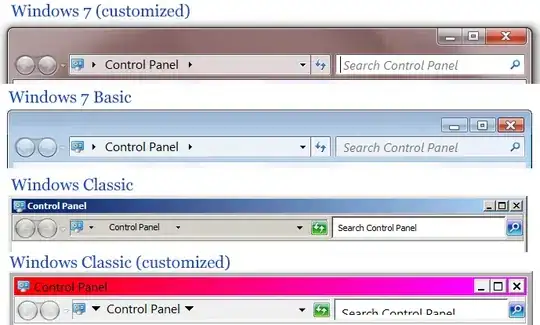