You could apply Hough transform to detect the grid lines. Once we have those you can infer the grid locations and the rotation angle:
%# load image, and process it
img = imread('print5.jpg');
img = imfilter(img, fspecial('gaussian',7,1));
BW = imcomplement(im2bw(img));
BW = imclearborder(BW);
BW(150:200,100:150) = 0; %# simulate a missing chunk!
%# detect dots centers
st = regionprops(BW, 'Centroid');
c = vertcat(st.Centroid);
%# hough transform, detect peaks, then get lines segments
[H,T,R] = hough(BW);
P = houghpeaks(H, 25);
L = houghlines(BW, T, R, P);
%# show image with overlayed connected components, their centers + detected lines
I = imoverlay(img, BW, [0.9 0.1 0.1]);
imshow(I, 'InitialMag',200, 'Border','tight'), hold on
line(c(:,1), c(:,2), 'LineStyle','none', 'Marker','+', 'Color','b')
for k = 1:length(L)
xy = [L(k).point1; L(k).point2];
plot(xy(:,1), xy(:,2), 'g-', 'LineWidth',2);
end
hold off
(I'm using imoverlay
function from the File Exchange)
The result:

Here is the accumulator matrix with the peaks corresponding to the lines detected highlighted:
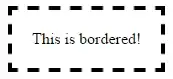
Now we can recover the angle of rotation by computing the mean slope of detected lines, filtered to those in one of the two directions (horizontals or verticals):
%# filter lines to extract almost vertical ones
%# Note that theta range is (-90:89), angle = theta + 90
LL = L( abs([L.theta]) < 30 );
%# compute the mean slope of those lines
slopes = vertcat(LL.point2) - vertcat(LL.point1);
slopes = atan2(slopes(:,2),slopes(:,1));
r = mean(slopes);
%# transform image by applying the inverse of the rotation
tform = maketform('affine', [cos(r) sin(r) 0; -sin(r) cos(r) 0; 0 0 1]);
img_align = imtransform(img, fliptform(tform));
imshow(img_align)
Here is the image rotated back so that the grid is aligned with the xy-axes:
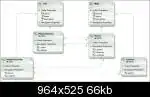