Months later, here's a small class PCA, and a picture:
#!/usr/bin/env python
""" a small class for Principal Component Analysis
Usage:
p = PCA( A, fraction=0.90 )
In:
A: an array of e.g. 1000 observations x 20 variables, 1000 rows x 20 columns
fraction: use principal components that account for e.g.
90 % of the total variance
Out:
p.U, p.d, p.Vt: from numpy.linalg.svd, A = U . d . Vt
p.dinv: 1/d or 0, see NR
p.eigen: the eigenvalues of A*A, in decreasing order (p.d**2).
eigen[j] / eigen.sum() is variable j's fraction of the total variance;
look at the first few eigen[] to see how many PCs get to 90 %, 95 % ...
p.npc: number of principal components,
e.g. 2 if the top 2 eigenvalues are >= `fraction` of the total.
It's ok to change this; methods use the current value.
Methods:
The methods of class PCA transform vectors or arrays of e.g.
20 variables, 2 principal components and 1000 observations,
using partial matrices U' d' Vt', parts of the full U d Vt:
A ~ U' . d' . Vt' where e.g.
U' is 1000 x 2
d' is diag([ d0, d1 ]), the 2 largest singular values
Vt' is 2 x 20. Dropping the primes,
d . Vt 2 principal vars = p.vars_pc( 20 vars )
U 1000 obs = p.pc_obs( 2 principal vars )
U . d . Vt 1000 obs, p.obs( 20 vars ) = pc_obs( vars_pc( vars ))
fast approximate A . vars, using the `npc` principal components
Ut 2 pcs = p.obs_pc( 1000 obs )
V . dinv 20 vars = p.pc_vars( 2 principal vars )
V . dinv . Ut 20 vars, p.vars( 1000 obs ) = pc_vars( obs_pc( obs )),
fast approximate Ainverse . obs: vars that give ~ those obs.
Notes:
PCA does not center or scale A; you usually want to first
A -= A.mean(A, axis=0)
A /= A.std(A, axis=0)
with the little class Center or the like, below.
See also:
http://en.wikipedia.org/wiki/Principal_component_analysis
http://en.wikipedia.org/wiki/Singular_value_decomposition
Press et al., Numerical Recipes (2 or 3 ed), SVD
PCA micro-tutorial
iris-pca .py .png
"""
from __future__ import division
import numpy as np
dot = np.dot
# import bz.numpyutil as nu
# dot = nu.pdot
__version__ = "2010-04-14 apr"
__author_email__ = "denis-bz-py at t-online dot de"
#...............................................................................
class PCA:
def __init__( self, A, fraction=0.90 ):
assert 0 <= fraction <= 1
# A = U . diag(d) . Vt, O( m n^2 ), lapack_lite --
self.U, self.d, self.Vt = np.linalg.svd( A, full_matrices=False )
assert np.all( self.d[:-1] >= self.d[1:] ) # sorted
self.eigen = self.d**2
self.sumvariance = np.cumsum(self.eigen)
self.sumvariance /= self.sumvariance[-1]
self.npc = np.searchsorted( self.sumvariance, fraction ) + 1
self.dinv = np.array([ 1/d if d > self.d[0] * 1e-6 else 0
for d in self.d ])
def pc( self ):
""" e.g. 1000 x 2 U[:, :npc] * d[:npc], to plot etc. """
n = self.npc
return self.U[:, :n] * self.d[:n]
# These 1-line methods may not be worth the bother;
# then use U d Vt directly --
def vars_pc( self, x ):
n = self.npc
return self.d[:n] * dot( self.Vt[:n], x.T ).T # 20 vars -> 2 principal
def pc_vars( self, p ):
n = self.npc
return dot( self.Vt[:n].T, (self.dinv[:n] * p).T ) .T # 2 PC -> 20 vars
def pc_obs( self, p ):
n = self.npc
return dot( self.U[:, :n], p.T ) # 2 principal -> 1000 obs
def obs_pc( self, obs ):
n = self.npc
return dot( self.U[:, :n].T, obs ) .T # 1000 obs -> 2 principal
def obs( self, x ):
return self.pc_obs( self.vars_pc(x) ) # 20 vars -> 2 principal -> 1000 obs
def vars( self, obs ):
return self.pc_vars( self.obs_pc(obs) ) # 1000 obs -> 2 principal -> 20 vars
class Center:
""" A -= A.mean() /= A.std(), inplace -- use A.copy() if need be
uncenter(x) == original A . x
"""
# mttiw
def __init__( self, A, axis=0, scale=True, verbose=1 ):
self.mean = A.mean(axis=axis)
if verbose:
print "Center -= A.mean:", self.mean
A -= self.mean
if scale:
std = A.std(axis=axis)
self.std = np.where( std, std, 1. )
if verbose:
print "Center /= A.std:", self.std
A /= self.std
else:
self.std = np.ones( A.shape[-1] )
self.A = A
def uncenter( self, x ):
return np.dot( self.A, x * self.std ) + np.dot( x, self.mean )
#...............................................................................
if __name__ == "__main__":
import sys
csv = "iris4.csv" # wikipedia Iris_flower_data_set
# 5.1,3.5,1.4,0.2 # ,Iris-setosa ...
N = 1000
K = 20
fraction = .90
seed = 1
exec "\n".join( sys.argv[1:] ) # N= ...
np.random.seed(seed)
np.set_printoptions( 1, threshold=100, suppress=True ) # .1f
try:
A = np.genfromtxt( csv, delimiter="," )
N, K = A.shape
except IOError:
A = np.random.normal( size=(N, K) ) # gen correlated ?
print "csv: %s N: %d K: %d fraction: %.2g" % (csv, N, K, fraction)
Center(A)
print "A:", A
print "PCA ..." ,
p = PCA( A, fraction=fraction )
print "npc:", p.npc
print "% variance:", p.sumvariance * 100
print "Vt[0], weights that give PC 0:", p.Vt[0]
print "A . Vt[0]:", dot( A, p.Vt[0] )
print "pc:", p.pc()
print "\nobs <-> pc <-> x: with fraction=1, diffs should be ~ 0"
x = np.ones(K)
# x = np.ones(( 3, K ))
print "x:", x
pc = p.vars_pc(x) # d' Vt' x
print "vars_pc(x):", pc
print "back to ~ x:", p.pc_vars(pc)
Ax = dot( A, x.T )
pcx = p.obs(x) # U' d' Vt' x
print "Ax:", Ax
print "A'x:", pcx
print "max |Ax - A'x|: %.2g" % np.linalg.norm( Ax - pcx, np.inf )
b = Ax # ~ back to original x, Ainv A x
back = p.vars(b)
print "~ back again:", back
print "max |back - x|: %.2g" % np.linalg.norm( back - x, np.inf )
# end pca.py
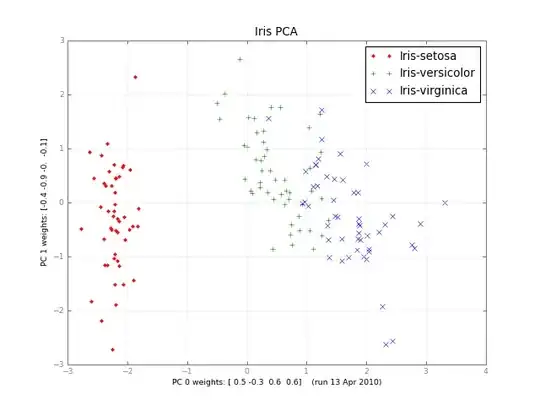