I've adjusted my RANSAC algorithm from this answer: Detect semi-circle in opencv
Idea:
- choose randomly 3 points from your binary edge image
- create a circle from those 3 points
- test how "good" this circle is
if it is better than the previously best found circle in this image, remember
loop 1-4 until some number of iterations reached. then accept the best found circle.
remove that accepted circle from the image
repeat 1-6 until you have found all circles
problems:
- at the moment you must know how many circles you want to find in the image
- tested only for that one image.
- c++ code
result:
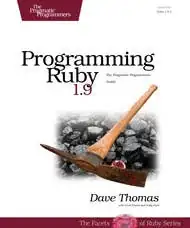
code:
inline void getCircle(cv::Point2f& p1,cv::Point2f& p2,cv::Point2f& p3, cv::Point2f& center, float& radius)
{
float x1 = p1.x;
float x2 = p2.x;
float x3 = p3.x;
float y1 = p1.y;
float y2 = p2.y;
float y3 = p3.y;
// PLEASE CHECK FOR TYPOS IN THE FORMULA :)
center.x = (x1*x1+y1*y1)*(y2-y3) + (x2*x2+y2*y2)*(y3-y1) + (x3*x3+y3*y3)*(y1-y2);
center.x /= ( 2*(x1*(y2-y3) - y1*(x2-x3) + x2*y3 - x3*y2) );
center.y = (x1*x1 + y1*y1)*(x3-x2) + (x2*x2+y2*y2)*(x1-x3) + (x3*x3 + y3*y3)*(x2-x1);
center.y /= ( 2*(x1*(y2-y3) - y1*(x2-x3) + x2*y3 - x3*y2) );
radius = sqrt((center.x-x1)*(center.x-x1) + (center.y-y1)*(center.y-y1));
}
std::vector<cv::Point2f> getPointPositions(cv::Mat binaryImage)
{
std::vector<cv::Point2f> pointPositions;
for(unsigned int y=0; y<binaryImage.rows; ++y)
{
//unsigned char* rowPtr = binaryImage.ptr<unsigned char>(y);
for(unsigned int x=0; x<binaryImage.cols; ++x)
{
//if(rowPtr[x] > 0) pointPositions.push_back(cv::Point2i(x,y));
if(binaryImage.at<unsigned char>(y,x) > 0) pointPositions.push_back(cv::Point2f(x,y));
}
}
return pointPositions;
}
float verifyCircle(cv::Mat dt, cv::Point2f center, float radius, std::vector<cv::Point2f> & inlierSet)
{
unsigned int counter = 0;
unsigned int inlier = 0;
float minInlierDist = 2.0f;
float maxInlierDistMax = 100.0f;
float maxInlierDist = radius/25.0f;
if(maxInlierDist<minInlierDist) maxInlierDist = minInlierDist;
if(maxInlierDist>maxInlierDistMax) maxInlierDist = maxInlierDistMax;
// choose samples along the circle and count inlier percentage
for(float t =0; t<2*3.14159265359f; t+= 0.05f)
{
counter++;
float cX = radius*cos(t) + center.x;
float cY = radius*sin(t) + center.y;
if(cX < dt.cols)
if(cX >= 0)
if(cY < dt.rows)
if(cY >= 0)
if(dt.at<float>(cY,cX) < maxInlierDist)
{
inlier++;
inlierSet.push_back(cv::Point2f(cX,cY));
}
}
return (float)inlier/float(counter);
}
float evaluateCircle(cv::Mat dt, cv::Point2f center, float radius)
{
float completeDistance = 0.0f;
int counter = 0;
float maxDist = 1.0f; //TODO: this might depend on the size of the circle!
float minStep = 0.001f;
// choose samples along the circle and count inlier percentage
//HERE IS THE TRICK that no minimum/maximum circle is used, the number of generated points along the circle depends on the radius.
// if this is too slow for you (e.g. too many points created for each circle), increase the step parameter, but only by factor so that it still depends on the radius
// the parameter step depends on the circle size, otherwise small circles will create more inlier on the circle
float step = 2*3.14159265359f / (6.0f * radius);
if(step < minStep) step = minStep; // TODO: find a good value here.
//for(float t =0; t<2*3.14159265359f; t+= 0.05f) // this one which doesnt depend on the radius, is much worse!
for(float t =0; t<2*3.14159265359f; t+= step)
{
float cX = radius*cos(t) + center.x;
float cY = radius*sin(t) + center.y;
if(cX < dt.cols)
if(cX >= 0)
if(cY < dt.rows)
if(cY >= 0)
if(dt.at<float>(cY,cX) <= maxDist)
{
completeDistance += dt.at<float>(cY,cX);
counter++;
}
}
return counter;
}
int main()
{
//RANSAC
cv::Mat color = cv::imread("HoughCirclesAccuracy.png");
// convert to grayscale
cv::Mat gray;
cv::cvtColor(color, gray, CV_RGB2GRAY);
// get binary image
cv::Mat mask = gray > 0;
unsigned int numberOfCirclesToDetect = 2; // TODO: if unknown, you'll have to find some nice criteria to stop finding more (semi-) circles
for(unsigned int j=0; j<numberOfCirclesToDetect; ++j)
{
std::vector<cv::Point2f> edgePositions;
edgePositions = getPointPositions(mask);
std::cout << "number of edge positions: " << edgePositions.size() << std::endl;
// create distance transform to efficiently evaluate distance to nearest edge
cv::Mat dt;
cv::distanceTransform(255-mask, dt,CV_DIST_L1, 3);
unsigned int nIterations = 0;
cv::Point2f bestCircleCenter;
float bestCircleRadius;
//float bestCVal = FLT_MAX;
float bestCVal = -1;
//float minCircleRadius = 20.0f; // TODO: if you have some knowledge about your image you might be able to adjust the minimum circle radius parameter.
float minCircleRadius = 0.0f;
//TODO: implement some more intelligent ransac without fixed number of iterations
for(unsigned int i=0; i<2000; ++i)
{
//RANSAC: randomly choose 3 point and create a circle:
//TODO: choose randomly but more intelligent,
//so that it is more likely to choose three points of a circle.
//For example if there are many small circles, it is unlikely to randomly choose 3 points of the same circle.
unsigned int idx1 = rand()%edgePositions.size();
unsigned int idx2 = rand()%edgePositions.size();
unsigned int idx3 = rand()%edgePositions.size();
// we need 3 different samples:
if(idx1 == idx2) continue;
if(idx1 == idx3) continue;
if(idx3 == idx2) continue;
// create circle from 3 points:
cv::Point2f center; float radius;
getCircle(edgePositions[idx1],edgePositions[idx2],edgePositions[idx3],center,radius);
if(radius < minCircleRadius)continue;
//verify or falsify the circle by inlier counting:
//float cPerc = verifyCircle(dt,center,radius, inlierSet);
float cVal = evaluateCircle(dt,center,radius);
if(cVal > bestCVal)
{
bestCVal = cVal;
bestCircleRadius = radius;
bestCircleCenter = center;
}
++nIterations;
}
std::cout << "current best circle: " << bestCircleCenter << " with radius: " << bestCircleRadius << " and nInlier " << bestCVal << std::endl;
cv::circle(color,bestCircleCenter,bestCircleRadius,cv::Scalar(0,0,255));
//TODO: hold and save the detected circle.
//TODO: instead of overwriting the mask with a drawn circle it might be better to hold and ignore detected circles and dont count new circles which are too close to the old one.
// in this current version the chosen radius to overwrite the mask is fixed and might remove parts of other circles too!
// update mask: remove the detected circle!
cv::circle(mask,bestCircleCenter, bestCircleRadius, 0, 10); // here the radius is fixed which isnt so nice.
}
cv::namedWindow("edges"); cv::imshow("edges", mask);
cv::namedWindow("color"); cv::imshow("color", color);
cv::imwrite("detectedCircles.png", color);
cv::waitKey(-1);
return 0;
}