This is a derivative of a previous post where I provided an answer to here: matlab template matching only for 0 (or 1) in matrix
However, this solution used for
loops which is quite inefficient. As such, we can use im2col
, bsxfun
and col2im
to help us perform this more quickly. im2col
takes overlapping regions in your image and places them each into individual columns. Essentially, this takes sliding windows of your image like you would with any kind of spatial image filtering and collects all pixels within a sliding window and places each window as individual columns.
Supposing the size of your template is M x N
, and the size of your image you want to search in is R x C
, and supposing that your image template is called imTemplate
while the image you want to search in is imSearch
, we can do the following setup. Let's also assume that both images are binary.
[M, N] = size(imTemplate);
[R, C] = size(imSearch);
%// Cast to double for precision
imTemplate = im2double(imTemplate);
imSearch = im2double(imSearch);
neigh = im2col(imSearch, [M, N]);
templateCol = imTemplate(:); %// Ensures we place template into single column
Now, you wish to exclude all pixels that are inside the circular boundary. As such, what we can do is invert the image so that black pixels become white, then remove all of the pixels around the border. This should then give us the interior of the circle.
imInvert = ~imTemplate;
imInvertNoBorder = imclearborder(imInvert, 8); %// Search 8-pixel neighbourhood
We will use this to figure out what pixels we are going to remove from searching. This can be done by:
rowsToRemove = imInvertNoBorder(:) == 1;
Now, what we can do is finally remove those pixels that are within the interior of the circle to not be searched in our correlation scheme.
neigh(rowsToRemove,:) = [];
What we can do now is compute the NCC over all of these columns. If you recall, the NCC between two signals is the following:
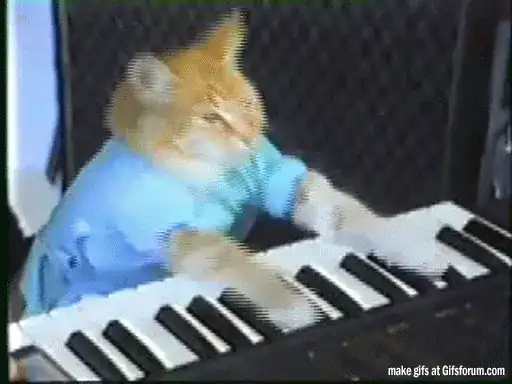
(source: www.jot.fm)
As such, we need to subtract the mean from each neighbourhood, and we also need to subtract the mean from each of the columns. We then compute the formula as shown above. We can easily achieve this vectorized in MATLAB like so:
neighMeanSubtract = bsxfun(@minus, neigh, mean(neigh));
templateMeanSubtract = templateCol - mean(templateCol);
We can compute the numerator of the NCC for each neighbourhood (before we sum) as follows:
numerator = bsxfun(@times, neighMeanSubtract, templateMeanSubtract);
Now, all we have to do is sum all of the columns and that will give us our final numerator:
sumNumerator = sum(numerator);
The denominator can be computed like so:
denominator1 = sqrt(sum(neighMeanSubtract.*neighMeanSubtract));
denominator2 = sqrt(sum(templateMeanSubtract.*templateMeanSubtract));
sumDenominator = denominator1 .* denominator2;
Finally, our NCC can be computed as so:
NCC = sumNumerator ./ sumDenominator;
You'll notice that this is a single row of values. Each row corresponds to the output defined at a neighbourhood. As such, we also need to reshape this back into a matrix, and so you can use col2im
:
finalOutput = col2im(NCC, [M, N], [R, C]);
The above statement will take overlapping neighbourhoods of M x N
defined in NCC, and reshapes it so that it becomes a R x C
matrix. Sometimes, you will get a divide by zero error, especially if the neighbourhood search window is all uniform. As such, you will get NaN
numbers. Areas of no variation are assumed to have no correlation in image processing, and so let's zero these locations:
finalOutput(isnan(finalOutput)) = 0;
If you want to find the location of where the highest correlation is, simply do:
[rowNCC, colNCC] = find(finalOutput == max(finalOutput(:)));
If you want to interpret negative correlation, that completely depends on your application. If you want to ensure that your template matching algorithm is rotationally invariant, then you should actually check for the max of the absolute values. Negative correlation simply means that the match between the template and a neighbourhood is simply rotated. As such, a better way to find the best neighbourhood is:
maxCoeff = max(abs(finalOutput(:)));
[rowNCC, colNCC] = find(abs(finalOutput) == maxCoeff);
For your copying and pasting pleasure, here is the code in its entirety:
function [rowNCC, colNCC] = testCorr(imTemplate, imSearch)
[M, N] = size(imTemplate);
[R, C] = size(imSearch);
%// Cast to double for precision
imTemplate = im2double(imTemplate);
imSearch = im2double(imSearch);
neigh = im2col(imSearch, [M, N]);
templateCol = imTemplate(:); %// Ensures we place template into single column
imInvert = ~imTemplate;
imInvertNoBorder = imclearborder(imInvert, 8); %// Search 8-pixel neighbourhood
rowsToRemove = imInvertNoBorder(:) == 1;
neigh(rowsToRemove,:) = [];
neighMeanSubtract = bsxfun(@minus, neigh, mean(neigh));
templateMeanSubtract = templateCol - mean(templateCol);
numerator = bsxfun(@times, neighMeanSubtract, templateMeanSubtract);
sumNumerator = sum(numerator);
denominator1 = sqrt(sum(neighMeanSubtract.*neighMeanSubtract));
denominator2 = sqrt(sum(templateMeanSubtract.*templateMeanSubtract));
sumDenominator = denominator1 .* denominator2;
NCC = sumNumerator ./ sumDenominator;
finalOutput = col2im(NCC, [M, N], [R, C]);
finalOutput(isnan(finalOutput)) = 0;
maxCoeff = max(abs(finalOutput(:)));
[rowNCC, colNCC] = find(abs(finalOutput) == maxCoeff);
end
Good luck!