Here's a way to shade the area between two density plots and calculate the magnitude of that area.
# Create some fake data
set.seed(10)
dat = data.frame(x=c(rnorm(1000, 0, 5), rnorm(2000, 0, 1)),
group=c(rep("Bad", 1000), rep("Good", 2000)))
# Plot densities
# Use y=..count.. to get counts on the vertical axis
p1 = ggplot(dat) +
geom_density(aes(x=x, y=..count.., colour=group), lwd=1)
Some extra calculations to shade the area between the two density plots
(adapted from this SO question):
pp1 = ggplot_build(p1)
# Create a new data frame with densities for the two groups ("Bad" and "Good")
dat2 = data.frame(x = pp1$data[[1]]$x[pp1$data[[1]]$group==1],
ymin=pp1$data[[1]]$y[pp1$data[[1]]$group==1],
ymax=pp1$data[[1]]$y[pp1$data[[1]]$group==2])
# We want ymax and ymin to differ only when the density of "Good"
# is greater than the density of "Bad"
dat2$ymax[dat2$ymax < dat2$ymin] = dat2$ymin[dat2$ymax < dat2$ymin]
# Shade the area between "Good" and "Bad"
p1a = p1 +
geom_ribbon(data=dat2, aes(x=x, ymin=ymin, ymax=ymax), fill='yellow', alpha=0.5)
Here are the two plots:
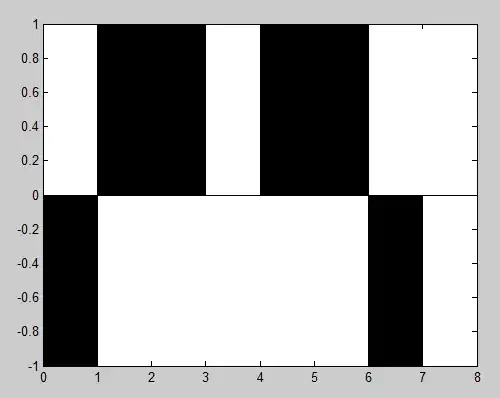
To get the area (number of values) in specific ranges of Good
and Bad
, use the density
function on each group (or you can continue to work with the data pulled from ggplot
as above, but this way you get more direct control over how the density distribution is generated):
## Calculate densities for Bad and Good.
# Use same number of points and same x-range for each group, so that the density
# values will line up. Use a higher value for n to get a finer x-grid for the density
# values. Use a power of 2 for n, because the density function rounds up to the nearest
# power of 2 anyway.
bad = density(dat$x[dat$group=="Bad"],
n=1024, from=min(dat$x), to=max(dat$x))
good = density(dat$x[dat$group=="Good"],
n=1024, from=min(dat$x), to=max(dat$x))
## Normalize so that densities sum to number of rows in each group
# Number of rows in each group
counts = tapply(dat$x, dat$group, length)
bad$y = counts[1]/sum(bad$y) * bad$y
good$y = counts[2]/sum(good$y) * good$y
## Results
# Number of "Good" in region where "Good" exceeds "Bad"
sum(good$y[good$y > bad$y])
[1] 1931.495 # Out of 2000 total in the data frame
# Number of "Bad" in region where "Good" exceeds "Bad"
sum(bad$y[good$y > bad$y])
[1] 317.7315 # Out of 1000 total in the data frame