This can be done using the Python bindings to the OpenCV
library. The following code has been adapted from something I had already, so it can probably be further optimised and improved.
The image you have given is not only rotated, but it is also not rectangular, as such the script works in two main stages. Firstly it determines the rotation on the image and rotates and crops it around a minimum rectangle. It then stretches the resulting image to fit the resulting rectangle.
Initial threshold image

Initial bounding rectangle
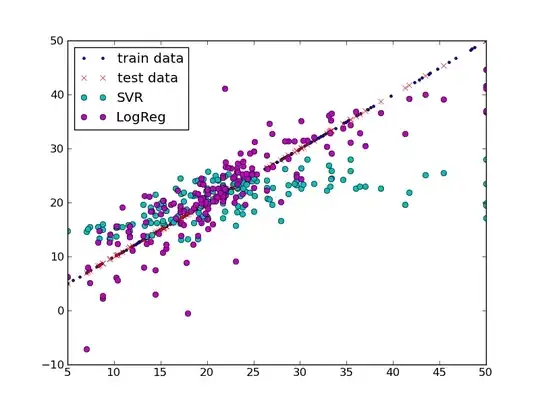
Rotated and cropped image

Polygon to stretch from
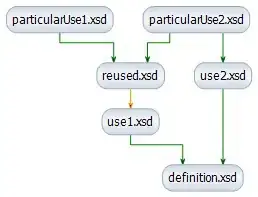
Final cropped image
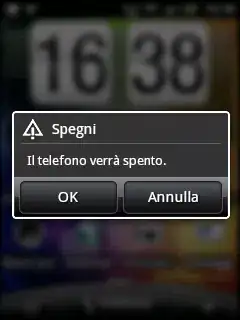
import numpy as np
import cv2
import math
THRESHOLD = 240
def subimage(image, center, theta, width, height):
if 45 < theta <= 90:
theta = theta - 90
width, height = height, width
theta *= math.pi / 180 # convert to rad
v_x = (math.cos(theta), math.sin(theta))
v_y = (-math.sin(theta), math.cos(theta))
s_x = center[0] - v_x[0] * (width / 2) - v_y[0] * (height / 2)
s_y = center[1] - v_x[1] * (width / 2) - v_y[1] * (height / 2)
mapping = np.array([[v_x[0],v_y[0], s_x], [v_x[1],v_y[1], s_y]])
return cv2.warpAffine(image, mapping, (width, height), flags=cv2.WARP_INVERSE_MAP, borderMode=cv2.BORDER_REPLICATE)
def auto_crop(image_source):
# First slightly crop edge - some images had a rogue 2 pixel black edge on one side
init_crop = 5
h, w = image_source.shape[:2]
image_source = image_source[init_crop:init_crop+(h-init_crop*2), init_crop:init_crop+(w-init_crop*2)]
# Add back a white border
image_source = cv2.copyMakeBorder(image_source, 5,5,5,5, cv2.BORDER_CONSTANT, value=(255,255,255))
image_gray = cv2.cvtColor(image_source, cv2.COLOR_BGR2GRAY)
_, image_thresh = cv2.threshold(image_gray, THRESHOLD, 255, cv2.THRESH_BINARY)
image_thresh2 = image_thresh.copy()
image_thresh2 = cv2.Canny(image_thresh2, 100, 100, apertureSize=3)
points = cv2.findNonZero(image_thresh2)
centre, dimensions, theta = cv2.minAreaRect(points)
rect = cv2.minAreaRect(points)
width = int(dimensions[0])
height = int(dimensions[1])
box = cv2.boxPoints(rect)
box = np.int0(box)
temp = image_source.copy()
cv2.drawContours(temp, [box], 0, (255,0,0), 2)
M = cv2.moments(box)
cx = int(M['m10']/M['m00'])
cy = int(M['m01']/M['m00'])
image_patch = subimage(image_source, (cx, cy), theta+90, height, width)
# add back a small border
image_patch = cv2.copyMakeBorder(image_patch, 1,1,1,1, cv2.BORDER_CONSTANT, value=(255,255,255))
# Convert image to binary, edge is black. Do edge detection and convert edges to a list of points.
# Then calculate a minimum set of points that can enclose the points.
_, image_thresh = cv2.threshold(image_patch, THRESHOLD, 255, 1)
image_thresh = cv2.Canny(image_thresh, 100, 100, 3)
points = cv2.findNonZero(image_thresh)
hull = cv2.convexHull(points)
# Find min epsilon resulting in exactly 4 points, typically between 7 and 21
# This is the smallest set of 4 points to enclose the image.
for epsilon in range(3, 50):
hull_simple = cv2.approxPolyDP(hull, epsilon, 1)
if len(hull_simple) == 4:
break
hull = hull_simple
# Find closest fitting image size and warp/crop to fit
# (ie reduce scaling to a minimum)
x,y,w,h = cv2.boundingRect(hull)
target_corners = np.array([[0,0],[w,0],[w,h],[0,h]], np.float32)
# Sort hull into tl,tr,br,bl order.
# n.b. hull is already sorted in clockwise order, we just need to know where top left is.
source_corners = hull.reshape(-1,2).astype('float32')
min_dist = 100000
index = 0
for n in xrange(len(source_corners)):
x,y = source_corners[n]
dist = math.hypot(x,y)
if dist < min_dist:
index = n
min_dist = dist
# Rotate the array so tl is first
source_corners = np.roll(source_corners , -(2*index))
try:
transform = cv2.getPerspectiveTransform(source_corners, target_corners)
return cv2.warpPerspective(image_patch, transform, (w,h))
except:
print "Warp failure"
return image_patch
cv2.namedWindow("Result")
image_src = cv2.imread("xray.png")
image_cropped = auto_crop(image_src)
cv2.imwrite("cropped xray.png", image_cropped)
cv2.imshow("Result", image_cropped)
cv2.waitKey(0)
Thanks go to this StackOverflow answer for the subimage
function.
Tested on Python 2.7 and OpenCV 3.0