You can do this efficiently using isin
on a multiindex constructed from the desired columns:
df1 = pd.DataFrame({'c': ['A', 'A', 'B', 'C', 'C'],
'k': [1, 2, 2, 2, 2],
'l': ['a', 'b', 'a', 'a', 'd']})
df2 = pd.DataFrame({'c': ['A', 'C'],
'l': ['b', 'a']})
keys = list(df2.columns.values)
i1 = df1.set_index(keys).index
i2 = df2.set_index(keys).index
df1[~i1.isin(i2)]
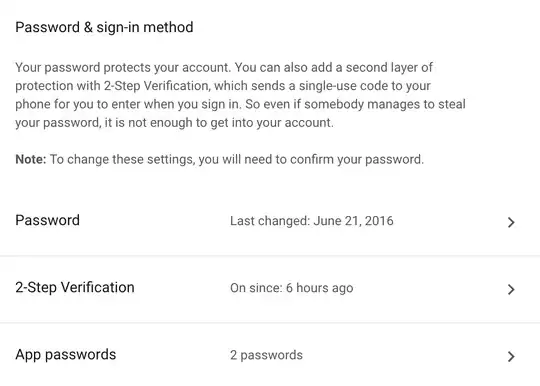
I think this improves on @IanS's similar solution because it doesn't assume any column type (i.e. it will work with numbers as well as strings).
(Above answer is an edit. Following was my initial answer)
Interesting! This is something I haven't come across before... I would probably solve it by merging the two arrays, then dropping rows where df2
is defined. Here is an example, which makes use of a temporary array:
df1 = pd.DataFrame({'c': ['A', 'A', 'B', 'C', 'C'],
'k': [1, 2, 2, 2, 2],
'l': ['a', 'b', 'a', 'a', 'd']})
df2 = pd.DataFrame({'c': ['A', 'C'],
'l': ['b', 'a']})
# create a column marking df2 values
df2['marker'] = 1
# join the two, keeping all of df1's indices
joined = pd.merge(df1, df2, on=['c', 'l'], how='left')
joined
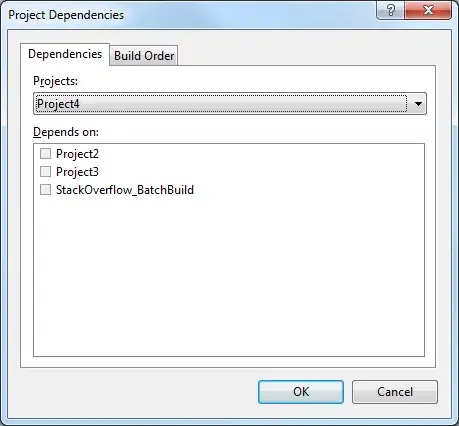
# extract desired columns where marker is NaN
joined[pd.isnull(joined['marker'])][df1.columns]
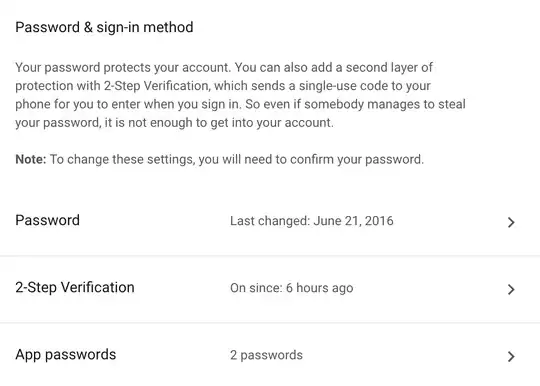
There may be a way to do this without using the temporary array, but I can't think of one. As long as your data isn't huge the above method should be a fast and sufficient answer.