I guess you could achieve that by clipping your result by the convex hull of your points. To do that I would probably use the shapely
module.
Given the SO post you linked I assume you are using the voronoi_finite_polygons_2d
function written in the post. So i think this could do the job:
import numpy as np
import matplotlib.pyplot as plt
from shapely.geometry import MultiPoint, Point, Polygon
from scipy.spatial import Voronoi
points = [[-30.0, 30.370371], [-27.777777, 35.925926], [-34.444443, 58.51852], [-2.9629631, 57.777779], [-17.777779, 75.185181], [-29.25926, 58.148151], [-11.111112, 33.703705], [-11.481482, 40.0], [-27.037037, 40.0], [-7.7777777, 94.444443], [-2.2222223, 122.22222], [-20.370371, 106.66667], [1.1111112, 125.18518], [-6.2962961, 128.88889], [6.666667, 133.7037], [11.851852, 136.2963], [8.5185184, 140.74074], [20.370371, 92.962959], [17.777779, 114.81482], [12.962962, 97.037041], [13.333334, 127.77778], [22.592592, 120.37037], [16.296295, 127.77778], [11.851852, 50.740742], [20.370371, 54.814816], [19.25926, 47.40741], [32.59259, 122.96296], [20.74074, 130.0], [24.814816, 84.814819], [26.296295, 91.111107], [56.296295, 131.48149], [60.0, 141.85185], [32.222221, 136.66667], [53.703705, 147.03703], [87.40741, 196.2963], [34.074074, 159.62964], [34.444443, -2.5925925], [36.666668, -1.8518518], [34.074074, -7.4074073], [35.555557, -18.888889], [76.666664, -39.629627], [35.185184, -37.777779], [25.185184, 14.074074], [42.962959, 32.962963], [35.925926, 9.2592592], [52.222221, 77.777779], [57.777779, 92.222221], [47.037041, 92.59259], [82.222221, 54.074074], [48.888889, 24.444445], [35.925926, 47.777779], [50.740742, 69.259254], [51.111111, 51.851849], [56.666664, -12.222222], [117.40741, -4.4444447], [59.629631, -5.9259262], [66.666664, 134.07408], [91.481483, 127.40741], [66.666664, 141.48149], [53.703705, 4.0740738], [85.185181, 11.851852], [69.629631, 0.37037039], [68.518517, 99.259262], [75.185181, 100.0], [70.370369, 113.7037], [74.444443, 82.59259], [82.222221, 93.703697], [72.222221, 84.444443], [77.777779, 167.03703], [88.888893, 168.88889], [73.703705, 178.88889], [87.037041, 123.7037], [78.518517, 97.037041], [95.555557, 52.962959], [85.555557, 57.037041], [90.370369, 23.333332], [100.0, 28.51852], [88.888893, 37.037037], [87.037041, -42.962959], [89.259262, -24.814816], [93.333328, 7.4074073], [98.518517, 5.185185], [92.59259, 1.4814816], [85.925919, 153.7037], [95.555557, 154.44444], [92.962959, 150.0], [97.037041, 95.925919], [106.66667, 115.55556], [92.962959, 114.81482], [108.88889, 56.296295], [97.777779, 50.740742], [94.074081, 89.259262], [96.666672, 91.851852], [102.22222, 77.777779], [107.40741, 40.370369], [105.92592, 29.629629], [105.55556, -46.296295], [118.51852, -47.777779], [112.22222, -43.333336], [112.59259, 25.185184], [115.92592, 27.777777], [112.59259, 31.851852], [107.03704, -36.666668], [118.88889, -32.59259], [114.07408, -25.555555], [115.92592, 85.185181], [105.92592, 18.888889], [121.11111, 14.444445], [129.25926, -28.51852], [127.03704, -18.518518], [139.25926, -12.222222], [141.48149, 3.7037036], [137.03703, -4.814815], [153.7037, -26.666668], [-2.2222223, 5.5555558], [0.0, 9.6296301], [10.74074, 20.74074], [2.2222223, 54.074074], [4.0740738, 50.740742], [34.444443, 46.296295], [11.481482, 1.4814816], [24.074076, -2.9629631], [74.814819, 79.259254], [67.777779, 152.22223], [57.037041, 127.03704], [89.259262, 12.222222]]
points = np.array(points)
vor = Voronoi(points)
regions, vertices = voronoi_finite_polygons_2d(vor)
pts = MultiPoint([Point(i) for i in points])
mask = pts.convex_hull
new_vertices = []
for region in regions:
polygon = vertices[region]
shape = list(polygon.shape)
shape[0] += 1
p = Polygon(np.append(polygon, polygon[0]).reshape(*shape)).intersection(mask)
poly = np.array(list(zip(p.boundary.coords.xy[0][:-1], p.boundary.coords.xy[1][:-1])))
new_vertices.append(poly)
plt.fill(*zip(*poly), alpha=0.4)
plt.plot(points[:,0], points[:,1], 'ko')
plt.title("Clipped Voronois")
plt.show()

More generally speaking (i.e without using voronoi_finite_polygons_2d
but using directly the output of Voronoi
if it fits my need), i would do :
import numpy as np
import matplotlib.pyplot as plt
from shapely.ops import polygonize,unary_union
from shapely.geometry import LineString, MultiPolygon, MultiPoint, Point
from scipy.spatial import Voronoi
points = [[-30.0, 30.370371], [-27.777777, 35.925926], [-34.444443, 58.51852], [-2.9629631, 57.777779], [-17.777779, 75.185181], [-29.25926, 58.148151], [-11.111112, 33.703705], [-11.481482, 40.0], [-27.037037, 40.0], [-7.7777777, 94.444443], [-2.2222223, 122.22222], [-20.370371, 106.66667], [1.1111112, 125.18518], [-6.2962961, 128.88889], [6.666667, 133.7037], [11.851852, 136.2963], [8.5185184, 140.74074], [20.370371, 92.962959], [17.777779, 114.81482], [12.962962, 97.037041], [13.333334, 127.77778], [22.592592, 120.37037], [16.296295, 127.77778], [11.851852, 50.740742], [20.370371, 54.814816], [19.25926, 47.40741], [32.59259, 122.96296], [20.74074, 130.0], [24.814816, 84.814819], [26.296295, 91.111107], [56.296295, 131.48149], [60.0, 141.85185], [32.222221, 136.66667], [53.703705, 147.03703], [87.40741, 196.2963], [34.074074, 159.62964], [34.444443, -2.5925925], [36.666668, -1.8518518], [34.074074, -7.4074073], [35.555557, -18.888889], [76.666664, -39.629627], [35.185184, -37.777779], [25.185184, 14.074074], [42.962959, 32.962963], [35.925926, 9.2592592], [52.222221, 77.777779], [57.777779, 92.222221], [47.037041, 92.59259], [82.222221, 54.074074], [48.888889, 24.444445], [35.925926, 47.777779], [50.740742, 69.259254], [51.111111, 51.851849], [56.666664, -12.222222], [117.40741, -4.4444447], [59.629631, -5.9259262], [66.666664, 134.07408], [91.481483, 127.40741], [66.666664, 141.48149], [53.703705, 4.0740738], [85.185181, 11.851852], [69.629631, 0.37037039], [68.518517, 99.259262], [75.185181, 100.0], [70.370369, 113.7037], [74.444443, 82.59259], [82.222221, 93.703697], [72.222221, 84.444443], [77.777779, 167.03703], [88.888893, 168.88889], [73.703705, 178.88889], [87.037041, 123.7037], [78.518517, 97.037041], [95.555557, 52.962959], [85.555557, 57.037041], [90.370369, 23.333332], [100.0, 28.51852], [88.888893, 37.037037], [87.037041, -42.962959], [89.259262, -24.814816], [93.333328, 7.4074073], [98.518517, 5.185185], [92.59259, 1.4814816], [85.925919, 153.7037], [95.555557, 154.44444], [92.962959, 150.0], [97.037041, 95.925919], [106.66667, 115.55556], [92.962959, 114.81482], [108.88889, 56.296295], [97.777779, 50.740742], [94.074081, 89.259262], [96.666672, 91.851852], [102.22222, 77.777779], [107.40741, 40.370369], [105.92592, 29.629629], [105.55556, -46.296295], [118.51852, -47.777779], [112.22222, -43.333336], [112.59259, 25.185184], [115.92592, 27.777777], [112.59259, 31.851852], [107.03704, -36.666668], [118.88889, -32.59259], [114.07408, -25.555555], [115.92592, 85.185181], [105.92592, 18.888889], [121.11111, 14.444445], [129.25926, -28.51852], [127.03704, -18.518518], [139.25926, -12.222222], [141.48149, 3.7037036], [137.03703, -4.814815], [153.7037, -26.666668], [-2.2222223, 5.5555558], [0.0, 9.6296301], [10.74074, 20.74074], [2.2222223, 54.074074], [4.0740738, 50.740742], [34.444443, 46.296295], [11.481482, 1.4814816], [24.074076, -2.9629631], [74.814819, 79.259254], [67.777779, 152.22223], [57.037041, 127.03704], [89.259262, 12.222222]]
points = np.array(points)
vor = Voronoi(points)
lines = [
LineString(vor.vertices[line])
for line in vor.ridge_vertices if -1 not in line
]
convex_hull = MultiPoint([Point(i) for i in points]).convex_hull.buffer(2)
result = MultiPolygon(
[poly.intersection(convex_hull) for poly in polygonize(lines)])
result = MultiPolygon(
[p for p in result]
+ [p for p in convex_hull.difference(unary_union(result))])
plt.plot(points[:,0], points[:,1], 'ko')
for r in result:
plt.fill(*zip(*np.array(list(
zip(r.boundary.coords.xy[0][:-1], r.boundary.coords.xy[1][:-1])))),
alpha=0.4)
plt.show()
Minus the small buffer on the convex hull, the result should look the same:

Or if you want a result which is slightly less "raw" on the exterior you can try to play with the buffer method (and its resolution
/join_style
/cap_style
properties) of your points (and/or the buffer of the convex hull):
pts = MultiPoint([Point(i) for i in points])
mask = pts.convex_hull.union(pts.buffer(10, resolution=5, cap_style=3))
result = MultiPolygon(
[poly.intersection(mask) for poly in polygonize(lines)])
And get something like (you can achieve better..!) :
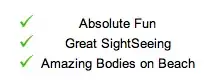