By now you may have found the Wikipedia page for the Centripetal Catmull-Rom spline, but in case you haven't, it includes this sample code:
import numpy
import matplotlib.pyplot as plt
def CatmullRomSpline(P0, P1, P2, P3, nPoints=100):
"""
P0, P1, P2, and P3 should be (x,y) point pairs that define the
Catmull-Rom spline.
nPoints is the number of points to include in this curve segment.
"""
# Convert the points to numpy so that we can do array multiplication
P0, P1, P2, P3 = map(numpy.array, [P0, P1, P2, P3])
# Calculate t0 to t4
alpha = 0.5
def tj(ti, Pi, Pj):
xi, yi = Pi
xj, yj = Pj
return ( ( (xj-xi)**2 + (yj-yi)**2 )**0.5 )**alpha + ti
t0 = 0
t1 = tj(t0, P0, P1)
t2 = tj(t1, P1, P2)
t3 = tj(t2, P2, P3)
# Only calculate points between P1 and P2
t = numpy.linspace(t1,t2,nPoints)
# Reshape so that we can multiply by the points P0 to P3
# and get a point for each value of t.
t = t.reshape(len(t),1)
A1 = (t1-t)/(t1-t0)*P0 + (t-t0)/(t1-t0)*P1
A2 = (t2-t)/(t2-t1)*P1 + (t-t1)/(t2-t1)*P2
A3 = (t3-t)/(t3-t2)*P2 + (t-t2)/(t3-t2)*P3
B1 = (t2-t)/(t2-t0)*A1 + (t-t0)/(t2-t0)*A2
B2 = (t3-t)/(t3-t1)*A2 + (t-t1)/(t3-t1)*A3
C = (t2-t)/(t2-t1)*B1 + (t-t1)/(t2-t1)*B2
return C
def CatmullRomChain(P):
"""
Calculate Catmull Rom for a chain of points and return the combined curve.
"""
sz = len(P)
# The curve C will contain an array of (x,y) points.
C = []
for i in range(sz-3):
c = CatmullRomSpline(P[i], P[i+1], P[i+2], P[i+3])
C.extend(c)
return C
which nicely computes the interpolation for n >= 4
points like so:
points = [[0,1.5],[2,2],[3,1],[4,0.5],[5,1],[6,2],[7,3]]
c = CatmullRomChain(points)
px, py = zip(*points)
x, y = zip(*c)
plt.plot(x, y)
plt.plot(px, py, 'or')
resulting in this matplotlib
image:

Update:
Alternatively, there is a scipy.interpolate
function for BarycentricInterpolator
that appears to do what you're looking for. It is rather straightforward to use and works for cases in which you have only 3 data points.
from scipy.interpolate import BarycentricInterpolator
# create some data points
points1 = [[0, 2], [1, 4], [2, -2], [3, 6], [4, 2]]
points2 = [[1, 1], [2, 5], [3, -1]]
# put data into x, y tuples
x1, y1 =zip(*points1)
x2, y2 = zip(*points2)
# create the interpolator
bci1 = BarycentricInterpolator(x1, y1)
bci2 = BarycentricInterpolator(x2, y2)
# define dense x-axis for interpolating over
x1_new = np.linspace(min(x1), max(x1), 1000)
x2_new = np.linspace(min(x2), max(x2), 1000)
# plot it all
plt.plot(x1, y1, 'o')
plt.plot(x2, y2, 'o')
plt.plot(x1_new, bci1(x1_new))
plt.plot(x2_new, bci2(x2_new))
plt.xlim(-1, 5)
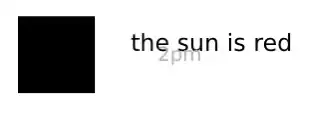
Update 2
Another option within scipy
is akima interpolation via Akima1DInterpolator
. It is as easy to implement as Barycentric, but has the advantage that it avoids large oscillations at the edge of a data set. Here's a few test cases that exhibit all the criteria you've asked for so far.
from scipy.interpolate import Akima1DInterpolator
x1, y1 = np.arange(13), np.random.randint(-10, 10, 13)
x2, y2 = [0,2,3,6,12], [100,50,30,18,14]
x3, y3 = [4, 6, 8], [60, 80, 40]
akima1 = Akima1DInterpolator(x1, y1)
akima2 = Akima1DInterpolator(x2, y2)
akima3 = Akima1DInterpolator(x3, y3)
x1_new = np.linspace(min(x1), max(x1), 1000)
x2_new = np.linspace(min(x2), max(x2), 1000)
x3_new = np.linspace(min(x3), max(x3), 1000)
plt.plot(x1, y1, 'bo')
plt.plot(x2, y2, 'go')
plt.plot(x3, y3, 'ro')
plt.plot(x1_new, akima1(x1_new), 'b', label='random points')
plt.plot(x2_new, akima2(x2_new), 'g', label='exponential')
plt.plot(x3_new, akima3(x3_new), 'r', label='3 points')
plt.xlim(-1, 15)
plt.ylim(-10, 110)
plt.legend(loc='best')
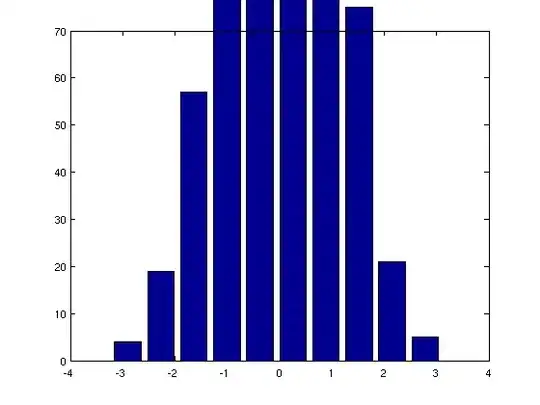