What you're describing isn't exactly image processing in the traditional sense, but it's fairly easy to do with numpy, etc.
Here's a rather large example doing some of the things you mentioned to get you pointed in the right direction... Note that the example images all show results for the origin at the center of the image, but the functions take an origin argument, so you should be able to directly adapt things for your purposes.
import numpy as np
import scipy as sp
import scipy.ndimage
import Image
import matplotlib.pyplot as plt
def main():
im = Image.open('mri_demo.png')
im = im.convert('RGB')
data = np.array(im)
plot_polar_image(data, origin=None)
plot_directional_intensity(data, origin=None)
plt.show()
def plot_directional_intensity(data, origin=None):
"""Makes a cicular histogram showing average intensity binned by direction
from "origin" for each band in "data" (a 3D numpy array). "origin" defaults
to the center of the image."""
def intensity_rose(theta, band, color):
theta, band = theta.flatten(), band.flatten()
intensities, theta_bins = bin_by(band, theta)
mean_intensity = map(np.mean, intensities)
width = np.diff(theta_bins)[0]
plt.bar(theta_bins, mean_intensity, width=width, color=color)
plt.xlabel(color + ' Band')
plt.yticks([])
# Make cartesian coordinates for the pixel indicies
# (The origin defaults to the center of the image)
x, y = index_coords(data, origin)
# Convert the pixel indices into polar coords.
r, theta = cart2polar(x, y)
# Unpack bands of the image
red, green, blue = data.T
# Plot...
plt.figure()
plt.subplot(2,2,1, projection='polar')
intensity_rose(theta, red, 'Red')
plt.subplot(2,2,2, projection='polar')
intensity_rose(theta, green, 'Green')
plt.subplot(2,1,2, projection='polar')
intensity_rose(theta, blue, 'Blue')
plt.suptitle('Average intensity as a function of direction')
def plot_polar_image(data, origin=None):
"""Plots an image reprojected into polar coordinages with the origin
at "origin" (a tuple of (x0, y0), defaults to the center of the image)"""
polar_grid, r, theta = reproject_image_into_polar(data, origin)
plt.figure()
plt.imshow(polar_grid, extent=(theta.min(), theta.max(), r.max(), r.min()))
plt.axis('auto')
plt.ylim(plt.ylim()[::-1])
plt.xlabel('Theta Coordinate (radians)')
plt.ylabel('R Coordinate (pixels)')
plt.title('Image in Polar Coordinates')
def index_coords(data, origin=None):
"""Creates x & y coords for the indicies in a numpy array "data".
"origin" defaults to the center of the image. Specify origin=(0,0)
to set the origin to the lower left corner of the image."""
ny, nx = data.shape[:2]
if origin is None:
origin_x, origin_y = nx // 2, ny // 2
else:
origin_x, origin_y = origin
x, y = np.meshgrid(np.arange(nx), np.arange(ny))
x -= origin_x
y -= origin_y
return x, y
def cart2polar(x, y):
r = np.sqrt(x**2 + y**2)
theta = np.arctan2(y, x)
return r, theta
def polar2cart(r, theta):
x = r * np.cos(theta)
y = r * np.sin(theta)
return x, y
def bin_by(x, y, nbins=30):
"""Bin x by y, given paired observations of x & y.
Returns the binned "x" values and the left edges of the bins."""
bins = np.linspace(y.min(), y.max(), nbins+1)
# To avoid extra bin for the max value
bins[-1] += 1
indicies = np.digitize(y, bins)
output = []
for i in xrange(1, len(bins)):
output.append(x[indicies==i])
# Just return the left edges of the bins
bins = bins[:-1]
return output, bins
def reproject_image_into_polar(data, origin=None):
"""Reprojects a 3D numpy array ("data") into a polar coordinate system.
"origin" is a tuple of (x0, y0) and defaults to the center of the image."""
ny, nx = data.shape[:2]
if origin is None:
origin = (nx//2, ny//2)
# Determine that the min and max r and theta coords will be...
x, y = index_coords(data, origin=origin)
r, theta = cart2polar(x, y)
# Make a regular (in polar space) grid based on the min and max r & theta
r_i = np.linspace(r.min(), r.max(), nx)
theta_i = np.linspace(theta.min(), theta.max(), ny)
theta_grid, r_grid = np.meshgrid(theta_i, r_i)
# Project the r and theta grid back into pixel coordinates
xi, yi = polar2cart(r_grid, theta_grid)
xi += origin[0] # We need to shift the origin back to
yi += origin[1] # back to the lower-left corner...
xi, yi = xi.flatten(), yi.flatten()
coords = np.vstack((xi, yi)) # (map_coordinates requires a 2xn array)
# Reproject each band individually and the restack
# (uses less memory than reprojection the 3-dimensional array in one step)
bands = []
for band in data.T:
zi = sp.ndimage.map_coordinates(band, coords, order=1)
bands.append(zi.reshape((nx, ny)))
output = np.dstack(bands)
return output, r_i, theta_i
if __name__ == '__main__':
main()
Original Image:
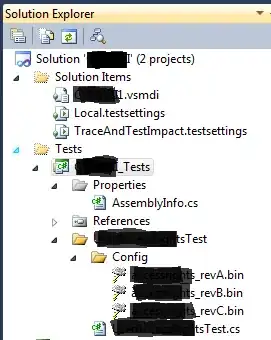
Projected into polar coordinates:
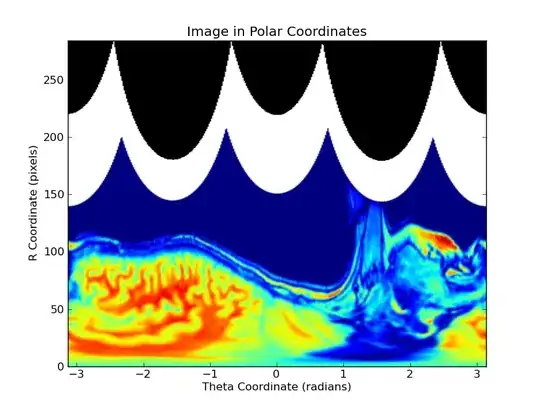
Intensity as a function of direction from the center of the image:
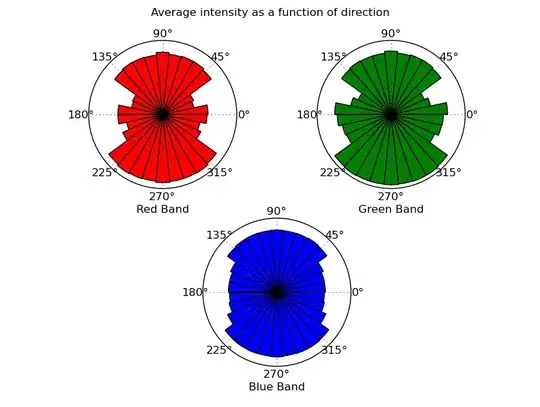