FOV of camera
Field of view of camera is absolute minimum to know to even start with this (how can you determine how to place object when you have no idea how it would affect scene). Basically you need transform matrix that maps from world GCS (global coordinate system) to Camera/Screen space and back. If you do not have a clue what about I am writing then perhaps you should not try any of this before you learn the math.
For unknown camera you can do some calibration based on markers or etalones (known size and shape) in the view. But much better is use real camera values (like FOV angles in x,y direction, focal length etc ...)
The goal for this is to create function that maps world GCS(x,y,z
) into Screen LCS(x,y
).
For more info read:
Silhouette matching
In order to compare rendered and real image similarity you need some kind of measure. As you need to match geometry I think silhouette matching is the way (ignoring textures, shadows and stuff).
So first you need to obtain silhouettes. Use image segmentation for that and create ROI mask of your object. For rendered image is this easy as you van render the object with single color without any lighting directly into ROI mask.
So you need to construct function that compute the difference between silhouettes. You can use any kind of measure but I think you should start with non overlapping areas pixel count (it is easy to compute).
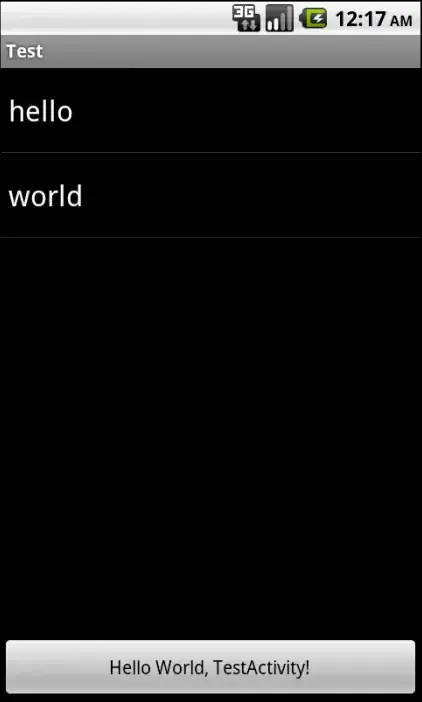
Basically you count pixels that are present only in one ROI (region of interest) mask.
estimate position
as you got the mesh then you know its size so place it in the GCS so rendered image has very close bounding box to real image. If you do not have FOV parameters then you need to rescale and translate each rendered image so it matches images bounding box (and as result you obtain only orientation not position of object of coarse). Cameras have perspective so the more far from camera you place your object the smaller it will be.
fit orientation
render few fixed orientations covering all orientations with some step 8^3
orientations. For each compute the difference of silhouette and chose orientation with smallest difference.
Then fit the orientation angles around it to minimize difference. If you do not know how optimization or fitting works see this:
Beware too small amount of initial orientations can cause false positioves or missed solutions. Too high amount will be slow.
Now that was some basics in a nutshell. As your mesh is not very simple you may need to tweak this like use contours instead of silhouettes and using distance between contours instead of non overlapping pixels count which is really hard to compute ... You should start with simpler meshes like dice , coin etc ... and when grasping all of this move to more complex shapes ...
[Edit1] algebraic approach
If you know some points in the image that coresponds to known 3D points (in your mesh) then you can along with the FOV of the camera used compute the transform matrix placing your object ...
if the transform matrix is M
(OpenGL style):
M = xx,yx,zx,ox
xy,yy,zy,oy
xz,yz,zz,oz
0, 0, 0, 1
Then any point from your mesh (x,y,z
) is transformed to global world (x',y',z')
like this:
(x',y',z') = M * (x,y,z)
The pixel position (x'',y''
) is done by camera FOV perspective projection like this:
y''=FOVy*(z'+focus)*y' + ys2;
x''=FOVx*(z'+focus)*x' + xs2;
where camera is at (0,0,-focus)
, projection plane is at z=0
and viewing direction is +z
so for any focal length focus
and screen resolution (xs,ys
):
xs2=xs*0.5;
ys2=ys*0.5;
FOVx=xs2/focus;
FOVy=ys2/focus;
When put all this together you obtain this:
xi'' = ( xx*xi + yx*yi + zx*zi + ox ) * ( xz*xi + yz*yi + zz*zi + ox + focus ) * FOVx
yi'' = ( xy*xi + yy*yi + zy*zi + oy ) * ( xz*xi + yz*yi + zz*zi + oy + focus ) * FOVy
where (xi,yi,zi
) is i-th
known point 3D position in mesh local coordinates and (xi'',yi''
) is corresponding known 2D pixel positions. So unknowns are the M
values:
{ xx,xy,xz,yx,yy,yx,zx,zy,zz,ox,oy,oz }
So we got 2 equations per each known point and 12 unknowns total. So you need to know 6 points. Solve the system of equations and construct your matrix M
.
Also you can exploit that M is a uniform orthogonal/orthonormal matrix so vectors
X = (xx,xy,xz)
Y = (yx,yy,yz)
Z = (zx,zy,zz)
Are perpendicular to each other so:
(X.Y) = (Y.Z) = (Z.X) = 0.0
Which can lower the number of needed points by introducing these to your system. Also you can exploit cross product so if you know 2 vectors the thirth can be computed
Z = (X x Y)*scale
So instead of 3 variables you need just single scale (which is 1 for orthonormal matrix). If I assume orthonormal matrix then:
|X| = |Y| = |Z| = 1
so we got 6 additional equations (3 x dot, and 3 for cross) without any additional unknowns so 3 point are indeed enough.