If you want a Monte Carlo simulation, you'll need to sample from the distribution a large number of times, not take a large sample one time.
Your object, points
, has values that increases as the index increases to a threshold around 400
, levels off, and then decreases. That's what plot(points ~ x)
shows. It may describe a distribution, but the actual distribution of values in points
is different. That shows how often values are within a certain range. You'll notice your x axis for the histogram is similar to the y axis for the plot(points ~ x)
plot. The actual distribution of values in the points
object is easy enough to see, and it is similar to what you're seeing when sampling 1000 values at random, without replacement from an object with 1900
values in it. Here's the distribution of values in points
(no simulation required):
hist(points, 100)
I used 100 breaks on purpose so you could see some of the fine details.
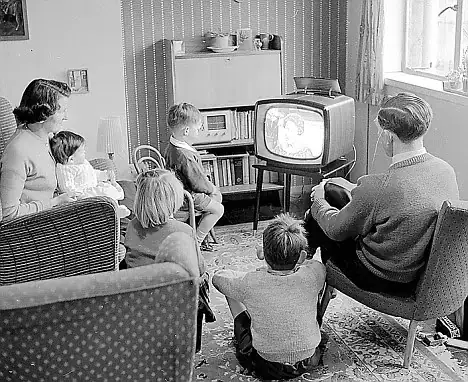
Notice the little bump in the tail at the top, that you may not be expecting if you want the histogram to look like the plot of the values vs. the index (or some increasing x). That means that there are more values in points
that are around 2
then there are around 1
. See if you can look at how the curve of plot(points ~ x)
flattens when the value is around 2
, and how it's very steep between 0.5
and 1.5
. Notice also the large hump at the low end of the histogram, and look at the plot(points ~ x)
curve again. Do you see how most of the values (whether they're at the low end or the high end of that curve) are close to 0
, or at least less than 0.25
. If you look at those details, you may be able to convince yourself that the histogram is, in fact, exactly what you should expect :)
If you want a Monte Carlo simulation of a sample from this object, you might try something like:
samples <- replicate(1000, sample(points, 100, replace = TRUE))
If you want to generate data using points
as a probability density function, that question has been asked and answered here