I have studied this issue a little bit more and it seems now to be best to create a new function for plotting radial cluster
directly from the linkage
output (rather than hacking the plotted one). I may cook up eventually something, but nothing very soon.
I'm assuming that your data naturally admit this kind of radial embedding. Have you verified that? Does there exists a suitable method in the linkage
for your purposes?
It seems that for any method linkage
will return a binary-tree structure. In your examples you have more general tree. You need some extra knowledge how to consolidate tree nodes. This all ready invalidates the idea of hacking the original dendrogram.
Update:
Would this naive example plot be a reasonable similar enough for your purposes? If so, I'll be able to post some really simple code to achieve it.
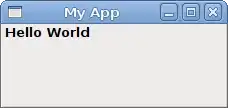
Update 2:
Here is the code:
radial_demo.py:
from numpy import r_, ones, pi, sort
from numpy.random import rand
from radial_grouper import tree, pre_order, post_order
from radial_visualizer import simple_link
from pylab import axis, figure, plot, subplot
# ToDo: create proper documentation
def _s(sp, t, o):
subplot(sp)
t.traverse(simple_link, order= o)
axis('equal')
def demo1(n):
p= r_[2* pi* rand(1, n)- pi, ones((1, n))]
t= tree(p)
f= figure()
_s(221, t, pre_order)
_s(222, t, post_order)
t= tree(p, tols= sort(2e0* rand(9)))
_s(223, t, pre_order)
_s(224, t, post_order)
f.show()
# f.savefig('test.png')
# ToDO: implement more demos
if __name__ == '__main__':
demo1(123)
radial_grouper.py:
"""All grouping functionality is collected here."""
from collections import namedtuple
from numpy import r_, arange, argsort, array, ones, pi, where
from numpy import logical_and as land
from radial_support import from_polar
__all__= ['tree', 'pre_order', 'post_order']
Node= namedtuple('Node', 'ndx lnk')
# ToDo: enhance documentation
def _groub_by(p, tol, r):
g, gm, gp= [], [], p- p[0]
while True:
if gp[-1]< 0: break
ndx= where(land(0.<= gp, gp< tol))[0]
if 0< len(ndx):
g.append(ndx)
gm.append(p[ndx].mean())
gp-= tol
return g, array([gm, [r]* len(gm)])
def _leafs(p):
return argsort(p[0])
def _create_leaf_nodes(ndx):
nodes= []
for k in xrange(len(ndx)):
nodes.append(Node(ndx[k], []))
return nodes
def _link_and_create_nodes(_n, n_, cn, groups):
nodes, n0= [], 0
for k in xrange(len(groups)):
nodes.append(Node(n_+ n0, [cn[m] for m in groups[k]]))
n0+= 1
return n_, n_+ n0, nodes
def _process_level(nodes, polar, p, tol, scale, _n, n_):
groups, p= _groub_by(p, tol, scale* polar[1, _n])
_n, n_, nodes= _link_and_create_nodes(_n, n_, nodes, groups)
polar[:, _n: n_]= p
return nodes, polar, _n, n_
def _create_tree(p, r0, scale, tols):
if None is tols:
tols= .3* pi/ 2** arange(5)[::-1]
_n, n_= 0, p.shape[1]
polar= ones((2, (len(tols)+ 2)* n_))
polar[0, :n_], polar[1, :n_]= p[0], r0
# leafs
nodes= _create_leaf_nodes(_leafs(p))
nodes, polar, _n, n_= _process_level(
nodes, polar, polar[0, _leafs(p)], tols[0], scale, _n, n_)
# links
for tol in tols[1:]:
nodes, polar, _n, n_= _process_level(
nodes, polar, polar[0, _n: n_], tol, scale, _n, n_)
# root
polar[:, n_]= [0., 0.]
return Node(n_, nodes), polar[:, :n_+ 1]
def _simplify(self):
# ToDo: combine single linkages
return self._root
def _call(self, node0, node1, f, level):
f(self, [node0.ndx, node1.ndx], level)
def pre_order(self, node0, f, level= 0):
for node1 in node0.lnk:
_call(self, node0, node1, f, level)
pre_order(self, node1, f, level+ 1)
def post_order(self, node0, f, level= 0):
for node1 in node0.lnk:
post_order(self, node1, f, level+ 1)
_call(self, node0, node1, f, level)
class tree(object):
def __init__(self, p, r0= pi, scale= .9, tols= None):
self._n= p.shape[1]
self._root, self._p= _create_tree(p, r0, scale, tols)
def traverse(self, f, order= pre_order, cs= 'Cartesian'):
self.points= self._p
if cs is 'Cartesian':
self.points= from_polar(self._p)
order(self, self._root, f, 0)
return self
def simplify(self):
self._root= _simplify(self)
return self
def is_root(self, ndx):
return ndx== self._p.shape[1]- 1
def is_leaf(self, ndx):
return ndx< self._n
if __name__ == '__main__':
# ToDO: add tests
from numpy import r_, round
from numpy.random import rand
from pylab import plot, show
def _l(t, n, l):
# print round(a, 3), n, l, t.is_root(n[0]), t.is_leaf(n[1])
plot(t.points[0, n], t.points[1, n])
if 0== l:
plot(t.points[0, n[0]], t.points[1, n[0]], 's')
if t.is_leaf(n[1]):
plot(t.points[0, n[1]], t.points[1, n[1]], 'o')
n= 123
p= r_[2* pi* rand(1, n)- pi, ones((1, n))]
t= tree(p).simplify().traverse(_l)
# t= tree(p).traverse(_l, cs= 'Polar')
show()
# print
# t.traverse(_l, post_order, cs= 'Polar')
radial_support.py:
"""All supporting functionality is collected here."""
from numpy import r_, arctan2, cos, sin
from numpy import atleast_2d as a2d
# ToDo: create proper documentation strings
def _a(a0, a1):
return r_[a2d(a0), a2d(a1)]
def from_polar(p):
"""(theta, radius) to (x, y)."""
return _a(cos(p[0])* p[1], sin(p[0])* p[1])
def to_polar(c):
"""(x, y) to (theta, radius)."""
return _a(arctan2(c[1], c[0]), (c** 2).sum(0)** .5)
def d_to_polar(D):
"""Distance matrix to (theta, radius)."""
# this functionality is to adopt for more general situations
# intended functionality:
# - embedd distance matrix to 2D
# - return that embedding in polar coordinates
pass
if __name__ == '__main__':
from numpy import allclose
from numpy.random import randn
c= randn(2, 5)
assert(allclose(c, from_polar(to_polar(c))))
# ToDO: implement more tests
radial_visualizer.py:
"""All visualization functionality is collected here."""
from pylab import plot
# ToDo: create proper documentation
def simple_link(t, ndx, level):
"""Simple_link is just a minimal example to demonstrate what can be
achieved when it's called from _grouper.tree.traverse for each link.
- t, tree instance
- ndx, a pair of (from, to) indicies
- level, of from, i.e. root is in level 0
"""
plot(t.points[0, ndx], t.points[1, ndx])
if 0== level:
plot(t.points[0, ndx[0]], t.points[1, ndx[0]], 's')
if t.is_leaf(ndx[1]):
plot(t.points[0, ndx[1]], t.points[1, ndx[1]], 'o')
# ToDO: implement more suitable link visualizers
# No doubt, this will the part to burn most of the dev. resources
if __name__ == '__main__':
# ToDO: implement tests
pass
You can find the source code here. Please feel free to modify it anyway you like, but please keep the future modifications synced with the gist.