An Empirical Approach.
Let's implement the erroneous algorithm in Mathematica:
p = 10; (* Range *)
s = {}
For[l = 1, l <= 30000, l++, (*Iterations*)
a = Range[p];
For[k = 1, k <= p, k++,
i = RandomInteger[{1, p}];
temp = a[[k]];
a[[k]] = a[[i]];
a[[i]] = temp
];
AppendTo[s, a];
]
Now get the number of times each integer is in each position:
r = SortBy[#, #[[1]] &] & /@ Tally /@ Transpose[s]
Let's take three positions in the resulting arrays and plot the frequency distribution for each integer in that position:
For position 1 the freq distribution is:
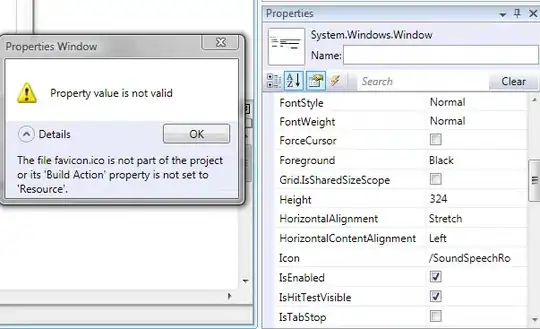
For position 5 (middle)

And for position 10 (last):
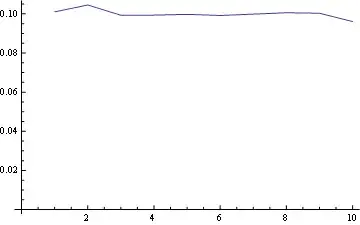
and here you have the distribution for all positions plotted together:
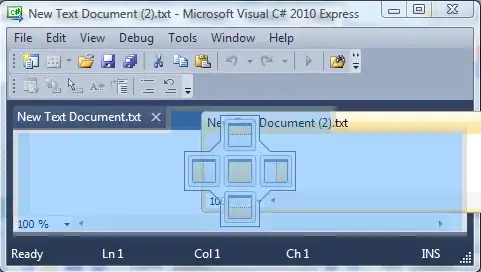
Here you have a better statistics over 8 positions:
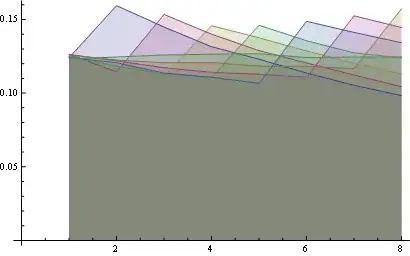
Some observations:
- For all positions the probability of
"1" is the same (1/n).
- The probability matrix is symmetrical
with respect to the big anti-diagonal
- So, the probability for any number in the last
position is also uniform (1/n)
You may visualize those properties looking at the starting of all lines from the same point (first property) and the last horizontal line (third property).
The second property can be seen from the following matrix representation example, where the rows are the positions, the columns are the occupant number, and the color represents the experimental probability:
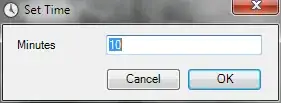
For a 100x100 matrix:
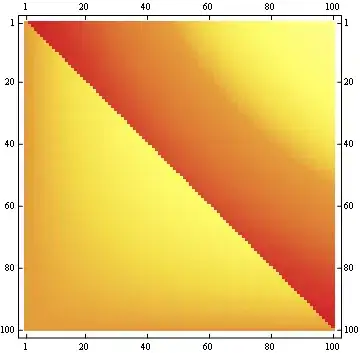
Edit
Just for fun, I calculated the exact formula for the second diagonal element (the first is 1/n). The rest can be done, but it's a lot of work.
h[n_] := (n-1)/n^2 + (n-1)^(n-2) n^(-n)
Values verified from n=3 to 6 ( {8/27, 57/256, 564/3125, 7105/46656} )
Edit
Working out a little the general explicit calculation in @wnoise answer, we can get a little more info.
Replacing 1/n by p[n], so the calculations are hold unevaluated, we get for example for the first part of the matrix with n=7 (click to see a bigger image):
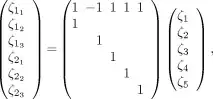
Which, after comparing with results for other values of n, let us identify some known integer sequences in the matrix:
{{ 1/n, 1/n , ...},
{... .., A007318, ....},
{... .., ... ..., ..},
... ....,
{A129687, ... ... ... ... ... ... ..},
{A131084, A028326 ... ... ... ... ..},
{A028326, A131084 , A129687 ... ....}}
You may find those sequences (in some cases with different signs) in the wonderful http://oeis.org/
Solving the general problem is more difficult, but I hope this is a start