The general question of "line simplification" seems to be an entire field of research. I recommend you to have a look, for instance, at the Ramer–Douglas–Peucker algorithm. There are several python modules, I could find: rdp and simplification (which also implement the Py-Visvalingam-Whyatt algorithm).
Anyway, I am trying something for evaluating the difference between two polylines, using interpolation. Any curves can be compared, even without common points.
The first idea is to compute the distance along the path for both polylines. They are used as landmarks to go from one given point on the first curve to a relatively close point on the other curve.
Then, the points of the first curve can be interpolated on the other curve. These two datasets can now be compared, point by point.
On the graph, the black curve is the interpolation of xy2 on the curve xy1. So the distances between the black squares and the orange circles can be computed, and averaged.
This gives an average distance measure, but nothing to compare against and decide if the applied reduction is good enough...
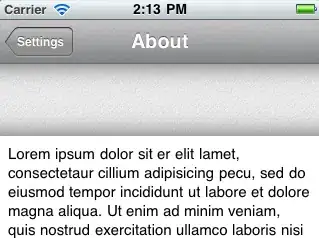
def normed_distance_along_path( polyline ):
polyline = np.asarray(polyline)
distance = np.cumsum( np.sqrt(np.sum( np.diff(polyline, axis=1)**2, axis=0 )) )
return np.insert(distance, 0, 0)/distance[-1]
def average_distance_between_polylines(xy1, xy2):
s1 = normed_distance_along_path(xy1)
s2 = normed_distance_along_path(xy2)
interpol_xy1 = interp1d( s1, xy1 )
xy1_on_2 = interpol_xy1(s2)
node_to_node_distance = np.sqrt(np.sum( (xy1_on_2 - xy2)**2, axis=0 ))
return node_to_node_distance.mean() # or use the max
# Two example polyline:
xy1 = [0, 1, 8, 2, 1.7], [1, 0, 6, 7, 1.9] # it should work in 3D too
xy2 = [.1, .6, 4, 8.3, 2.1, 2.2, 2], [.8, .1, 2, 6.4, 6.7, 4.4, 2.3]
average_distance_between_polylines(xy1, xy2) # 0.45004578069119189