Here is a solution where you only use networkx to create your multi-layer graph, and where you calculate the node positions by your own.
For the purpose of this explanation, we create a small graph of 30 nodes randomly.
The layers are sub-graphs with additional properties: (x, y) coordinates and a color.
The coordinates are used to position the layers relatively in a 2D grid.
import networkx as nx
# create a random graph of 30 nodes
graph = nx.fast_gnp_random_graph(30, .2, seed=2019)
# Layers have coordinates and colors
layers = [
(nx.Graph(), (0, 0), "#ffaaaa"),
(nx.Graph(), (0, 1), "#aaffaa"),
(nx.Graph(), (0, 2), "#aaaaff"),
(nx.Graph(), (1, 2), "#ffa500"),
]
Each layer is populated with the nodes of the main graph.
Here, we decided to split the nodes list in various ranges (the start and end index of the nodes in the graph).
The color of each node is stored in a color_map.
This variable is used later during graph plotting.
import itertools
# node ranges in the graph
ranges = [(0, 6), (6, 15), (15, 20), (20, 30)]
# fill the layers with nodes from the graph
# prepare the color map
color_map = []
for (layer, coord, color), (start, end) in zip(layers, ranges):
layer.add_nodes_from(itertools.islice(graph.nodes, start, end))
color_map.extend([color for _ in range(start, end)])
Then, we can calculate the position of each node.
The node position is shifted according to the layer coordinates.
# Calculate and move the nodes position
all_pos = {}
for layer, (sx, sy), color in layers:
pos = nx.circular_layout(layer, scale=2) # or spring_layout...
for node in pos:
all_pos[node] = pos[node]
all_pos[node] += (10 * sx, 10 * sy)
We can now draw the graph:
import matplotlib.pyplot as plt
# Draw and display the graph
nx.draw(graph, all_pos, node_size=500, node_color=color_map, with_labels=True)
plt.show()
The result looks like this:
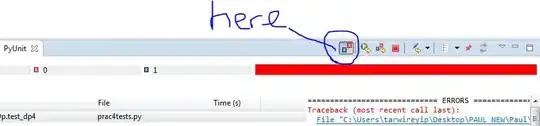
Of course, you can work with a 3D-grid and use a projection to have a 3D-like preview.