The idea is to threshold the image to obtain a binary image then find contours and sort using the contour area. The largest contour should be the index card. We can then apply a four point perspective transform to obtain a birds-eye view of the image. Here are the results:
Binary image
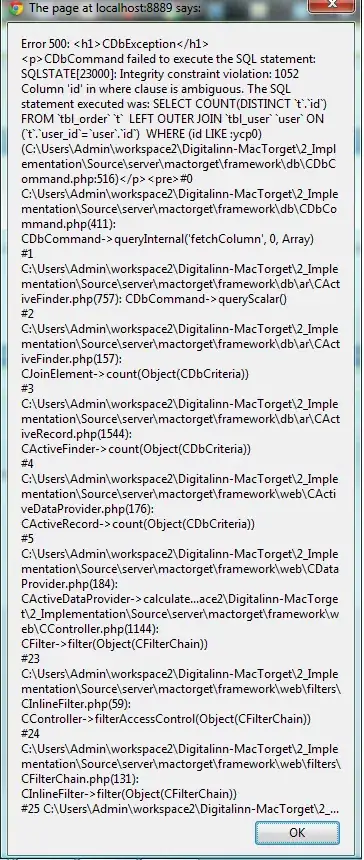
Result
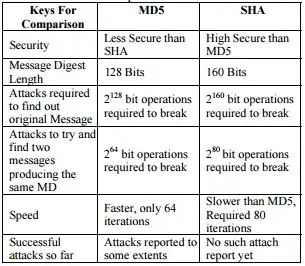
The result is dark so to increase the contrast look at Automatic contrast and brightness adjustment of a color photo of a sheet of paper with OpenCV. Also it's slightly skewed so you should perform skew correction. Take a look at Python OpenCV skew correction, How to de-skew an image, and Detect image orientation angle based on text direction
I'll leave these steps to you :)
Code
from imutils.perspective import four_point_transform
import cv2
import numpy
# Load image, grayscale, Gaussian blur, Otsu's threshold
image = cv2.imread("1.jpg")
gray = cv2.cvtColor(image, cv2.COLOR_BGR2GRAY)
blur = cv2.GaussianBlur(gray, (5,5), 0)
thresh = cv2.threshold(blur, 0, 255, cv2.THRESH_BINARY + cv2.THRESH_OTSU)[1]
# Find contours and sort for largest contour
cnts = cv2.findContours(thresh, cv2.RETR_EXTERNAL,cv2.CHAIN_APPROX_SIMPLE)
cnts = cnts[0] if len(cnts) == 2 else cnts[1]
cnts = sorted(cnts, key=cv2.contourArea, reverse=True)
displayCnt = None
for c in cnts:
# Perform contour approximation
peri = cv2.arcLength(c, True)
approx = cv2.approxPolyDP(c, 0.02 * peri, True)
if len(approx) == 4:
displayCnt = approx
break
# Obtain birds' eye view of image
warped = four_point_transform(image, displayCnt.reshape(4, 2))
cv2.imshow("thresh", thresh)
cv2.imshow("warped", warped)
cv2.imshow("image", image)
cv2.imwrite("thresh.png", thresh)
cv2.imwrite("warped.png", warped)
cv2.imwrite("image.png", image)
cv2.waitKey()