Q :
"... much slower then usual serial code with loops.
Could anyone help with issue?"
A :
Yes, we could help - first, let's agree what the issue is.
Tl;Dr; but important ...
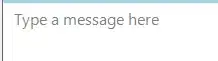
We will work using reproducible & evidence-based argumentation.
WORK PLAN - where is the PERFORMANCE gained or lost :
- measure the pure-
[SERIAL]
code as-is
- measure the pure-
[SERIAL]
code after performance was tuned-up
- measure the pure-
[SERIAL]
code once called using multiprocessing
- as-is code took
22,704,803 [us]
~ 22,7 sec ( ~ 5,375 sec on your localhost )
>>> from zmq import Stopwatch; aClk = Stopwatch() # a [us]-granular clock
>>>
>>> aClk.start(); _ = [ _.append( howmany_within_range( row,
... minimum = 4,
... maximum = 8 )
... ) for row in data ]; aClk.stop()
22704803 [us]
- improved code
1,877,015 [us]
~ 1.88 sec numpy-code ~ 12x faster & so will be on your localhost
Python Interpreter is known, since ever, to be slow on looping.
We can do better instead, the 1E7
-large, outer, list
-iterator based loop is a very frequent school-book example anti-pattern, the row
-wise internal loop inside the how_many_within_range()
is twice as bad anti-pattern ( besides calling 1E7
-times the call-signature processing (passing data + parameters' decoding overheads), the row-wise for-loop iterator is, again, a slo-mo syntax constructor, here repeated 1E7
-times - nothing to joy or celebrate, perhaps the book author was enthusiastic on efficiency devastation code practices - in that and only that case a performance devastator badge ought be awarded for such a few-SLOC performance anti-pattern ).
If we take time to understand what the code as-is actually calculates, we can right here improve the performance 12x better just by not losing a bit of time for non-productive steps.
>>> from zmq import Stopwatch; aClk = Stopwatch() # a [us]-granular clock
>>>
>>> aClk.start(); _ = np.where( ( arr >= 4 )*( arr <= 8 ),# if condition met
... 1, # set cell 1
... 0 # else 0
... ).sum( axis = 1 # .sum() row-wise
... ); aClk.stop()
1877015 [us]
- measure the pure-
[SERIAL]
code once called using multiprocessing
If one has successfully understood the step 2), the step 3) is many times worse due to another factor - introduced by "upgrading" the already awfully bad mix of triple-inefficient iterator-driven looping seen above to a next, many orders of magnitude less efficient, level of inefficiency - as we will have to be paying 1E7
-times (perhaps unexpectedly) expensive costs of passing (here at least small in RAM-size & easy to process, in serialisation-complexity terms) a set of parameters towards the "remote"-worker processes, each time a call-signature was called to do so.
1E7
-times ... (!)
That happens at a cost of a sum of add-on [TimeDOMAIN]
and [SpaceDOMAIN]
costs of:
- SER: a
pickle.dumps()
-SER-ialiser RAM-allocate/RAM-copy/CPU-process
- XFER: acquiring and using the O/S-tools for process-to-process communication ( pipe on POSIX compliant O/S, or even spending extra costs on building & using protocol-stack enriched TCP-emulated pipe on other O/S )
- DES: a
pickle.loads()
-DES-erialiser RAM-allocate/RAM-copy/CPU-process to decode the set of parameters received from XFER inside the "remote"-process
The costs of doing it this way are those that make up those about 5~22 seconds grow into observed minutes of slowed-down processing (awfully inefficient by all, not only these SER/XFER/DES, non-productive costs added - for the process-instantiation add-on overhead costs you may read raw details in the figure above or read the full story )
Last but not least, if that many [GB]
got copied ( at a one-stop add-on cost ), the inefficiency problem does not stop here - see the Virtual Memory [MB] details above - as on systems with not so large physical-RAM ( easily getting into the [TB]-scales gigantic footprints ), the O/S starts operating a so called RAM-to-DISK swapping, so as to emulate as if there were so much RAM ( now, moving [GB]-blocks from ~ 300 [ns]
RAM-storage, into a super-slower ~ 10,000,000 [ns]
DISK-storage ( all through a bottleneck of a few physical-RAM memory-I/O channels - imagine a Formule 1 racing ring, all running above 200 mph, suddenly having to cross the Potomac river, using but a pair of steam-engine ferry-boats ... puff-puff-puff ... going in there own pace there and back, there and back, each time carrying not more than a few racing cars (DATA) -- that slow are data transfers during the RAM-to-DISK swapping emulations.
It does not straight crash the O/S - fine, but indeed no big racing since this starts in the middle of the work ... This is why it is often called RAM-thrashing ... so each new multiprocessing
-spawned process moves you closer to this irreversible performance disaster (being a full copy of the Python Interpreter process - even if some folks keep saying here a fork
-backend is possible not to do so large copy, actually it is not, as many O/S-es cannot fork
at all, and most others claim insecure or even self-deadlocking side-effects if not using spawn
-backend for indeed spawning a full, as stateful as possible copy of the main-Python Interpreter process (plus add to this that some problems even with this top-down Python Interpreter process full-copy statefulness ambition still remain in 2022-Q2, so rather be even more careful on this )