Here is one way to do that in Python/OpenCV.
- Read the input
- Convert to gray
- Threshold to binary
- Get the contours and filter on area so that we have only the two primary lines
- Sort by area
- Select the first (smaller and thinner) contour
- Draw it white filled on a black background
- Get its skeleton
- Get the points of the skeleton
- Fit a line to the points and get the rotation angle of the skeleton
- Loop over each of the two contours and draw them white filled on a black background. Then rotate to horizontal lines. Then get the vertical thickness of the lines from the average thickness along each column using np.count_nonzero() and print the value.
- Save intermediate images
Input:

import cv2
import numpy as np
import skimage.morphology
import skimage.transform
import math
# read image
img = cv2.imread('lines.jpg')
# convert to grayscale
gray = cv2.cvtColor(img,cv2.COLOR_BGR2GRAY)
# threshold
thresh = cv2.threshold(gray, 0, 255, cv2.THRESH_BINARY+cv2.THRESH_OTSU)[1]
# get contours
new_contours = []
img2 = np.zeros_like(thresh, dtype=np.uint8)
contour_img = thresh.copy()
contour_img = cv2.merge([contour_img,contour_img,contour_img])
contours = cv2.findContours(thresh , cv2.RETR_EXTERNAL, cv2.CHAIN_APPROX_SIMPLE)
contours = contours[0] if len(contours) == 2 else contours[1]
for cntr in contours:
area = cv2.contourArea(cntr)
if area > 1000:
cv2.drawContours(contour_img, [cntr], 0, (0,0,255), 1)
cv2.drawContours(img2, [cntr], 0, (255), -1)
new_contours.append(cntr)
# sort contours by area
cnts_sort = sorted(new_contours, key=lambda x: cv2.contourArea(x), reverse=False)
# select first (smaller) sorted contour
first_contour = cnts_sort[0]
contour_first_img = np.zeros_like(thresh, dtype=np.uint8)
cv2.drawContours(contour_first_img, [first_contour], 0, (255), -1)
# thin smaller contour
thresh1 = (contour_first_img/255).astype(np.float64)
skeleton = skimage.morphology.skeletonize(thresh1)
skeleton = (255*skeleton).clip(0,255).astype(np.uint8)
# get skeleton points
pts = np.column_stack(np.where(skeleton.transpose()==255))
# fit line to pts
(vx,vy,x,y) = cv2.fitLine(pts, cv2.DIST_L2, 0, 0.01, 0.01)
#print(vx,vy,x,y)
x_axis = np.array([1, 0]) # unit vector in the same direction as the x axis
line_direction = np.array([vx, vy]) # unit vector in the same direction as your line
dot_product = np.dot(x_axis, line_direction)
[angle_line] = (180/math.pi)*np.arccos(dot_product)
print("angle:", angle_line)
# loop over each sorted contour
# draw contour filled on black background
# rotate
# get mean thickness from np.count_non-zeros
black = np.zeros_like(thresh, dtype=np.uint8)
i = 1
for cnt in cnts_sort:
cnt_img = black.copy()
cv2.drawContours(cnt_img, [cnt], 0, (255), -1)
cnt_img_rot = skimage.transform.rotate(cnt_img, angle_line, resize=False)
thickness = np.mean(np.count_nonzero(cnt_img_rot, axis=0))
print("line ",i,"=",thickness)
i = i + 1
# save resulting images
cv2.imwrite('lines_thresh.jpg',thresh)
cv2.imwrite('lines_filtered.jpg',img2)
cv2.imwrite('lines_small_contour_skeleton.jpg',skeleton )
# show thresh and result
cv2.imshow("thresh", thresh)
cv2.imshow("contours", contour_img)
cv2.imshow("lines_filtered", img2)
cv2.imshow("first_contour", contour_first_img)
cv2.imshow("skeleton", skeleton)
cv2.waitKey(0)
cv2.destroyAllWindows()
Threshold image:
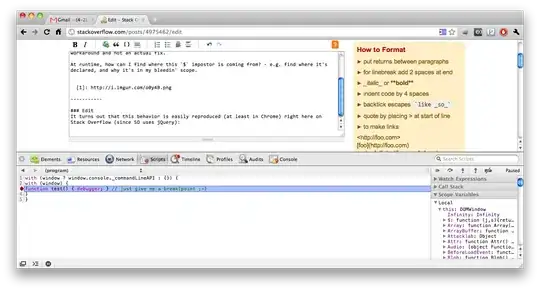
Contour image:
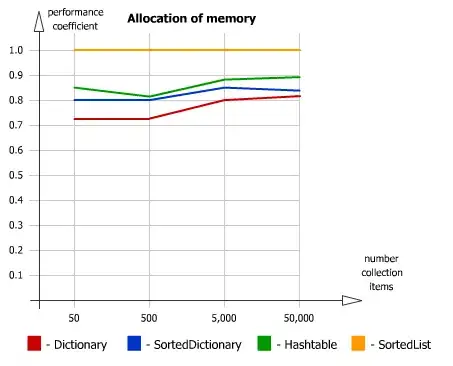
Filtered contour image:
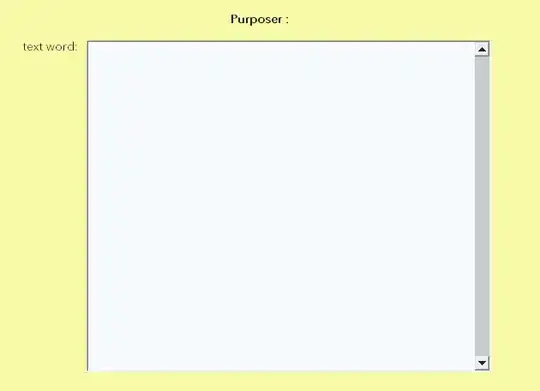
Skeleton image:
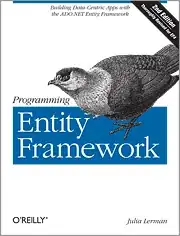
Angle (in degrees) and Thicknesses (in pixels):
angle: 3.1869032185349733
line 1 = 8.79219512195122
line 2 = 49.51609756097561
To get the thickness in nm, multiply thickness in pixels by your 314 nm/pixel.
ADDITION
If I start with your tiff image, the following shows my preprocessing, which is similar to yours.
import cv2
import numpy as np
import skimage.morphology
import skimage.transform
import math
# read image
img = cv2.imread('lines.tif')
# convert to grayscale
gray = cv2.cvtColor(img,cv2.COLOR_BGR2GRAY)
# threshold
thresh = cv2.threshold(gray, 128, 255, cv2.THRESH_BINARY)[1]
# apply morphology
kernel = cv2.getStructuringElement(cv2.MORPH_RECT, (1,5))
morph = cv2.morphologyEx(thresh, cv2.MORPH_OPEN, kernel)
kernel = cv2.getStructuringElement(cv2.MORPH_RECT, (29,1))
morph = cv2.morphologyEx(morph, cv2.MORPH_CLOSE, kernel)
# get contours
new_contours = []
img2 = np.zeros_like(gray, dtype=np.uint8)
contour_img = gray.copy()
contour_img = cv2.merge([contour_img,contour_img,contour_img])
contours = cv2.findContours(morph , cv2.RETR_EXTERNAL, cv2.CHAIN_APPROX_SIMPLE)
contours = contours[0] if len(contours) == 2 else contours[1]
for cntr in contours:
area = cv2.contourArea(cntr)
if area > 1000:
cv2.drawContours(contour_img, [cntr], 0, (0,0,255), 1)
cv2.drawContours(img2, [cntr], 0, (255), -1)
new_contours.append(cntr)
# sort contours by area
cnts_sort = sorted(new_contours, key=lambda x: cv2.contourArea(x), reverse=False)
# select first (smaller) sorted contour
first_contour = cnts_sort[0]
contour_first_img = np.zeros_like(morph, dtype=np.uint8)
cv2.drawContours(contour_first_img, [first_contour], 0, (255), -1)
# thin smaller contour
thresh1 = (contour_first_img/255).astype(np.float64)
skeleton = skimage.morphology.skeletonize(thresh1)
skeleton = (255*skeleton).clip(0,255).astype(np.uint8)
# get skeleton points
pts = np.column_stack(np.where(skeleton.transpose()==255))
# fit line to pts
(vx,vy,x,y) = cv2.fitLine(pts, cv2.DIST_L2, 0, 0.01, 0.01)
#print(vx,vy,x,y)
x_axis = np.array([1, 0]) # unit vector in the same direction as the x axis
line_direction = np.array([vx, vy]) # unit vector in the same direction as your line
dot_product = np.dot(x_axis, line_direction)
[angle_line] = (180/math.pi)*np.arccos(dot_product)
print("angle:", angle_line)
# loop over each sorted contour
# draw contour filled on black background
# rotate
# get mean thickness from np.count_non-zeros
black = np.zeros_like(thresh, dtype=np.uint8)
i = 1
for cnt in cnts_sort:
cnt_img = black.copy()
cv2.drawContours(cnt_img, [cnt], 0, (255), -1)
cnt_img_rot = skimage.transform.rotate(cnt_img, angle_line, resize=False)
thickness = np.mean(np.count_nonzero(cnt_img_rot, axis=0))
print("line ",i,"=",thickness)
i = i + 1
# save resulting images
cv2.imwrite('lines_thresh2.jpg',thresh)
cv2.imwrite('lines_morph2.jpg',morph)
cv2.imwrite('lines_filtered2.jpg',img2)
cv2.imwrite('lines_small_contour_skeleton2.jpg',skeleton )
# show thresh and result
cv2.imshow("thresh", thresh)
cv2.imshow("morph", morph)
cv2.imshow("contours", contour_img)
cv2.imshow("lines_filtered", img2)
cv2.imshow("first_contour", contour_first_img)
cv2.imshow("skeleton", skeleton)
cv2.waitKey(0)
cv2.destroyAllWindows()
Threshold image:
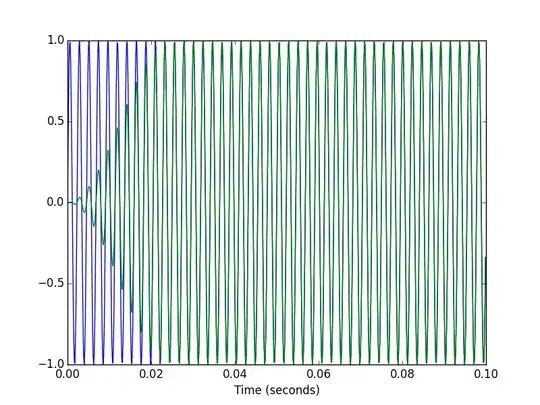
Morphology image:
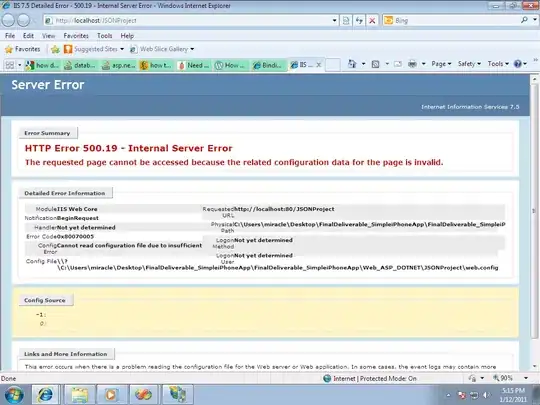
Filtered Lines image:
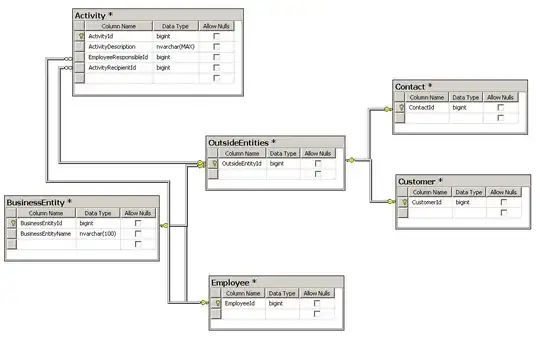
Skeleton image:
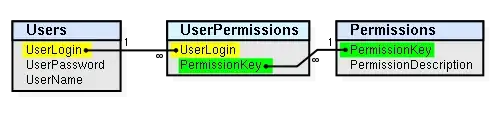
Angle (degrees) and Thickness (pixels):
angle: 3.206927978669998
line 1 = 9.26171875
line 2 = 49.693359375