One possible algorithm to clean up the images is as follows:
- Scale the image up, so that the letters are more substantial.
- Reduce the image to only 8 colours by k-means clustering.
- Threshold the image, and erode it to fill in any small gaps and make the letters more substantial.
- Invert the image to make masking easier.
- Create a blank mask image of the same size, set to all zeros
- Find contours in the image. For each contour:
- Find bounding box of the contour
- Find the area of the bounding box
- If the area is too small or too large, drop the contour (I chose 1000 and 10000 as limits)
- Otherwise draw a filled rectangle corresponding to the bounding box on the mask with white colour (255)
- Store the bounding box and the corresponding image ROI
- For each separated character (bounding box + image)
Note: I prototyped this in Python 2.7 with OpenCV 3.1. C++ ports of this code are near the end of this answer.
Character Recognition
I took inspiration for the character recognition from this question on SO.
Then I found an image that we can use to extract training images for the correct font. I cut them down to only include digits and letters without accents.
train_digits.png
:

train_letters.png
:
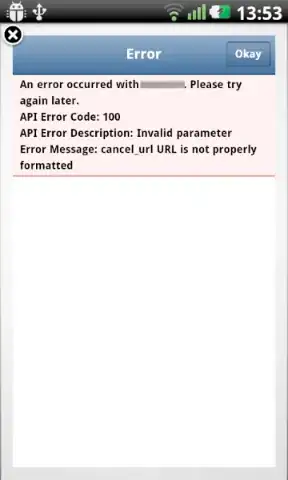
Then i wrote a script that splits the individual characters, scales them up and prepares the training images that contain single character per file:
import os
import cv2
import numpy as np
# ============================================================================
def extract_chars(img):
bw_image = cv2.bitwise_not(img)
contours = cv2.findContours(bw_image, cv2.RETR_EXTERNAL, cv2.CHAIN_APPROX_NONE)[1]
char_mask = np.zeros_like(img)
bounding_boxes = []
for contour in contours:
x,y,w,h = cv2.boundingRect(contour)
x,y,w,h = x-2, y-2, w+4, h+4
bounding_boxes.append((x,y,w,h))
characters = []
for bbox in bounding_boxes:
x,y,w,h = bbox
char_image = img[y:y+h,x:x+w]
characters.append(char_image)
return characters
# ============================================================================
def output_chars(chars, labels):
for i, char in enumerate(chars):
filename = "chars/%s.png" % labels[i]
char = cv2.resize(char
, None
, fx=3
, fy=3
, interpolation=cv2.INTER_CUBIC)
cv2.imwrite(filename, char)
# ============================================================================
if not os.path.exists("chars"):
os.makedirs("chars")
img_digits = cv2.imread("train_digits.png", 0)
img_letters = cv2.imread("train_letters.png", 0)
digits = extract_chars(img_digits)
letters = extract_chars(img_letters)
DIGITS = [0, 9, 8 ,7, 6, 5, 4, 3, 2, 1]
LETTERS = [chr(ord('A') + i) for i in range(25,-1,-1)]
output_chars(digits, DIGITS)
output_chars(letters, LETTERS)
# ============================================================================
The next step was to generate the training data from the character files we created with the previous script.
I followed the algorithm from the answer to the question mentioned above, resizing each character image to 10x10 and using all the pixels as keypoints.
I save the training data as char_samples.data
and char_responses.data
Script to generate training data:
import cv2
import numpy as np
CHARS = [chr(ord('0') + i) for i in range(10)] + [chr(ord('A') + i) for i in range(26)]
# ============================================================================
def load_char_images():
characters = {}
for char in CHARS:
char_img = cv2.imread("chars/%s.png" % char, 0)
characters[char] = char_img
return characters
# ============================================================================
characters = load_char_images()
samples = np.empty((0,100))
for char in CHARS:
char_img = characters[char]
small_char = cv2.resize(char_img,(10,10))
sample = small_char.reshape((1,100))
samples = np.append(samples,sample,0)
responses = np.array([ord(c) for c in CHARS],np.float32)
responses = responses.reshape((responses.size,1))
np.savetxt('char_samples.data',samples)
np.savetxt('char_responses.data',responses)
# ============================================================================
Once we have the training data created, we can run the main script:
import cv2
import numpy as np
# ============================================================================
def reduce_colors(img, n):
Z = img.reshape((-1,3))
# convert to np.float32
Z = np.float32(Z)
# define criteria, number of clusters(K) and apply kmeans()
criteria = (cv2.TERM_CRITERIA_EPS + cv2.TERM_CRITERIA_MAX_ITER, 10, 1.0)
K = n
ret,label,center=cv2.kmeans(Z,K,None,criteria,10,cv2.KMEANS_RANDOM_CENTERS)
# Now convert back into uint8, and make original image
center = np.uint8(center)
res = center[label.flatten()]
res2 = res.reshape((img.shape))
return res2
# ============================================================================
def clean_image(img):
gray_img = cv2.cvtColor(img, cv2.COLOR_BGR2GRAY)
resized_img = cv2.resize(gray_img
, None
, fx=5.0
, fy=5.0
, interpolation=cv2.INTER_CUBIC)
resized_img = cv2.GaussianBlur(resized_img,(5,5),0)
cv2.imwrite('licence_plate_large.png', resized_img)
equalized_img = cv2.equalizeHist(resized_img)
cv2.imwrite('licence_plate_equ.png', equalized_img)
reduced = cv2.cvtColor(reduce_colors(cv2.cvtColor(equalized_img, cv2.COLOR_GRAY2BGR), 8), cv2.COLOR_BGR2GRAY)
cv2.imwrite('licence_plate_red.png', reduced)
ret, mask = cv2.threshold(reduced, 64, 255, cv2.THRESH_BINARY)
cv2.imwrite('licence_plate_mask.png', mask)
kernel = cv2.getStructuringElement(cv2.MORPH_RECT, (3, 3))
mask = cv2.erode(mask, kernel, iterations = 1)
cv2.imwrite('licence_plate_mask2.png', mask)
return mask
# ============================================================================
def extract_characters(img):
bw_image = cv2.bitwise_not(img)
contours = cv2.findContours(bw_image, cv2.RETR_TREE, cv2.CHAIN_APPROX_NONE)[1]
char_mask = np.zeros_like(img)
bounding_boxes = []
for contour in contours:
x,y,w,h = cv2.boundingRect(contour)
area = w * h
center = (x + w/2, y + h/2)
if (area > 1000) and (area < 10000):
x,y,w,h = x-4, y-4, w+8, h+8
bounding_boxes.append((center, (x,y,w,h)))
cv2.rectangle(char_mask,(x,y),(x+w,y+h),255,-1)
cv2.imwrite('licence_plate_mask3.png', char_mask)
clean = cv2.bitwise_not(cv2.bitwise_and(char_mask, char_mask, mask = bw_image))
bounding_boxes = sorted(bounding_boxes, key=lambda item: item[0][0])
characters = []
for center, bbox in bounding_boxes:
x,y,w,h = bbox
char_image = clean[y:y+h,x:x+w]
characters.append((bbox, char_image))
return clean, characters
def highlight_characters(img, chars):
output_img = cv2.cvtColor(img, cv2.COLOR_GRAY2BGR)
for bbox, char_img in chars:
x,y,w,h = bbox
cv2.rectangle(output_img,(x,y),(x+w,y+h),255,1)
return output_img
# ============================================================================
img = cv2.imread("licence_plate.jpg")
img = clean_image(img)
clean_img, chars = extract_characters(img)
output_img = highlight_characters(clean_img, chars)
cv2.imwrite('licence_plate_out.png', output_img)
samples = np.loadtxt('char_samples.data',np.float32)
responses = np.loadtxt('char_responses.data',np.float32)
responses = responses.reshape((responses.size,1))
model = cv2.ml.KNearest_create()
model.train(samples, cv2.ml.ROW_SAMPLE, responses)
plate_chars = ""
for bbox, char_img in chars:
small_img = cv2.resize(char_img,(10,10))
small_img = small_img.reshape((1,100))
small_img = np.float32(small_img)
retval, results, neigh_resp, dists = model.findNearest(small_img, k = 1)
plate_chars += str(chr((results[0][0])))
print("Licence plate: %s" % plate_chars)
Script Output
Enlarged 5x:
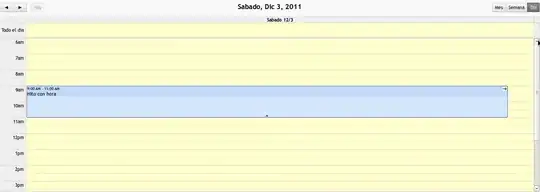
Equalized:
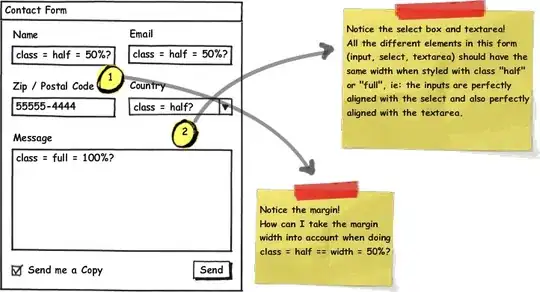
Reduced to 8 colours:
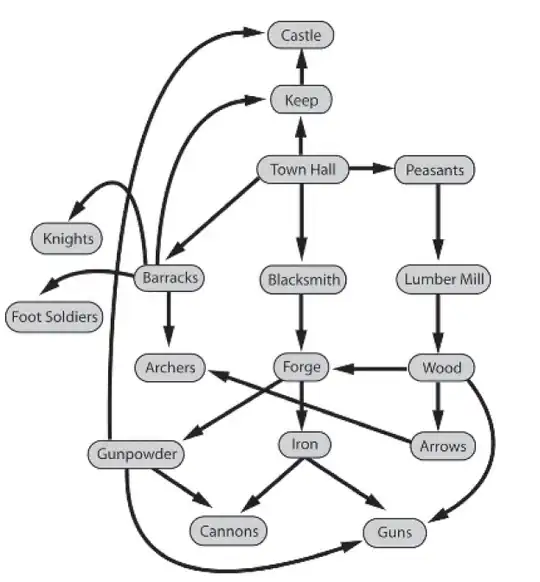
Thresholded:
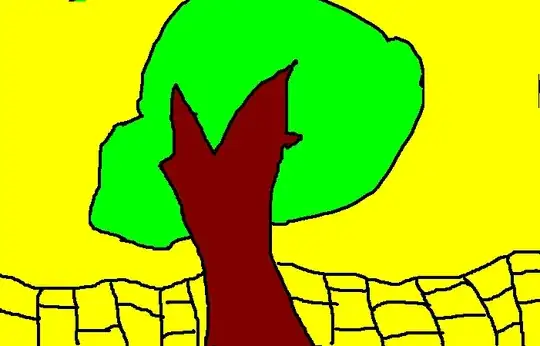
Eroded:
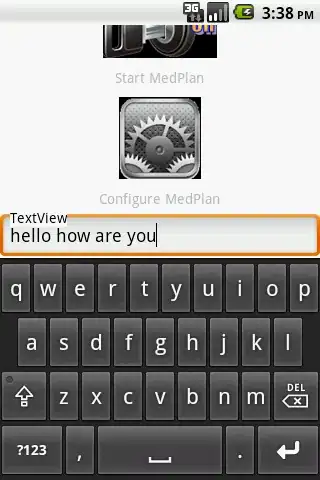
Mask selecting only characters:
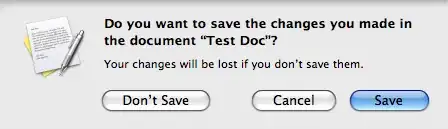
Clean image with bounding boxes:
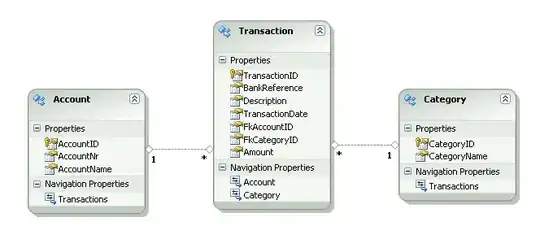
Console output:
Licence plate: 2B99996
C++ code, using OpenCV 2.4.11 and Boost.Filesystem to iterate over files in a directory.
#include <boost/filesystem.hpp>
#include <opencv2/opencv.hpp>
#include <iostream>
#include <string>
// ============================================================================
namespace fs = boost::filesystem;
// ============================================================================
typedef std::vector<std::string> string_list;
struct char_match_t
{
cv::Point2i position;
cv::Mat image;
};
typedef std::vector<char_match_t> char_match_list;
// ----------------------------------------------------------------------------
string_list find_input_files(std::string const& dir)
{
string_list result;
fs::path dir_path(dir);
fs::directory_iterator end_itr;
for (fs::directory_iterator i(dir_path); i != end_itr; ++i) {
if (!fs::is_regular_file(i->status())) continue;
if (i->path().extension() == ".png") {
result.push_back(i->path().string());
}
}
return result;
}
// ----------------------------------------------------------------------------
cv::Mat reduce_image(cv::Mat const& img, int K)
{
int n = img.rows * img.cols;
cv::Mat data = img.reshape(1, n);
data.convertTo(data, CV_32F);
std::vector<int> labels;
cv::Mat1f colors;
cv::kmeans(data, K, labels
, cv::TermCriteria(CV_TERMCRIT_ITER | CV_TERMCRIT_EPS, 10000, 0.0001)
, 5, cv::KMEANS_PP_CENTERS, colors);
for (int i = 0; i < n; ++i) {
data.at<float>(i, 0) = colors(labels[i], 0);
}
cv::Mat reduced = data.reshape(1, img.rows);
reduced.convertTo(reduced, CV_8U);
return reduced;
}
// ----------------------------------------------------------------------------
cv::Mat clean_image(cv::Mat const& img)
{
cv::Mat resized_img;
cv::resize(img, resized_img, cv::Size(), 5.0, 5.0, cv::INTER_CUBIC);
cv::Mat equalized_img;
cv::equalizeHist(resized_img, equalized_img);
cv::Mat reduced_img(reduce_image(equalized_img, 8));
cv::Mat mask;
cv::threshold(reduced_img
, mask
, 64
, 255
, cv::THRESH_BINARY);
cv::Mat kernel(cv::getStructuringElement(cv::MORPH_RECT, cv::Size(3, 3)));
cv::erode(mask, mask, kernel, cv::Point(-1, -1), 1);
return mask;
}
// ----------------------------------------------------------------------------
cv::Point2i center(cv::Rect const& bounding_box)
{
return cv::Point2i(bounding_box.x + bounding_box.width / 2
, bounding_box.y + bounding_box.height / 2);
}
// ----------------------------------------------------------------------------
char_match_list extract_characters(cv::Mat const& img)
{
cv::Mat inverse_img;
cv::bitwise_not(img, inverse_img);
std::vector<std::vector<cv::Point>> contours;
std::vector<cv::Vec4i> hierarchy;
cv::findContours(inverse_img.clone(), contours, hierarchy, CV_RETR_EXTERNAL, CV_CHAIN_APPROX_NONE);
char_match_list result;
double const MIN_CONTOUR_AREA(1000.0);
double const MAX_CONTOUR_AREA(6000.0);
for (uint32_t i(0); i < contours.size(); ++i) {
cv::Rect bounding_box(cv::boundingRect(contours[i]));
int bb_area(bounding_box.area());
if ((bb_area >= MIN_CONTOUR_AREA) && (bb_area <= MAX_CONTOUR_AREA)) {
int PADDING(2);
bounding_box.x -= PADDING;
bounding_box.y -= PADDING;
bounding_box.width += PADDING * 2;
bounding_box.height += PADDING * 2;
char_match_t match;
match.position = center(bounding_box);
match.image = img(bounding_box);
result.push_back(match);
}
}
std::sort(begin(result), end(result)
, [](char_match_t const& a, char_match_t const& b) -> bool
{
return a.position.x < b.position.x;
});
return result;
}
// ----------------------------------------------------------------------------
std::pair<float, cv::Mat> train_character(char c, cv::Mat const& img)
{
cv::Mat small_char;
cv::resize(img, small_char, cv::Size(10, 10), 0, 0, cv::INTER_LINEAR);
cv::Mat small_char_float;
small_char.convertTo(small_char_float, CV_32FC1);
cv::Mat small_char_linear(small_char_float.reshape(1, 1));
return std::pair<float, cv::Mat>(
static_cast<float>(c)
, small_char_linear);
}
// ----------------------------------------------------------------------------
std::string process_image(cv::Mat const& img, cv::KNearest& knn)
{
cv::Mat clean_img(clean_image(img));
char_match_list characters(extract_characters(clean_img));
std::string result;
for (char_match_t const& match : characters) {
cv::Mat small_char;
cv::resize(match.image, small_char, cv::Size(10, 10), 0, 0, cv::INTER_LINEAR);
cv::Mat small_char_float;
small_char.convertTo(small_char_float, CV_32FC1);
cv::Mat small_char_linear(small_char_float.reshape(1, 1));
float p = knn.find_nearest(small_char_linear, 1);
result.push_back(char(p));
}
return result;
}
// ============================================================================
int main()
{
string_list train_files(find_input_files("./chars"));
cv::Mat samples, responses;
for (std::string const& file_name : train_files) {
cv::Mat char_img(cv::imread(file_name, 0));
std::pair<float, cv::Mat> tinfo(train_character(file_name[file_name.size() - 5], char_img));
responses.push_back(tinfo.first);
samples.push_back(tinfo.second);
}
cv::KNearest knn;
knn.train(samples, responses);
string_list input_files(find_input_files("./input"));
for (std::string const& file_name : input_files) {
cv::Mat plate_img(cv::imread(file_name, 0));
std::string plate(process_image(plate_img, knn));
std::cout << file_name << " : " << plate << "\n";
}
}
// ============================================================================
C++ code, using OpenCV 3.1 and Boost.Filesystem to iterate over files in a directory.
#include <boost/filesystem.hpp>
#include <opencv2/opencv.hpp>
#include <iostream>
#include <string>
// ============================================================================
namespace fs = boost::filesystem;
// ============================================================================
typedef std::vector<std::string> string_list;
struct char_match_t
{
cv::Point2i position;
cv::Mat image;
};
typedef std::vector<char_match_t> char_match_list;
// ----------------------------------------------------------------------------
string_list find_input_files(std::string const& dir)
{
string_list result;
fs::path dir_path(dir);
boost::filesystem::directory_iterator end_itr;
for (boost::filesystem::directory_iterator i(dir_path); i != end_itr; ++i) {
if (!boost::filesystem::is_regular_file(i->status())) continue;
if (i->path().extension() == ".png") {
result.push_back(i->path().string());
}
}
return result;
}
// ----------------------------------------------------------------------------
cv::Mat reduce_image(cv::Mat const& img, int K)
{
int n = img.rows * img.cols;
cv::Mat data = img.reshape(1, n);
data.convertTo(data, CV_32F);
std::vector<int> labels;
cv::Mat1f colors;
cv::kmeans(data, K, labels
, cv::TermCriteria(CV_TERMCRIT_ITER | CV_TERMCRIT_EPS, 10000, 0.0001)
, 5, cv::KMEANS_PP_CENTERS, colors);
for (int i = 0; i < n; ++i) {
data.at<float>(i, 0) = colors(labels[i], 0);
}
cv::Mat reduced = data.reshape(1, img.rows);
reduced.convertTo(reduced, CV_8U);
return reduced;
}
// ----------------------------------------------------------------------------
cv::Mat clean_image(cv::Mat const& img)
{
cv::Mat resized_img;
cv::resize(img, resized_img, cv::Size(), 5.0, 5.0, cv::INTER_CUBIC);
cv::Mat equalized_img;
cv::equalizeHist(resized_img, equalized_img);
cv::Mat reduced_img(reduce_image(equalized_img, 8));
cv::Mat mask;
cv::threshold(reduced_img
, mask
, 64
, 255
, cv::THRESH_BINARY);
cv::Mat kernel(cv::getStructuringElement(cv::MORPH_RECT, cv::Size(3, 3)));
cv::erode(mask, mask, kernel, cv::Point(-1, -1), 1);
return mask;
}
// ----------------------------------------------------------------------------
cv::Point2i center(cv::Rect const& bounding_box)
{
return cv::Point2i(bounding_box.x + bounding_box.width / 2
, bounding_box.y + bounding_box.height / 2);
}
// ----------------------------------------------------------------------------
char_match_list extract_characters(cv::Mat const& img)
{
cv::Mat inverse_img;
cv::bitwise_not(img, inverse_img);
std::vector<std::vector<cv::Point>> contours;
std::vector<cv::Vec4i> hierarchy;
cv::findContours(inverse_img.clone(), contours, hierarchy, CV_RETR_EXTERNAL, CV_CHAIN_APPROX_NONE);
char_match_list result;
double const MIN_CONTOUR_AREA(1000.0);
double const MAX_CONTOUR_AREA(6000.0);
for (int i(0); i < contours.size(); ++i) {
cv::Rect bounding_box(cv::boundingRect(contours[i]));
int bb_area(bounding_box.area());
if ((bb_area >= MIN_CONTOUR_AREA) && (bb_area <= MAX_CONTOUR_AREA)) {
int PADDING(2);
bounding_box.x -= PADDING;
bounding_box.y -= PADDING;
bounding_box.width += PADDING * 2;
bounding_box.height += PADDING * 2;
char_match_t match;
match.position = center(bounding_box);
match.image = img(bounding_box);
result.push_back(match);
}
}
std::sort(begin(result), end(result)
, [](char_match_t const& a, char_match_t const& b) -> bool
{
return a.position.x < b.position.x;
});
return result;
}
// ----------------------------------------------------------------------------
std::pair<float, cv::Mat> train_character(char c, cv::Mat const& img)
{
cv::Mat small_char;
cv::resize(img, small_char, cv::Size(10, 10), 0, 0, cv::INTER_LINEAR);
cv::Mat small_char_float;
small_char.convertTo(small_char_float, CV_32FC1);
cv::Mat small_char_linear(small_char_float.reshape(1, 1));
return std::pair<float, cv::Mat>(
static_cast<float>(c)
, small_char_linear);
}
// ----------------------------------------------------------------------------
std::string process_image(cv::Mat const& img, cv::Ptr<cv::ml::KNearest> knn)
{
cv::Mat clean_img(clean_image(img));
char_match_list characters(extract_characters(clean_img));
std::string result;
for (char_match_t const& match : characters) {
cv::Mat small_char;
cv::resize(match.image, small_char, cv::Size(10, 10), 0, 0, cv::INTER_LINEAR);
cv::Mat small_char_float;
small_char.convertTo(small_char_float, CV_32FC1);
cv::Mat small_char_linear(small_char_float.reshape(1, 1));
cv::Mat tmp;
float p = knn->findNearest(small_char_linear, 1, tmp);
result.push_back(char(p));
}
return result;
}
// ============================================================================
int main()
{
string_list train_files(find_input_files("./chars"));
cv::Mat samples, responses;
for (std::string const& file_name : train_files) {
cv::Mat char_img(cv::imread(file_name, 0));
std::pair<float, cv::Mat> tinfo(train_character(file_name[file_name.size() - 5], char_img));
responses.push_back(tinfo.first);
samples.push_back(tinfo.second);
}
cv::Ptr<cv::ml::KNearest> knn(cv::ml::KNearest::create());
cv::Ptr<cv::ml::TrainData> training_data =
cv::ml::TrainData::create(samples
, cv::ml::SampleTypes::ROW_SAMPLE
, responses);
knn->train(training_data);
string_list input_files(find_input_files("./input"));
for (std::string const& file_name : input_files) {
cv::Mat plate_img(cv::imread(file_name, 0));
std::string plate(process_image(plate_img, knn));
std::cout << file_name << " : " << plate << "\n";
}
}
// ============================================================================